Traditional financial modeling, while important, can be a slow, error-prone process. It depends heavily on historical data and manual analysis, which limits its ability to capture the full picture. A survey by KPMG found that 70% of financial models contain errors, leading to inaccurate investment decisions and missed opportunities. And that’s where AI financial modeling helps. AI uses sophisticated machine learning algorithms to analyze vast datasets, which allows real-time data integration, pattern identification, and generation of insightful predictions that surpass the capabilities of conventional methods.
Big names in the industry are already reaping the benefits. Upstart, a FinTech leader, uses AI to approve loans for creditworthy individuals overlooked by traditional models. JPMorgan Chase leverages AI to detect fraudulent transactions in real time, saving millions. BlackRock, the world’s largest asset manager, utilizes AI to build more robust and adaptable investment portfolios for its clients.
In this blog, we’ll explore how Artificial Intelligence boosts efficiency and accuracy and provides insights for smarter investing. We’ll also showcase real-world use cases and compelling case studies from leading companies. Let’s begin.
What Is Financial Modeling?
Financial modeling is the construction of a numerical representation of a company’s financial performance. It is typically built within a spreadsheet or specialized software. This model uses historical financial data to predict future performance based on a set of well-defined assumptions and projections. Financial modeling is an important tool for businesses and investors to make informed decisions. It is used for:
- Analyzing profitability and cash flow: The model assesses a company’s ability to generate revenue, control costs, and ultimately create value for shareholders.
- Evaluating investment opportunities: Financial models can be used to project the potential return on investment (ROI) for various projects or acquisitions.
- Managing risk: By adding different scenarios and assumptions, the model helps identify and quantify potential financial risks.
- Facilitating strategic planning: Financial models provide a framework for analyzing different business strategies and their potential financial implications.
Core Elements and Components of a Financial Model
Here’s a table outlining the Core Elements and Components of a Financial Model:
Core Element | Description | Key Components |
Income Statement | Shows a company’s profitability over a specific period. | Revenue, Cost of Goods Sold (COGS), Operating Expenses, Interest Expense, Taxes, Net Income |
Balance Sheet | Provides a snapshot of a company’s financial position at a specific point in time. | Assets, Liabilities, Shareholders’ Equity |
Cash Flow Statement | Tracks the movement of cash in and out of a business. | Operating Cash Flow, Investing Cash Flow, Financing Cash Flow |
Assumptions | Underpinning factors are used to forecast future financial results. | Growth rates, discount rates, margin assumptions, capital expenditures |
Sensitivity Analysis | Evaluates how changes in assumptions impact financial forecasts. | What-if scenarios testing different assumptions |
Charts and Graphs | Visually represent financial data for easier interpretation. | Line charts, bar charts, pie charts |
Market Trends and Analysis of AI in Financial Services
The global generative AI market in financial services is experiencing explosive growth. A report by Precedence Research predicts a staggering 1,014% increase by 2033, with the market value increasing from $1.19 billion in 2023 to a whopping $13.33 billion. This rapid rise reflects the growing adoption of this powerful technology within the financial sector.
Zooming in on the US market, the trend is equally promising. The US generative AI in the financial services market reached USD 340 million in 2023 and is projected to rise to a remarkable USD 4.03 billion by 2033. This signifies a CAGR of 27.90%, mirroring the global trend and highlighting the significant interest in AI-powered financial modeling within the US.
Hire ex-FANG developers, with combined 50000+ coding hours experience
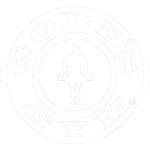
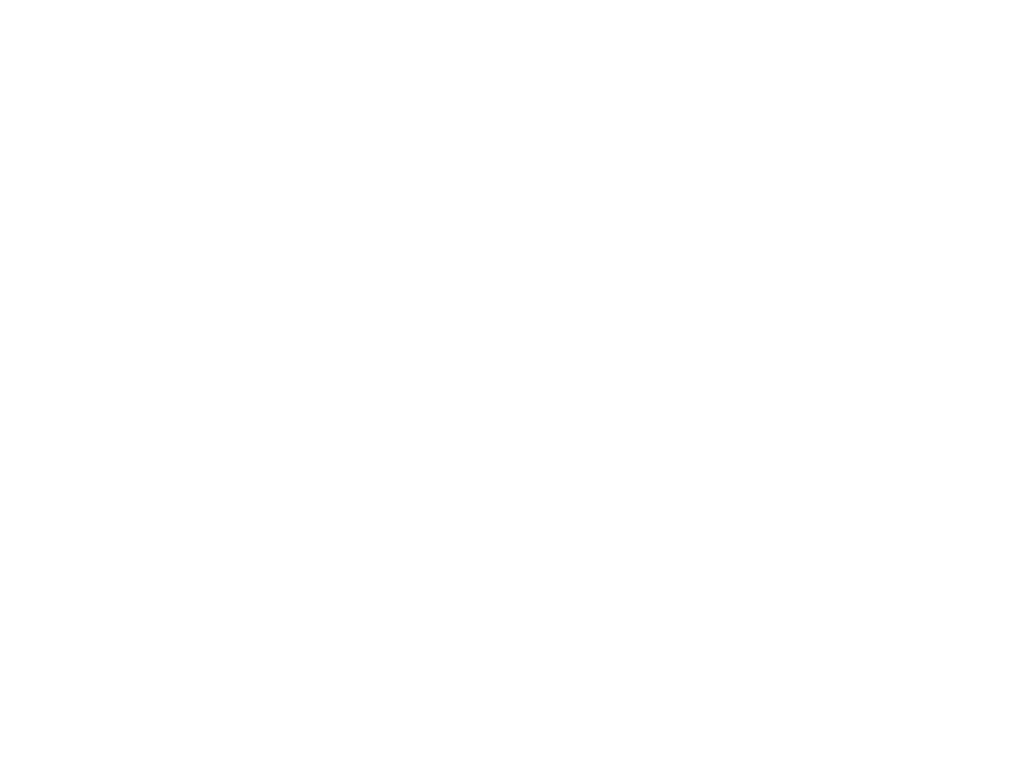
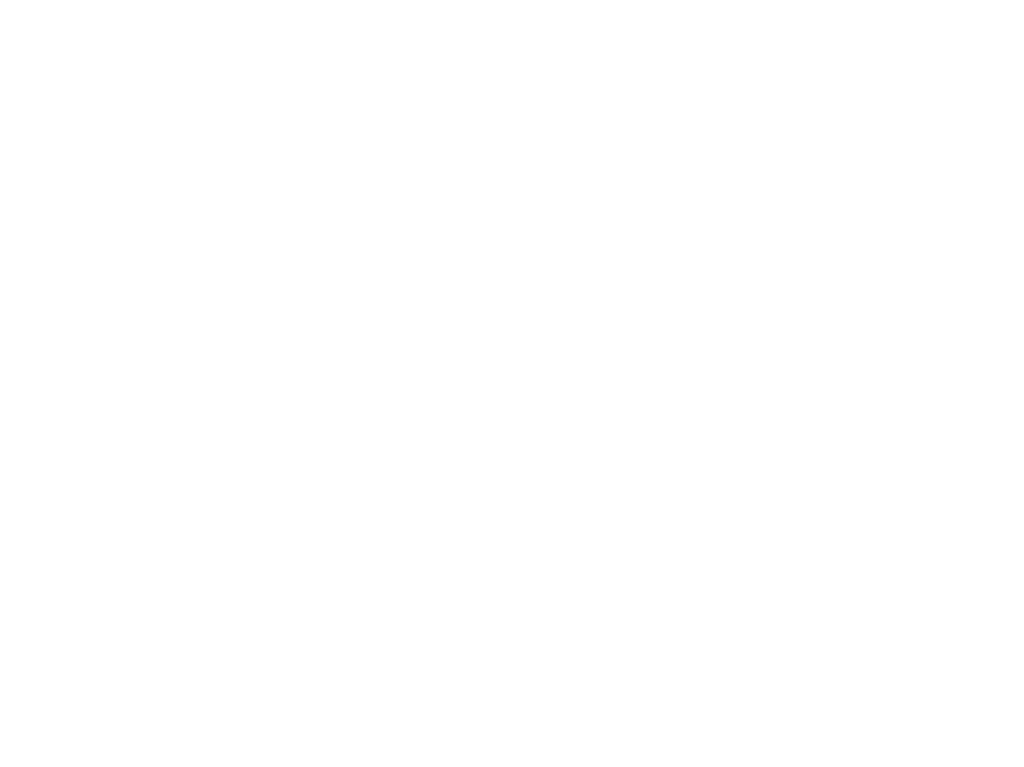
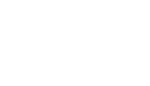
How Does AI Enhance Traditional Financial Modeling?
Let’s look at the limitations of traditional financial modeling and how AI helps:
Automating Data
Financial analysts traditionally spend a lot of time gathering and cleaning data, which leaves less time for deep analysis. AI automates these tasks, allowing analysts to focus on higher-level activities. AI can scan vast datasets from various sources, including financial reports, market trends, and news articles. This comprehensive data pool allows for the identification of hidden patterns and relationships that might be missed by manual analysis.
Real-Time Data Processing
Traditional models rely on historical data, which can become outdated quickly in today’s fast-paced financial landscape. AI can process real-time data feeds, incorporating market fluctuations, news sentiment shifts, and social media buzz into the model. This allows for more responsive and dynamic financial forecasts that adjust with the changing market conditions.
Enhanced Predictivity
Traditional models often use pre-defined formulas and assumptions, limiting their ability to predict future trends. AI uses machine learning algorithms to uncover complex relationships within data. This results in more accurate forecasts and risk assessments. These insights empower analysts to make data-driven decisions and identify potential opportunities based on a more comprehensive understanding of future market behavior.
Anomaly Detection
Traditional models might miss outliers and hidden risks buried in the data. AI analyzes all the data and sniffs out anomalies and potential red flags that could significantly impact financial performance. Early detection of these anomalies allows for proactive risk management, enabling analysts to mitigate potential threats before they materialize.
Improved Stress Testing
Traditional models usually struggle to adapt to changing market conditions. Whereas AI can create stress tests by simulating various “what-if” scenarios based on market shifts, economic changes, or unforeseen events. This allows analysts to test the model’s robustness under different conditions and identify potential weaknesses in a company’s financial performance.
Personalized Insights
Traditional models often lack the ability to personalize financial forecasts for specific investment goals. AI can analyze an investor’s unique profile and risk tolerance, generating customized financial insights tailored to their needs. These personalized insights enable analysts to develop more targeted investment strategies with a higher likelihood of success.
Cost Efficiency
Traditional modeling can be time-consuming and resource-intensive, requiring analysts to spend significant time on repetitive tasks. AI automates these tasks, freeing up valuable time and resources. This allows analysts to focus on higher-level activities such as interpreting results, developing investment strategies, and making informed decisions.
Key Use Cases Of AI In Financial Modeling
Let’s delve into the key use cases of AI in financial modeling in various sectors:
Banking
In the competitive banking sector, AI plays an important role in expanding your customer base while minimizing risk. By analyzing “alternative data” like social media behavior and utility payments, AI helps identify creditworthy borrowers who might be overlooked by traditional methods.
Additionally, AI acts as a real-time fraud detection system, safeguarding your financial institution from fraudulent activities. Furthermore, AI helps optimize loan portfolios by analyzing historical data and market trends, ensuring healthy financials and allowing you to offer competitive rates to attract new customers.
Insurance
The insurance industry can leverage AI to personalize insurance premiums for policyholders, leading to fairer pricing and potentially higher customer satisfaction. AI can also streamline the processing of often cumbersome claims by analyzing medical records and accident reports. This means faster turnaround times and improved customer experience. Similar to the banking sector, AI plays a vital role in identifying fraudulent insurance claims protecting your company from financial losses.
Investment Management
AI helps investment management firms construct dynamic investment portfolios that adapt to market changes in real time. This ensures your clients’ investments are positioned for optimal performance, potentially leading to higher returns. Furthermore, AI-powered algorithms can execute high-frequency trades at incredible speeds, capitalizing on fleeting market opportunities that human traders might miss. This can significantly enhance your investment strategies and potentially generate higher returns for your clients.
Fintech
The rise of Fintech has democratized investing, and AI plays an important role in this transformation. Robo-advisors, powered by AI, offer automated investment management services at a lower cost, making financial planning more accessible to a wider audience. Additionally, AI helps assess borrowers on crowdfunding platforms, allowing you to make informed investment choices and minimize risk in this exciting yet potentially risky space.
Real Estate
In the real estate market, AI provides accurate property valuations by analyzing market data, property features, and location. This streamlines the buying and selling process, preventing under or over-pricing of properties. AI can also predict future property values based on historical data and market trends. This allows you to identify undervalued properties with high potential returns, giving you a competitive edge in the market.
By adopting AI financial modeling, businesses across various industries can automate tasks, identify opportunities, and mitigate risks. This leads to smarter financial decisions, improved customer experiences, and, ultimately, greater success in achieving your business goals.
Case Studies: Successful Implementation of AI in Financial Modeling
Here are some compelling case studies showcasing successful implementations of AI in financial modeling:
Bank of America: Automating Loan Underwriting with AI
Challenge: Traditionally, loan underwriting involved a lengthy manual process prone to human bias. This slowed down loan approvals and limited access to credit for some borrowers.
Solution: Bank of America implemented an AI-powered underwriting system that analyzes various data points beyond credit scores, including social media activity and cash flow. This allows for a more holistic assessment of creditworthiness and faster loan approvals.
Results: The AI system reduced loan processing times by 30% and expanded loan approvals by 15%, particularly for individuals with limited credit history. This lead to increased customer satisfaction and a more inclusive lending approach.
BlackRock: Building Dynamic Investment Portfolios with AI
Challenge: Actively managing investment portfolios requires constant monitoring and adjustments based on market fluctuations. This can be a time-consuming and resource-intensive task.
Solution: BlackRock, a leading investment management firm, utilizes AI to build and manage dynamic investment portfolios. The AI models scan vast amounts of data, including market trends, economic indicators, and company financials, to identify investment opportunities and adjust portfolio allocations accordingly.
Results: BlackRock’s AI-powered portfolios have consistently outperformed the market average, demonstrating the effectiveness of AI in identifying undervalued assets and optimizing investment strategies.
JPMorgan Chase: AI-Powered Cash Flow Model Reduces Manual Work
Challenge: Financial analysts traditionally spend a significant amount of time gathering and cleaning data for financial modeling, leaving less time for deep analysis.
Solution: JPMorgan Chase developed an AI model that can ingest financial documents from various sources and automatically extract relevant data. This streamlines data processing and frees up analysts’ time for higher-level tasks.
Results: The AI model has reduced the time spent on data preparation by 90%. This helps analysts to focus on more strategic tasks, such as identifying trends and developing financial forecasts.
Future Outlook On AI In Finance
As AI financial modeling continues to evolve, we can expect to see its impact extend far beyond the current applications. Here’s a glimpse into the exciting possibilities that lie ahead:
Hyper-Personalization
AI will become even more skillful at understanding individual financial needs and risk profiles. This will result in the creation of hyper-personalized financial products and services, from custom insurance plans to robo-advisors that tailor investment strategies to your unique goals.
Democratization of Finance
AI has the potential to eliminate the barriers to entry in the financial sector. By automating tasks and simplifying complex financial products, AI can help individuals take control of their finances, regardless of their background or financial literacy.
Enhanced Risk Management
AI’s ability to study vast amounts of data in real time will lead to more sophisticated risk management tools. This will not only benefit financial institutions but also allow businesses of all sizes to make better, informed decisions and mitigate potential financial risks.
Algorithmic Trading and Market Prediction
AI-powered algorithms will continue to play a more prominent role in trading, potentially leading to faster and more efficient markets. However, ethical considerations surrounding algorithmic trading and the potential for market manipulation will need to be carefully addressed.
Fraud Detection and Cybersecurity
AI will be at the forefront of the fight against financial fraud. By identifying suspicious patterns and anomalies in real time, AI can help financial institutions prevent fraudulent activities and protect customer data. However, as AI systems become more sophisticated, so too will cyber criminals. The cybersecurity landscape will require constant vigilance and adaptation.
Regulatory Landscape and Transparency
As AI becomes more embedded in financial services, regulatory frameworks will need to adjust and ensure fairness, transparency, and accountability. Explainability and bias detection in AI algorithms will be important to building trust and ensuring the responsible use of AI in finance.
Conclusion
The financial sector is changing with the help of AI, and the benefits are undeniable. From automating tasks and streamlining processes to unlocking hidden opportunities and mitigating risk, AI financial modeling empowers businesses to make smarter choices and achieve long-term success.
The time to adopt AI financial modeling is now. Don’t get left behind by your competitors who are using AI to gain a significant edge. The success stories we’ve explored are just the beginning. AI financial modeling is a rapidly evolving field with the potential to change fundamentally how you manage your finances. By taking a proactive approach and exploring how AI can benefit your business, you can position yourself for a future of financial success and usher your entrepreneurial dreams forward.
Want To Implement AI Solutions In Your Business?
The future is bright for businesses that embrace AI, and financial modeling is just the tip of the iceberg. At Idea Usher, we’re passionate about helping entrepreneurs like you leverage the power of AI to achieve groundbreaking results.
Our Proven AI Implementation Framework takes the guesswork out of integrating AI. We’ll work closely with you to deliver to your specific needs, develop a customized AI strategy, and ensure seamless implementation across your organization.
Don’t wait to get started! Book your free consultation with Idea Usher today.
Hire ex-FANG developers, with combined 50000+ coding hours experience
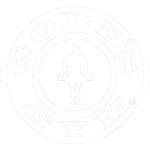
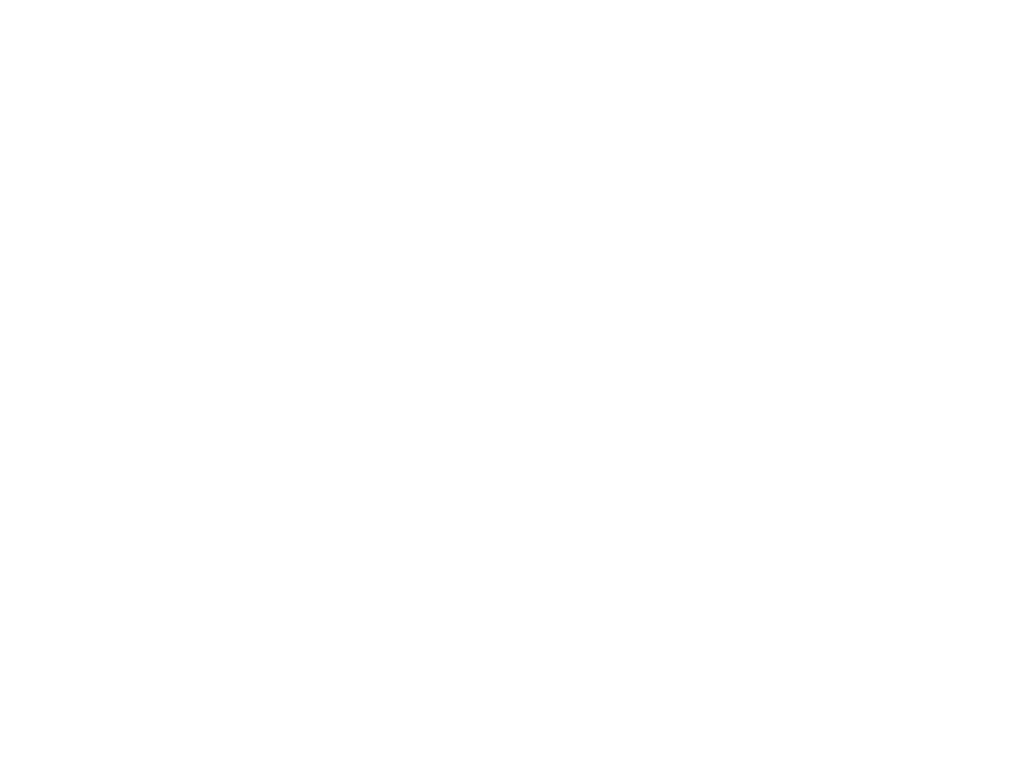
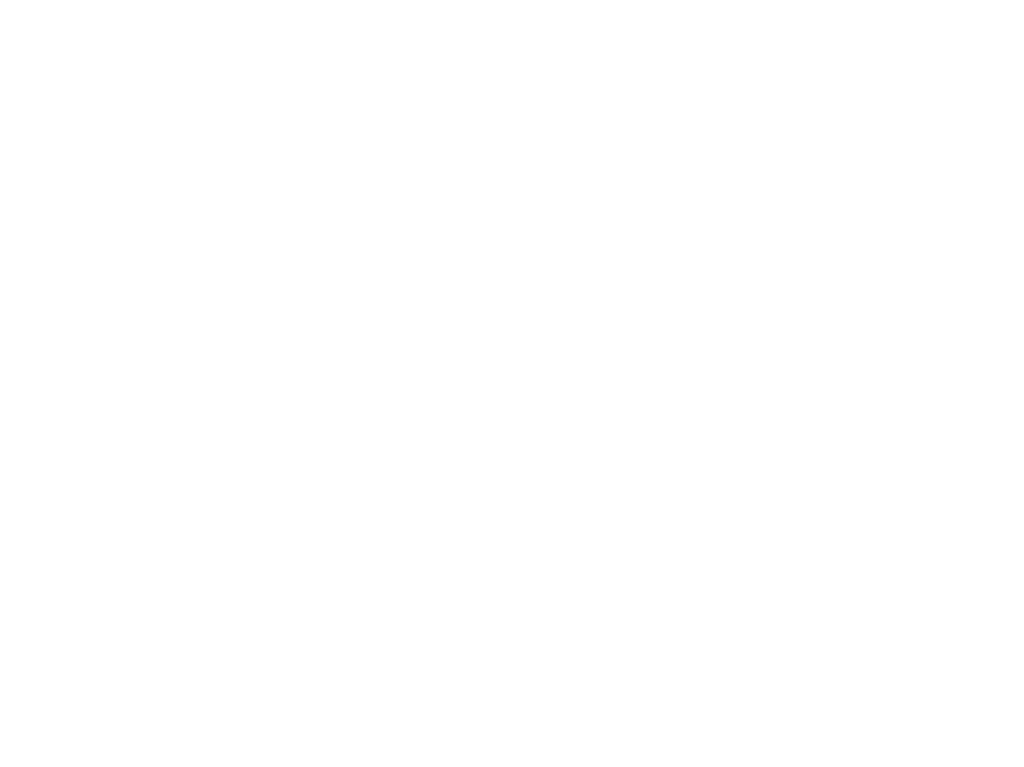
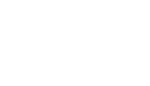
FAQs
How can AI be used in finance?
AI can study vast amounts of data to assess creditworthiness, personalize insurance premiums, and even execute high-speed trades. This automates tasks and frees up professionals for more strategic work.
Can financial modeling be automated?
AI can study market trends and historical data to build dynamic investment portfolios and predict future value. This allows for data-driven financial modeling that adapts to changing conditions.
Why is AI good for finance?
There are several benefits:
- Efficiency: Automating tasks saves time and reduces human error.
- Insights: AI can uncover hidden patterns in data, leading to better risk management and informed decisions.
- Personalization: AI tailors financial products and services to individual needs.
What is generative AI for financial models?
This emerging field uses AI to create entirely new financial models based on specific parameters. It can be used for tasks like stress testing portfolios or simulating different economic scenarios.