Generative AI has emerged as a transformative force within the banking sector, redefining traditional processes and possibilities. This fusion of artificial intelligence and finance empowers institutions to craft personalized experiences, automate intricate tasks, as well as forecast market trends with unprecedented accuracy.
Generative AI’s applications span risk assessment, fraud detection, customer service, and investment analysis, catalyzing efficiency gains as well as innovative breakthroughs. However, alongside its potential, ethical considerations and data security remain paramount.
In this blog, we’ll talk about Generative AI in Banking’s case studies, real-world examples and much more! So, without further ado, let’s get started!
What is Generative AI in Banking?
Generative AI in banking refers to the application of advanced artificial intelligence techniques, particularly generative models, to various tasks within the financial industry. Generative AI models, such as Generative Adversarial Networks (GANs) and Variational Autoencoders (VAEs), have the capability to create new data samples that closely resemble existing data. In the context of banking, these models can be utilized for diverse purposes, including the generation of synthetic financial data for testing and training algorithms, the creation of personalized customer interactions, fraud detection by generating potential fraudulent scenarios, and even the development of realistic economic simulations.
By harnessing the power of generative AI, banks and financial institutions can enhance decision-making, improve customer experiences, and bolster their overall operational efficiency.
How Does Generative AI in Banking Work?
Generative AI holds the potential to revolutionize the way banks operate, make decisions, and interact with customers. But how exactly does Generative AI work in the context of banking?
Generating Synthetic Data
One of the primary applications of Generative AI in banking is the creation of synthetic financial data. Banks deal with enormous amounts of sensitive customer information, and using real data for testing and training purposes can pose security and privacy risks. Generative models like GANs and VAEs can generate synthetic data that closely resembles real data, allowing banks to develop and test algorithms without compromising sensitive information.
Personalized Customer Interactions
Customer experience is paramount in the banking industry. Generative AI can analyze vast amounts of customer data, such as transaction histories and behavior patterns, to create personalized interactions. Chatbots powered by Generative AI can simulate natural language conversations, providing customers with real-time assistance and recommendations tailored to their financial needs.
Fraud Detection and Prevention
Detecting fraudulent activities is a constant challenge in banking. Generative AI can play a crucial role in fraud detection by generating potential scenarios of fraudulent transactions. By training models on historical fraud data, these AI systems can identify unusual patterns and flag suspicious transactions, enhancing banks’ ability to prevent financial fraud.
Economic Simulations
Banks need to navigate complex economic landscapes. Generative AI can assist in developing economic simulations that model different market conditions and potential scenarios. These simulations enable banks to make more informed decisions, assess risks, and devise strategies that are better aligned with the ever-changing economic environment.
Risk Assessment and Portfolio Management
Generative AI can aid in risk assessment by simulating various market conditions and predicting potential outcomes. This can be particularly useful in portfolio management, where banks can use generative models to assess how different investments might perform under different circumstances, thereby optimizing their investment strategies.
Anti-Money Laundering (AML) Compliance
Compliance with regulations such as Anti-Money Laundering (AML) is a critical aspect of banking operations. Generative AI can help banks generate synthetic data for training AML detection models, ensuring these systems are accurate and effective in identifying suspicious activities.
Generative AI in Banking: Key Market Stats
The growth trajectory of Generative AI in the banking sector is undeniably remarkable. With the AI in Banking Market size valued at USD 5.13 Billion in 2021 and projected to surge to USD 64.03 Billion by 2030, at an impressive CAGR of 32.36% from 2023 to 2030, it’s evident that the industry recognizes the transformative potential of AI. Within this landscape, the development and integration of Generative AI hold vast promise.
Source: Verified Market Research
As financial institutions continue to harness data-driven insights for enhanced decision-making, risk assessment, and customer engagement, Generative AI offers a new frontier. Its ability to generate data, simulate scenarios, and predict outcomes can lead to more accurate risk modeling, personalized financial products, and even innovative investment strategies. The scope of developing Generative AI in banking platforms encompasses a spectrum of applications, including crafting tailored customer experiences, augmenting predictive analytics, and advancing fraud detection methods. This dynamic intersection of AI and finance heralds a future where Generative AI reshapes the banking landscape, ushering in unprecedented levels of efficiency and innovation.
Top Generative AI Banking Models
Generative AI models are at the forefront of this transformation, offering innovative solutions to a range of challenges faced by the industry. Let’s delve into some of the top Generative AI banking models that are driving these changes:
Variational Autoencoders (VAEs):
Variational Autoencoders (VAEs) are a class of generative models known for their ability to learn underlying data representations. In banking, VAEs are used to generate synthetic financial data while preserving critical statistical characteristics. This synthetic data is invaluable for testing and training algorithms without compromising customer privacy. VAEs excel at capturing the underlying structure of complex financial data, making them a powerful tool for risk assessment, portfolio management, and even fraud detection.
Generative Adversarial Networks (GANs):
Generative Adversarial Networks (GANs) have swiftly become a cornerstone of modern AI, finding particularly intriguing applications in the finance sector. This unique class of generative AI models operates through a dual mechanism involving a generator and a discriminator. In finance, GANs have garnered attention for their proficiency in synthetic data creation, market simulation, and augmenting risk modeling.
Understanding the GAN Framework
The crux of GANs lies in their intricate interplay between the generator and the discriminator. The generator’s role is to learn the intricacies of real financial data and then fabricate new samples that closely resemble actual data points, such as stock prices, transaction records, or market indicators. Armed with random noise as input, the generator endeavors to craft data that is virtually indistinguishable from genuine financial data. In contrast, the discriminator is honed to differentiate between authentic and generated data. By discerning the telltale traits of real financial data, the discriminator strives to classify synthesized samples as counterfeit.
Adversarial Training: A Quest for Realism
The dynamic training process of GANs is marked by adversarial confrontation. The generator is on a mission to dupe the discriminator by crafting increasingly realistic data, while the discriminator is committed to enhancing its prowess in segregating real from fabricated data. Over time, this adversarial tête-à-tête drives the generator to produce ever-more authentic financial data, while the discriminator hones its ability to differentiate the genuine from the spurious.
Wide-Ranging Applications in Finance
The applications of GANs within the financial realm are both potent and diverse:
Synthetic Data Generation: GANs address data limitations and biases by producing synthetic financial data. This synthetic data proves invaluable for tasks like risk modeling, algorithmic trading, and portfolio optimization. This is where a rich and varied dataset is paramount.
Fraud Detection: GANs contribute to the battle against financial fraud by discerning genuine transactions from fraudulent ones. Their ability to identify patterns within the data bolsters the precision and efficacy of fraud detection in the financial sector.
Market Simulation and Scenario Analysis: GANs can conjure artificial market data. Consequently, aiding in comprehending market dynamics, forecasting price fluctuations, and evaluating the repercussions of diverse variables on financial markets.
Anomaly Detection: GANs excel at detecting anomalies or outliers within financial data. Consequently, facilitating the early identification of irregular patterns that might signify emerging risks.
Autoregressive Models:
Autoregressive models are a traditional yet effective approach in the realm of Generative AI. These models generate sequences of data points where each point is predicted based on preceding data points. In banking, autoregressive models can be employed to forecast market trends, stock prices, and economic indicators. Their ability to capture sequential dependencies makes them valuable for time series analysis. Thus, aiding financial experts in making informed investment decisions.
Transformer Models:
Transformer models have revolutionized the field of natural language processing and have found applications in banking as well. These models, known for their attention mechanisms, are adept at capturing intricate patterns and relationships in sequential data. In the banking sector, transformer models are used to generate human-like text for customer interactions, fraud detection alerts, and market trend analyses. Their versatility and contextual understanding make them a valuable asset in enhancing customer experiences and decision-making processes.
Challenges and Considerations of Generative AI in Finance Industry
The rapid strides of generative AI have ignited a discourse on its ethical dimensions, particularly in sectors like finance or with AI in fintech sector, where its potential impact is profound. The ethical exploration goes beyond what generative AI can accomplish; it delves into what it should do and when, striking a delicate balance between innovation and responsibility.
Addressing Bias and Fairness
Generative AI models can inadvertently perpetuate biases present in their training data, leading to discriminatory outputs. In finance, this could lead to skewed decisions affecting individuals’ financial well-being. Institutions must prioritize fairness, transparency, and equity in the deployment of these models, actively identifying and mitigating biases to ensure unbiased outcomes.
Safeguarding Data Privacy and Security
Financial institutions handle sensitive data, demanding stringent data privacy measures. Training AI models with this data raises concerns about inadvertent exposure or misuse. Robust data anonymization, encryption, and strict access controls are imperative to protect customer information and maintain data integrity.
Ensuring Model Accuracy
Generative AI models’ outputs hold substantial consequences, especially in financial contexts. Rigorous verification is essential to prevent AI-generated inaccuracies that could have far-reaching impacts on decisions related to taxes, investments, or financial planning. Human oversight remains crucial to maintaining accuracy and accountability.
Bridging the Expertise Gap
Developing generative AI models requires a fusion of finance and AI expertise. The scarcity of professionals well-versed in both domains presents a challenge for financial institutions. Fostering collaboration between data scientists, AI specialists, and finance professionals is pivotal to harnessing generative AI effectively.
Balancing Scalability and Integration
Integrating generative AI solutions across a financial institution is complex. The seamless scalability necessary for widespread adoption must not disrupt ongoing operations or compromise compliance efforts. Diligent planning ensures a harmonious integration that aligns with operational continuity and regulatory standards.
Complying with Regulations
Stringent financial regulations and standards like AML, GDPR, and KYC dictate data handling and customer protection. Generative AI must navigate these regulatory frameworks, ensuring compliance to avoid legal and compliance risks. The evolving landscape, including new regulations like the Consumer Duty, heightens the responsibility of financial institutions to act in customers’ best interests.
Use Cases for Generative AI in Banking
Here are some prominent Generative AI use cases —
Fraud Detection and Prevention
Fraud detection and prevention are paramount concerns within the banking and financial services industry. The ever-evolving landscape of fraudulent activities presents substantial challenges for institutions striving to fortify their systems and shield their clientele. Conventional rule-based systems and static fraud detection models often struggle to keep pace with the ingenious techniques employed by fraudsters. Additionally, the sheer volume of financial transactions and data generated makes manual identification of fraudulent patterns both onerous and time-sensitive. This underscores the need to explore cutting-edge technologies such as generative AI to bolster the effectiveness of fraud detection and prevention strategies.
Utilizing Generative AI for Synthetic Data Generation to Simulate Fraudulent Patterns
Generative AI emerges as a potent tool for crafting synthetic data that impeccably mirrors fraudulent patterns. By training generative AI models on expansive datasets encompassing documented instances of fraudulent transactions, it becomes possible to generate synthetic data that precisely imitates the attributes and behaviors of fraudulent activities. This synthesized data serves as a testing ground for financial institutions to fine-tune and evaluate their fraud detection systems against lifelike scenarios. Such exposure equips these systems to discern an array of potential fraudulent patterns, thus enhancing their ability to preclude and counteract fraudulent endeavors effectively.
Elevating Fraud Detection Algorithms and Systems via Generative AI
Generative AI holds the potential to substantially elevate the capabilities of fraud detection algorithms and systems. By harnessing synthetic data spawned by generative AI, financial institutions can elevate the precision and efficacy of their fraud detection models. These models can extrapolate from the synthetic data to pinpoint subtle deviations and anomalies that may signify fraudulent conduct. The marriage of generative AI with fraud detection begets sturdier algorithms capable of evolution, adaption, and resilience against emerging fraud strategies, empowering institutions to pre-empt and outwit sophisticated fraudsters.
Training Machine Learning Models with Synthetic Transaction Data
Generative AI-crafted transactional data becomes fodder for training machine learning models tailored explicitly for fraud prediction. By assimilating synthetic data into the training process, these models broaden their knowledge base of fraudulent patterns, thus refining predictive proficiencies. Models infused with generative AI-derived data can discern fraudulent undertakings with enhanced precision, ameliorating instances of both false positives and negatives. This translates into a more efficient and discerning fraud detection system, curbing financial losses due to fraudulent transactions and assuaging disruptions in bona fide customer dealings.
Securing Financial Transactions Through Generative AI
Generative AI extends a spectrum of benefits to fortify the security of financial transactions. Harnessing the potency of generative AI, financial institutions can proactively unmask and thwart fraudulent activities, bestowing a protective cloak upon customer accounts and assets. The capacity to spawn synthetic data mirroring fraudulent patterns empowers institutions to continually stress-test and hone their fraud detection systems, imbuing them with robustness and efficacy. This, in turn, fosters customer trust in the institution’s commitment to security measures
Personalized Customer Experience
The significance of providing such personalized experiences goes beyond meeting expectations; it’s about nurturing stronger customer relationships and standing out in a highly competitive market. By offering personalized services, financial institutions not only foster trust and loyalty but also create a deeper connection with customers, driving their long-term engagement.
Use of Generative AI to Offer Personalized Financial Advice
One of the remarkable applications of Generative AI in banking is the ability to provide personalized financial advice. By harnessing individual customer data and preferences, Generative AI algorithms analyze intricate details like spending habits, transaction histories, and future financial aspirations. With this information at hand, the algorithms generate tailored recommendations that align with each customer’s specific circumstances. This empowers customers to make well-informed decisions about their finances, thus, enabling smarter choices related to budgeting, saving, investing, and more. Ultimately, this personalized guidance contributes in order to enhancing customers’ financial well-being and confidence.
Generation of Customized Investment Portfolios using Generative AI Algorithms:
Generative AI algorithms hold immense potential for crafting customized investment portfolios that cater in order to customers’ unique preferences and financial goals. By considering factors like risk tolerance, investment objectives, as well as time horizons, these algorithms optimize asset allocation and propose suitable investment options. The algorithms analyze historical market data as well as utilize advanced techniques in order to suggest investment strategies that are finely aligned with customers’ financial aspirations. This personalized approach not only enhances the effectiveness of investment decisions but also ensures a more tailored and satisfying experience for customers.
Benefits of Personalized Product Recommendations and Offers through Generative AI:
Generative AI brings a significant advantage to the table by enabling personalized product recommendations and offers. By delving into customer behavior, preferences, and past transaction patterns, these algorithms can generate finely tuned product suggestions, encompassing items such as credit cards, loans, insurance policies, or investment products. These precise recommendations help customers discover products that resonate with their specific needs, thereby enhancing satisfaction and boosting conversion rates. For financial institutions, personalized product recommendations open avenues for cross-selling and upselling. Consequently, leading to increased revenue and amplified customer lifetime value.
Impact of Generative AI on Customer Engagement and Satisfaction Levels:
The introduction of Generative AI in the realm of personalized experiences dramatically impacts customer engagement and satisfaction. Through tailor-made solutions, financial institutions can strengthen their bonds with customers. The ability to offer bespoke financial advice, investment strategies, and product suggestions showcases a profound understanding of customers’ individual requirements. This, in turn, bolsters customer engagement and satisfaction, fostering a sense of appreciation and support from their financial service providers.
Risk Assessment and Credit Scoring
Generative AI is a game-changer in risk assessment and credit scoring. By analyzing a diverse range of data sources, including traditional credit histories and unconventional indicators like online behavior, social media activity, and more, these AI models provide a more comprehensive view of an individual’s financial behavior. This leads to more accurate risk assessments. Thus, enabling banks to make informed lending decisions, especially for those with limited credit histories.
Chatbots and Virtual Assistants
Chatbots and virtual assistants have emerged as game-changers in the banking and financial services sector, elevating customer support and engagement to new heights. These AI-driven conversational agents, designed to interact naturally with customers, bring automated assistance and query resolution to the forefront. With their ability to be available around the clock, these virtual assistants and chatbots have become indispensable tools for financial institutions, driving personalized experiences, operational efficiency, and customer satisfaction to new levels.
Enhancing Conversational Abilities through Generative AI
At the heart of their success lies Generative AI, which significantly elevates the conversational capabilities of virtual agents. By tapping into generative AI models, virtual assistants can generate responses that mirror human-like interactions while remaining contextually relevant. These models comprehend the underlying intent of customer queries, enabling them to furnish accurate and meaningful answers. Generative AI empowers virtual agents to engage in fluid, dynamic conversations, fostering a seamlessly integrated customer experience.
Crafting Context-Aware and Realistic Responses
Generative AI empowers virtual agents to craft responses that are deeply embedded in context and authenticity. By analyzing extensive datasets encompassing customer interactions, historical data, and relevant knowledge bases, generative AI algorithms curate responses tailored to the specific query and customer context. This level of personalization and contextual comprehension equips virtual agents to furnish precise and pertinent information, fundamentally enhancing the overall customer journey.
Benefits of Generative AI-Powered Chatbots
The advantages of employing Generative AI-powered chatbots in customer support as well as engagement are manifold. Firstly, these chatbots ensure instantaneous and uninterrupted assistance, curbing customer wait times and amplifying response efficiency. Swift query resolution not only boosts customer satisfaction but also fosters trust in the institution’s commitment to service. Secondly, Generative AI-driven chatbots deliver personalized responses, consequently, forging more immersive and customer-centric interactions. By deciphering individual customer preferences and historical patterns, chatbots proffer recommendations, suggestions, as well as remedies aligned with specific needs, thus enriching the customer experience.
Trading and Investment Strategies
For investment banks and asset management firms, Generative AI provides a competitive edge. These AI models analyze historical market data, economic indicators, as well as global events in order to generate insights that guide trading strategies as well as investment decisions. The ability to uncover hidden patterns as well as predict market trends can lead to more successful trading outcomes.
Compliance and Regulatory Reporting
The banking industry is subject to rigorous regulatory requirements. Generative AI streamlines compliance efforts by automating the generation of reports needed for audits and regulatory submissions. These models can sift through complex data sets in order to compile accurate as well as comprehensive reports. Thus, ensuring adherence to regulatory standards.
Cybersecurity and Risk Management
As cybersecurity threats evolve, banks must stay one step ahead. Generative AI enhances cybersecurity by simulating potential attack scenarios as well as identifying vulnerabilities in the system. Additionally, these AI models contribute to risk management by assisting in the identification and mitigation of emerging threats.
Loan Underwriting and Mortgage Approval
Generative AI transforms the loan underwriting process by assessing an applicant’s creditworthiness through a holistic lens. By analyzing a variety of data points beyond credit history, including income patterns as well as spending behaviors. These models provide a more nuanced perspective. This leads to fairer loan approvals as well as reduces the risk of defaults.
Top 5 Generative AI Banking Platforms in the Market Right Now
Here are the top 5 Generative AI banking platforms currently making waves in the market:
Morgan Stanley’s Next Best Action
Morgan Stanley’s Next Best Action (NBA) engine stands as a prime example of Generative AI’s transformative impact. This AI-based engine empowers financial advisors with personalized investment recommendations, operational alerts, as well as valuable insights tailored to each client’s unique needs and circumstances. By leveraging advanced algorithms, NBA enables advisors in order to deliver timely and relevant advice, thus, enhancing the client-advisor relationship as well as fostering more informed investment decisions.
JPMorgan Chase & Co.’s ChatGPT-like Software
JPMorgan Chase & Co. has embraced the power of Generative AI with its own ChatGPT-like software. This platform employs natural language processing and generation capabilities in order to facilitate seamless interactions between customers and the bank. Chatbots powered by this technology offer real-time assistance, addressing customer queries, providing transaction details, and even guiding users through the application process—all while delivering a user experience that feels personalized and human-like.
Bloomberg’s BloombergGPT Language Model
Bloomberg, a leader in financial data and news, has harnessed Generative AI in order to develop the BloombergGPT language model. This AI-driven model can analyze vast amounts of financial data, news articles, as well as market trends in order to generate insightful reports, summaries, and even predictive analysis. By providing users with timely and accurate information, BloombergGPT aids financial professionals in making informed decisions as well as staying ahead of market trends.
Brex’s AI-Enabled Insights for CFOs and Finance Teams
Brex, a fintech company, has integrated Generative AI into its platform to provide AI-enabled insights to CFOs and finance teams. This platform analyzes financial data to generate actionable insights that help businesses optimize their spending, manage expenses, as well as make strategic financial decisions. By leveraging AI-generated insights, companies can consequently enhance their financial planning as well as drive greater fiscal efficiency.
ATP Bot’s AI-Quantitative Trading Bot Platform
Quantitative trading has found a new ally in ATP Bot’s AI-Quantitative trading bot platform. This Generative AI-driven solution employs sophisticated algorithms in order to analyze market trends, historical data, as well as macroeconomic indicators. It then generates trading strategies that can be executed automatically, consequently, allowing traders as well as investors in order to capitalize on market opportunities in real-time and make data-driven investment decisions.
Conclusion
As financial institutions harness their potential, they must tread thoughtfully, balancing the advantages of personalization and efficiency with the ethical dimensions of data usage as well as security. Navigating this landscape requires a harmonious synergy between technological advancement and responsible implementation.
Generative AI’s impact on banking is not just a trend but a pivotal juncture, urging the industry to adapt, evolve, and ultimately shape a future where finance and artificial intelligence coalesce for the betterment of all stakeholders.
Unlock the Potential of Generative AI Today! Connect with Ideausher, where our team of experts is poised to unleash the remarkable capabilities of Generative AI. Thus, transforming your business processes like never before.
Hire ex-FANG developers, with combined 50000+ coding hours experience
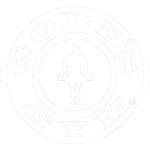
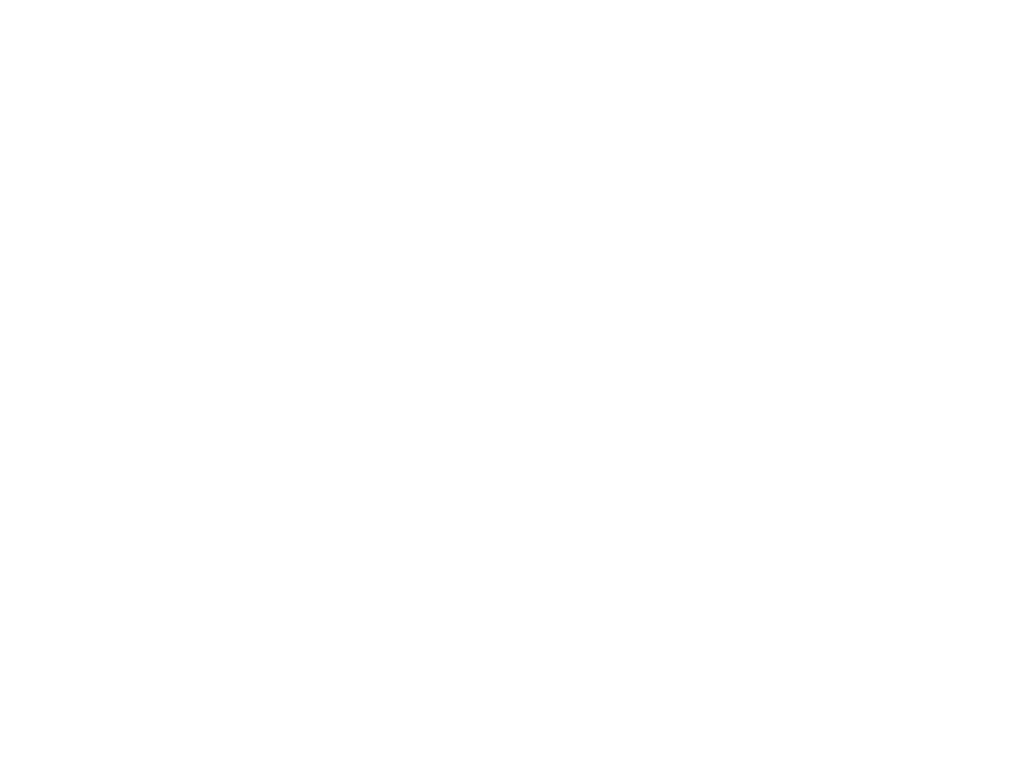
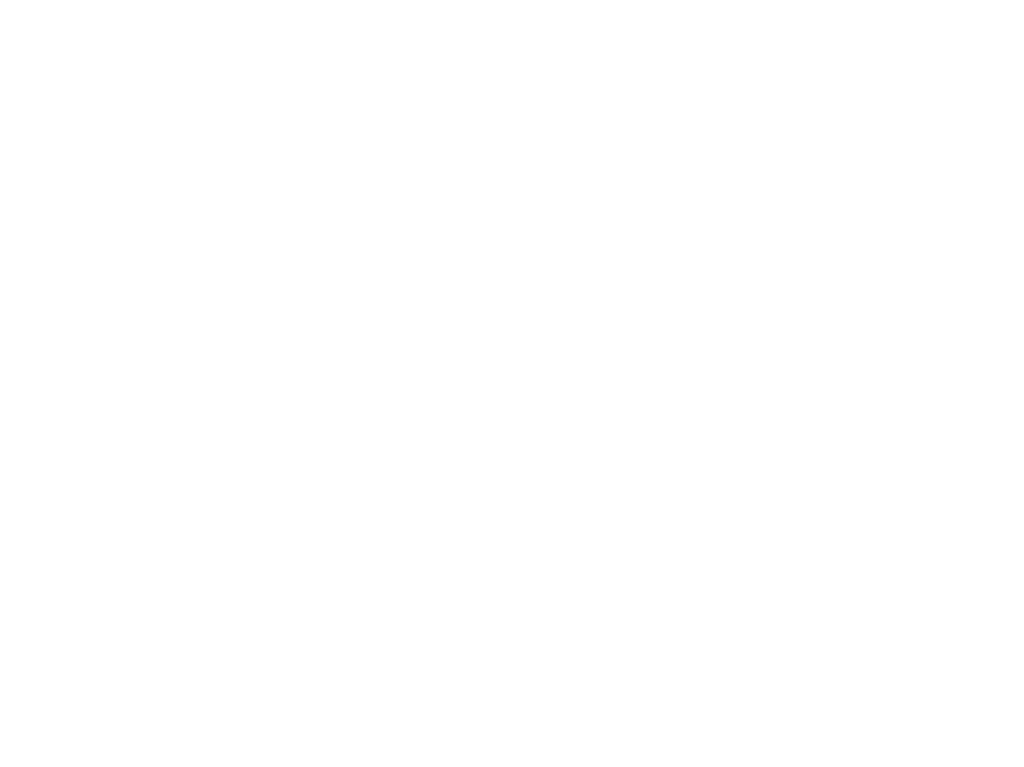
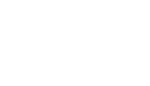
Frequently Asked Questions
Q. How do Recurrent Neural Networks (RNNs) enhance customer service in the banking sector?
A. Recurrent Neural Networks (RNNs) are vital for improving customer interactions in banking. With their ability to process sequential data, RNNs can power chatbots as well as virtual assistants that understand and respond contextually to customer queries. This technology enables personalized customer service, efficient query resolution, as well as even financial advisory services.
Q. What challenges arise when implementing Generative AI for fraud detection in banking?
A. Implementing Generative AI for fraud detection brings challenges like class imbalance as well as the need for continuous learning. Training a model to distinguish between genuine as well as fraudulent activities requires substantial high-quality data. Additionally, staying ahead of evolving fraud tactics demands models that can adapt and learn from new patterns in real-time.
Q. How can Reinforcement Learning be employed for algorithmic trading in banking?
A. Reinforcement Learning (RL) offers opportunities for algorithmic trading in banking by optimizing trading strategies. RL models learn by interacting with a trading environment, adjusting strategies based on rewards as well as penalties. This approach enables the creation of adaptive trading algorithms that can navigate complex market dynamics more effectively.
Q. What privacy measures should be in place when using Generative AI for customer data analysis?
A. Privacy is paramount when using Generative AI for customer data analysis. Techniques like Differential Privacy can be applied to ensure that individual data remains anonymized while still contributing to the training of AI models. Federated Learning is another approach where models are trained locally on individual devices, consequently minimizing the need to centralize sensitive data.