Personalization is the key to capturing and retaining customer attention. Businesses face the challenge of adapting to a competitive marketplace with ever-shortening attention spans. Much like the art of selecting a memorable gift, successful customer engagement stems from understanding an individual’s tastes and preferences. Consumers no longer want to be lumped into broad segments; they crave unique, individualized interactions and recommendations.
However, the vast sea of data and myriad marketing channels make delivering tailored experiences a daunting task. This is where Artificial Intelligence (AI) steps in as a transformative force. AI offers the potential to revolutionize customer experiences by enabling personalization at scale, ushering in a new era of customer engagement across various industries.
AI’s importance in personalization
AI is not just a technological innovation but a powerful enabler of meaningful customer engagement. It is the tool that allows businesses to understand, connect, and respond to each customer’s unique preferences, ultimately crafting a personalized experience that stands out in the competitive marketplace.
But what makes AI so essential for personalization, and why can’t traditional statistical models suffice in this data-rich landscape?
AI’s Power in Personalization
AI’s ability to consolidate diverse data sources into a unified customer profile is a game-changer. By employing advanced analytics, AI can discern the optimal response to individual behaviors, commonly known as the Next Best Action (NBA). This approach is not confined to a specific industry; it is applicable from financial services to life sciences, as companies are reimagining their customer engagement strategies to match the evolving consumer mindset.
Why AI Shines Over Traditional Models
Traditional statistical models, while valuable in their own right, struggle to cope with the sheer volume and complexity of today’s data. AI, on the other hand, excels in this arena, providing scalability and efficiency. It adapts to ever-changing data patterns and customer preferences, something traditional models often fall short of achieving.
AI’s Role in Crafting Unique Customer Experiences
In our exploration of Next Best Action AI, we will delve into its inner workings, unravel its mechanisms, and uncover the key technologies supporting it. From machine learning algorithms to natural language processing, we will dissect the tools that make AI a linchpin of personalization.
Practical Applications Across Industries
This journey through personalization and AI’s pivotal role will also reveal real-world applications across diverse industries. From guiding financial institutions to recommend the best investment strategies for individual clients to aid life sciences companies in tailoring patient treatments, AI holds the potential to redefine customer engagement strategies across the board.
The Road Ahead
As we navigate the intricate world of personalization and the indispensable role of AI, we aim to provide a comprehensive understanding of the opportunities and challenges that lie ahead. The quest for a truly individualized customer experience is ongoing, but with AI leading the way, businesses are better equipped than ever to meet these demands.
What is the Next Best Action AI?
Businesses are now seeking innovative ways to connect with their audiences on a deeper level. One such breakthrough approach, Next Best Action (NBA), powered by cutting-edge machine-learning algorithms, is reshaping the dynamics of customer interaction and personalization.
NBA, a data-driven strategy, stands out from traditional personalization methods that rely solely on demographics or broad audience segments. It harnesses the power of real-time data to decipher intricate patterns in customer behavior across various touchpoints. This agility enables organizations to predict the most effective actions that lead to successful outcomes, such as conversions.
The journey begins with the collection of granular customer behavioral data as they traverse a myriad of channels. This data serves as the lifeblood of the NBA machine-learning algorithm, constantly recalibrating the likelihood of conversion for different action options. This dynamic approach ensures that organizations remain in sync with ever-evolving customer behaviors.
The NBA decision focuses on selecting the right channel, delivering the perfect message, and timing it impeccably. The ultimate goal is to customize communications and offers for targeted customer profiles, with the primary objective being maximizing desired outcomes, often centered around making a purchase.
Building a triumphant NBA model involves three key steps:
Comprehensive Customer Insight
It begins with developing a comprehensive view of customer interactions across multiple channels. This deep understanding is the cornerstone of effective NBA implementation.
Segmentation Mastery
Identifying distinct customer segments is the next vital step. This allows organizations to fine-tune their approaches for maximum resonance.
Tailored Tactics
Understanding what tactics resonate best with each customer group is key. By aligning actions with customer preferences, businesses can forge a more personalized and relevant experience.
Importance of Next Best Action AI
In the marketing industry, the key to success lies in creating personalized, timely, and contextually relevant experiences for customers. This is where Next Best Action AI steps in, revolutionizing the way marketers engage with their audience. Unlike traditional marketing campaigns, which follow pre-planned strategies, Next Best Action (NBA) AI operates in real-time, responding dynamically to specific customer interactions. This real-time adaptability is what sets the NBA apart and allows businesses to craft the optimal customer experience.
Picture a retailer striving to offer a seamless shopping experience, whether in-store or online. As customers interact with the brand, it’s imperative to respond in ways that align with their unique preferences and needs. However, personalizing these experiences at scale for thousands or even millions of customers can be a monumental challenge. NBA AI bridges this gap by harnessing the power of machine learning to understand context, analyze behavior, and make decisions in near-real time.
Next Best Action AI surpasses the capabilities of rule-based algorithms, the foundation of A/B and multivariate testing. While rule-based systems inform basic decisions, they struggle when it comes to identifying behavioral cues at scale, especially in scenarios with multiple available actions. Machine learning, a subset of AI, excels in this area by learning from vast datasets and detecting intricate patterns in customer behavior, leading to more precise and contextually relevant actions.
Let’s delve into a few standout examples:
Adobe Sensei’s One-Click Personalization
Adobe Sensei’s Auto Target, or “One-Click Personalization,” utilizes machine learning models to discern which content layouts resonate most with consumers. This empowers marketers to customize customer experiences based on what’s most likely to engage individual customers. Algorithmically guided tools help sellers find the optimal approach for contacting prospects and recommend messaging strategies with the highest likelihood of success.
Salesforce Einstein’s Next Best Action
Salesforce Einstein leverages Next Best Action technology to empower sellers with informed decisions about product recommendations, timing, and channels. This is facilitated through the embedded Einstein Prediction Builder on customer contact pages. Additionally, NBA technology aids contact center agents by offering AI-guided interactions, preventing the frustration caused by redundant information requests.
Netflix’s Content Recommendations
Netflix, the streaming giant, is a prime example of Next Best Action AI in action. It employs machine learning algorithms to analyze your viewing history, preferences, and even the behavior of users with similar tastes. This enables Netflix to provide you with highly personalized content recommendations. The platform’s success is, in large part, due to its ability to keep viewers engaged by continually suggesting shows and movies that align with their interests, resulting in increased viewer satisfaction and longer subscription retention.
Spotify’s Music Discovery
Spotify, the popular music streaming service, uses Next Best Action AI to enhance music discovery. By analyzing your listening habits and comparing them with those of users with similar tastes, Spotify generates customized playlists like “Discover Weekly” and “Release Radar.” These playlists introduce users to new artists and songs they are likely to enjoy. This approach keeps users engaged and provides an exceptional listening experience, ultimately improving customer loyalty and app usage.
These examples demonstrate how Next Best Action AI is making waves not only in marketing but also in the entertainment and music streaming industries, showcasing its potential to create remarkable customer experiences and drive user engagement.
In these cases, Next Best Action AI plays a pivotal role in enhancing customer experiences, optimizing marketing efforts, and guiding sales and service interactions based on data-driven insights. Whether it’s through personalization, timely recommendations, or intelligent assistance for contact center agents, AI and machine learning technologies are reshaping the way businesses connect with their customers.
AI Next Best Action models
Reinforcement Learning (RL) stands out as a powerful paradigm for determining the Next Best Action in a multitude of scenarios. RL is rooted in the idea of learning through interactions with the environment, where an agent makes decisions, receives feedback in the form of rewards or penalties, and endeavors to learn a policy that maximizes cumulative rewards over time.
At the core of RL lies the concept of a Markov Decision Process (MDP), a mathematical framework ideally suited for modeling decision-making problems characterized by a blend of randomness and decision-maker control. This framework comprises five vital components that shape the decision-making process:
States
States encapsulate the array of situations or contexts an RL agent may encounter. They are the backdrop against which actions unfold.
Actions
Actions represent the choices the agent can make in each state, paving the way for subsequent interactions.
Rewards
Rewards serve as immediate feedback, providing the agent with information on the consequences of its actions in a given state.
Transition Probabilities
Transition probabilities outline the likelihood of moving from one state to another upon taking a specific action. They embody the dynamic nature of the environment.
Discount Factors
Discount factors introduce a temporal dimension by determining the importance of future rewards in contrast to immediate ones. They weigh the long-term consequences of actions.
In the RL ecosystem, the agent takes action, observes state changes, reaps rewards, and continually refines its policy—a mapping from states to actions. The objective is to unearth the optimal policy that maximizes cumulative rewards over time.
RL navigates this journey through various techniques, including:
Value-Based Methods: Such as Q-learning, which learns the values of state-action pairs and makes decisions based on the estimated value.
Policy-Based Methods: Like Policy Gradient, which learns a direct policy, specifying the probability of taking each action in every state.
Model-Based Methods: These methods aim to construct a model of the environment to inform decision-making.
However, the game-changing innovation lies in Deep Reinforcement Learning (DRL), which marries RL with deep neural networks to decipher intricate policies from high-dimensional input data. DRL has cast its transformative spell across diverse domains, including robotics, recommendation systems, financial optimization, and even the realm of gaming with milestones like AlphaGo.
Now, consider the application of RL in the context of Next Best Action. RL becomes a beacon for determining the most effective actions for each customer in real time, taking into account a customer’s historical interactions, current context, and potential future engagements. The potential implications are profound: enhanced customer satisfaction, elevated revenue streams, and optimized resource allocation. It’s the beacon guiding businesses toward data-driven, personalized, and contextually rich customer interactions.
As AI continues to advance, the synergy of Reinforcement Learning and Next Best Action is poised to be a game-changer, delivering enhanced decision-making capabilities in a wide array of scenarios.
How does Next Best Action AI work?
Next Best Action AI (NBA) is like the key player that makes personalized suggestions happen. To demystify the workings of the NBA, we delve into its intricate elements and illuminate the manifold advantages it bestows upon businesses.
Next Best Action AI is the vanguard of customer engagement, wielding data-driven insights and cutting-edge AI technologies. This approach equips businesses to provide interactions that are personalized, timely, and contextual, ultimately fostering profitability and customer satisfaction.
Single Customer View (SCV)
NBA embarks on its journey by sculpting a Single Customer View. This all-encompassing profile amalgamates a customer’s multifaceted interactions, transactions, and inclinations across various touchpoints. It embraces a spectrum spanning demographics, purchase history, online habits, and even social media activities. By knitting together data from diverse sources—CRM systems, marketing databases, transaction archives—organizations construct a panoramic customer profile. This coherent perspective empowers them to make judicious decisions and customize their approach.
Real-time Feedback Loop
At the core of NBA lies the real-time feedback loop—a continuous, data-rich conduit. It captures and assimilates fresh customer interactions in the present moment, molding insights based on these updates. Every customer touchpoint, whether it’s a website visit, email opening, or link clicking, is meticulously recorded and timestamped. This wealth of real-time data nourishes the machine-learning models underpinning NBA systems. These models evolve and fine-tune themselves incessantly, enhancing the quality of recommendations over time.
AI and ML
The bedrock of the NBA system is undoubtedly AI and Machine Learning. These formidable technologies process torrents of data, ferreting out patterns in customer behavior, inclinations, and interactions. Machine learning models undergo training on historical data and then face the crucible of uncharted data, guaranteeing precise predictions. These models employ algorithms with real-time analytical prowess, enabling them to make judicious decisions about preferred channels, optimal engagement timings, and the content, messages, or offers to present.
Recommendations
NBA results in predefined responses or actions, given to people or machines to help them know what to do next when dealing with customers. These recommendations reside as standard objects, holding images, textual values, custom fields, and assigned workflows. The essence of this process rests in meticulous planning—the circumstances dictating their appearance and the targeted recipients. A well-defined automation plan comes to the fore in case of customer acceptance.
Flows
Recommendations are guided by “flows”. These mechanized processes ensure that the right recommendations find their way to the right individuals at the right time, all guided by the parameters of business criteria. A user-friendly flow builder, with an intuitive visual interface, simplifies the creation of these flows. For instance, a flow may be triggered when a customer visits a specific product page, prompting the suggestion of a related product.
Action Strategies
Action strategies serve as guides for agents, helping them decide which recommendations to prioritize and follow. Rooted in data and business logic, these strategies are bespoke for different scenarios, user segments, and departments. They emerge from both the Flow builder and the Strategy builder. The latter, with its unique elements like recommendations, recommendation logic, and branch logic, facilitates the art of declarative recommendation creation.
Let’s now learn about this process in more detail:
Planning Recommendations and Automation: The expedition commences with meticulous planning—where recommendations should surface, who the recipients are, the conditions governing each recommendation, and the route to traverse upon acceptance. This phase is pivotal, ensuring that the NBA system unfailingly serves up pertinent and timely recommendations.
Creating Flows: Flows are meticulously crafted with a flow builder. These automated routines define the actions triggered if a user accepts or rejects a recommendation. For example, a flow might be tailored to dispatch a follow-up email with a discount code when a customer abandons a shopping cart. Flows stand as gatekeepers, ensuring the opportune delivery of recommendations.
Creating Recommendations: In this phase, recommendations take shape, influenced by business rules, predictive models, and a diverse array of data sources. These recommendations are context-specific, factoring in a customer’s recent interactions, proclivities, and the prevailing market milieu. Machine learning models lend their prowess to further optimize these recommendations, guaranteeing relevance and timeliness.
Creating Action Strategies: The design of action strategies serves as the rudder determining when and how recommendations are presented. The choice lies between employing the Flow builder or the Strategy builder, with the latter boasting unique elements such as recommendations, recommendation logic, and branch logic. These components converge to facilitate the art of crafting personalized recommendations.
Displaying Recommendations: The crescendo unfolds when action strategies are brought to life. The ultimate step involves selecting the digital stage where recommendations are performed and showcased. This can manifest on e-commerce websites, within customer support chats, or at any other point of customer interaction.
Benefits of Next Best Action AI
Next Best Action AI technology is revolutionizing customer acquisition, relationship enhancement, and retention strategies across various industries. This innovative approach offers a myriad of benefits, including improved acquisition, enhanced customer relationships, and boosted customer retention.
Enhanced Customer Acquisition
Personalization at Scale: Traditional marketing efforts often struggle to achieve true 1:1 personalization due to limited data on individual prospects. AI, however, optimizes acquisition by refining ad targeting and placement. It continuously analyzes prospect behavior, updating segments dynamically and ensuring precise targeting.
Tailored Messaging: AI deciphers a prospect’s intent through content consumption and behavior analysis, enabling the delivery of tailored messages. Recommendation engines take personalization a step further by using machine learning to predict products of interest, significantly improving the chances of conversion.
Enhanced Customer Relationships
Targeted Customer Engagement: Imagine a leading national bank using Next Best Action AI to offer next-level customer experiences. Call center agents receive tailored product recommendations during each call, increasing the likelihood of conversion and fostering deeper engagement.
Intelligent Call Routing: The bank employs intelligent call routing to match customers with care agents possessing the specific skills needed for their queries. This reduces wait times, increases resolution rates, and creates a more satisfying customer experience.
Multi-Channel Engagement: The bank’s commitment to deepening customer engagement extends to multiple touchpoints, such as ATMs, email, SMS, and more, offering consistent and personalized interactions regardless of the customer’s preferred channel.
Increased Loyalty and Conversion: Personalized experiences demonstrate a commitment to customers’ unique needs, fostering a sense of being valued and driving increased loyalty. Tailored product recommendations resonate with customers, increasing conversion rates.
Boosting Retention
Proactive Churn Prevention: AI’s continuous monitoring of customer behavior enables proactive churn management by identifying patterns that indicate a customer might be considering leaving. This allows for targeted action to address customer needs and concerns, improving satisfaction and retention.
Targeted Customer Retention: By analyzing customer data, AI identifies customers more likely to churn, enabling organizations to focus on retaining higher-value customers who represent a significant loss if they were to leave.
Understanding Attrition Drivers: AI can identify the primary drivers of customer attrition, such as product dissatisfaction, poor customer service, or competitor offerings. Brands can take targeted actions to address these issues and prevent future churn.
Optimized Retention Budgets: AI technology provides insights into the effectiveness of retention efforts, allowing brands to allocate resources to the most successful strategies and maximize ROI.
Improved Customer Experience: Brands can enhance the customer experience by understanding the factors influencing retention, thereby increasing customer loyalty and reducing churn.
Increased Customer Lifetime Value: Retaining higher-value customers boosts customer lifetime value, leading to long-term revenue growth and profitability.
Technology Stack to consider
Features | Description |
Data Collection and Storage | Databases: Use databases such as SQL (e.g., PostgreSQL, MySQL) for structured data and NoSQL (e.g., MongoDB, Cassandra) for unstructured data.Data Warehousing: Tools like Amazon Redshift or Google BigQuery can be useful for large-scale data warehousing. |
Data Processing | ETL (Extract, Transform, Load): ETL tools like Apache Nifi or Talend can help in data preprocessing and transformation.Big Data Frameworks: For handling large datasets, Apache Hadoop and Apache Spark are commonly used. |
Machine Learning and AI | Machine Learning Libraries: Python libraries like TensorFlow, PyTorch, and scikit-learn for developing and training machine learning models.AI Frameworks: Utilize AI platforms like IBM Watson, Google AI, or Microsoft Azure AI, which offer pre-built AI and machine learning services. |
Recommendation Engines | Collaborative Filtering: Implement collaborative filtering algorithms like user-based and item-based filtering.Content-Based Filtering: Use content-based recommendation models that rely on user preferences and item attributes.Hybrid Approaches: Combine collaborative and content-based filtering for more accurate recommendations. |
Decision Engines | Rules Engine: Implement business rules and logic to determine the “next best action” based on the recommendation.Reinforcement Learning: Use reinforcement learning for decision-making in dynamic environments. |
Real-time Data Processing | Stream Processing: Apache Kafka or Apache Flink can be used to process real-time data and events.Message Queues: Tools like RabbitMQ or Apache ActiveMQ for handling asynchronous messaging. |
Scalability and Deployment | Containerization: Docker and container orchestration with Kubernetes for scalable and efficient deployment.Serverless Computing: Services like AWS Lambda or Azure Functions can handle functions on-demand.Microservices Architecture: Develop the system as microservices for modularity and scalability. |
Data Visualization | Data Visualization Tools: Use tools like Tableau, Power BI, or Matplotlib/Seaborn for creating interactive dashboards and visualizing recommendations and actions. |
Monitoring and Analytics | Logging and Monitoring Tools: Implement monitoring with tools like Prometheus, Grafana, and ELK Stack (Elasticsearch, Logstash, Kibana).Analytics: Use tools like Google Analytics or Mixpanel to monitor user interactions and feedback. |
Next Best Action AI case studies
Case Study 1: Healthcare – Personalized Treatment Recommendations
Introduction:
In the healthcare sector, Next Best Action AI plays a critical role in providing personalized treatment recommendations to improve patient outcomes. One real-world example is the deployment of IBM Watson for Oncology at Memorial Sloan Kettering Cancer Center (MSKCC) in New York.
Challenges:
MSKCC faced challenges in keeping up with the ever-expanding body of medical knowledge and delivering consistent, evidence-based cancer treatment recommendations to patients.
Solution:
IBM Watson for Oncology is an AI system designed to assist oncologists in making treatment decisions. It analyzes a patient’s medical records, including their medical history, test results, and the latest clinical research, to recommend personalized treatment plans. This ensures that patients receive the most appropriate and up-to-date care.
Results:
- By utilizing Next Best Action AI, MSKCC improved the consistency and quality of treatment recommendations across its network of healthcare providers.
- The AI system significantly reduced the time required for oncologists to research and develop personalized treatment plans, allowing them to focus more on patient care.
- Patients benefited from more precise and evidence-based treatment options, ultimately improving their chances of recovery and quality of life.
Case Study 2: Financial Services – Personalized Investment Advice
Introduction:
The financial services industry utilizes Next Best Action AI to provide personalized investment recommendations to clients. One prominent example is the wealth management platform, Wealthfront.
Challenges:
Wealthfront faced the challenge of delivering tailored investment strategies to a diverse client base with varying financial goals and risk tolerances.
Solution:
Wealthfront’s Next Best Action AI system assesses each client’s financial situation, goals, and risk preferences through a detailed questionnaire. Based on this information, it creates a personalized investment portfolio using a combination of ETFs (Exchange-Traded Funds). The system continuously monitors market conditions and the client’s financial situation to make dynamic portfolio adjustments.
Results:
- Clients of Wealthfront benefit from personalized investment strategies that align with their specific financial goals and risk profiles.
- The AI system provides ongoing portfolio management, optimizing investments as market conditions change, thus enhancing clients’ potential for long-term returns.
- Wealthfront has seen significant growth in its client base, thanks to its ability to provide scalable, personalized investment advice using Next Best Action AI.
Case Study 3: Retail – E-commerce Personalization
Introduction:
In the highly competitive e-commerce industry, providing personalized shopping experiences is crucial. Amazon, one of the world’s largest e-commerce platforms, has harnessed the power of Next Best Action AI to recommend products to its customers.
Challenges:
Amazon needed to enhance customer engagement and boost sales by suggesting relevant products to its vast customer base.
Solution:
Amazon’s recommendation engine, often referred to as Amazon Personalize, employs Next Best Action AI to analyze customer behavior, purchase history, and browsing patterns. It then generates personalized product recommendations, which can be seen on the Amazon website and in emails. This provides a tailored shopping experience for each user.
Results:
- By using Next Best Action AI, Amazon has seen a significant increase in customer engagement and sales. Customers are more likely to purchase products that are recommended based on their preferences.
- The AI system helps Amazon cross-sell and upsell products, boosting revenue and customer satisfaction.
- Amazon Personalize has contributed to Amazon’s dominant position in the e-commerce market by ensuring that customers keep returning for personalized shopping experiences.
Case Study 4: Telecommunications – Customer Retention and Upselling
Introduction:
Telecom companies face challenges in retaining customers and growing their revenue. Airtel, a leading telecommunications provider in India, has successfully implemented Next Best Action AI to address these challenges.
Challenges:
Airtel needed a way to reduce customer churn and increase revenue by offering the right services to the right customers at the right time.
Solution:
Airtel deployed a Next Best Action AI system that analyzes customer usage patterns, billing history, and preferences. Based on this data, it recommends personalized offers, such as data plans, voice packs, or additional services, to individual customers. These recommendations are delivered through various channels, including SMS and mobile apps.
Results:
- Airtel’s Next Best Action AI system has significantly reduced customer churn by offering tailored promotions and services that match each customer’s needs.
- Revenue per customer has increased as a result of upselling relevant products and services.
- Airtel’s ability to provide a personalized customer experience has helped the company maintain its competitive edge in the highly competitive telecommunications market.
Conclusion
Next Best Action AI is poised to transform industries, streamlining operations and boosting outcomes. While initial implementation demands an internal review and investment, the rewards of improved response times, content accuracy, and cost savings far outweigh the initial effort. With evolving AI tech, machine learning and deep learning capabilities will supercharge recommendations. However, success hinges not just on tech but on organizational adaptability, strong strategy, robust datasets, executive support, and broad buy-in. By embracing AI holistically, organizations can optimize decision-making, fostering growth, innovation, and customer satisfaction.
How Ideausher can help
Ready to embark on your journey into Next Best Action AI innovation? Take the first step by clicking the ‘Connect with Us’ button below. At Idea Usher, we specialize in bringing your visionary ideas to life. Begin your Next Best Action AI project today with our ‘AI Development’ service. Our team creates dynamic solutions with responsive designs, real-time capabilities, and seamless mobile optimization. We offer a wide range of services and customization options, all while being budget-friendly. Choosing Idea Usher ensures your vision becomes a high-performing reality.
Click here!
Hire ex-FANG developers, with combined 50000+ coding hours experience
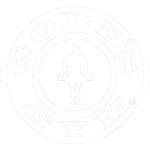
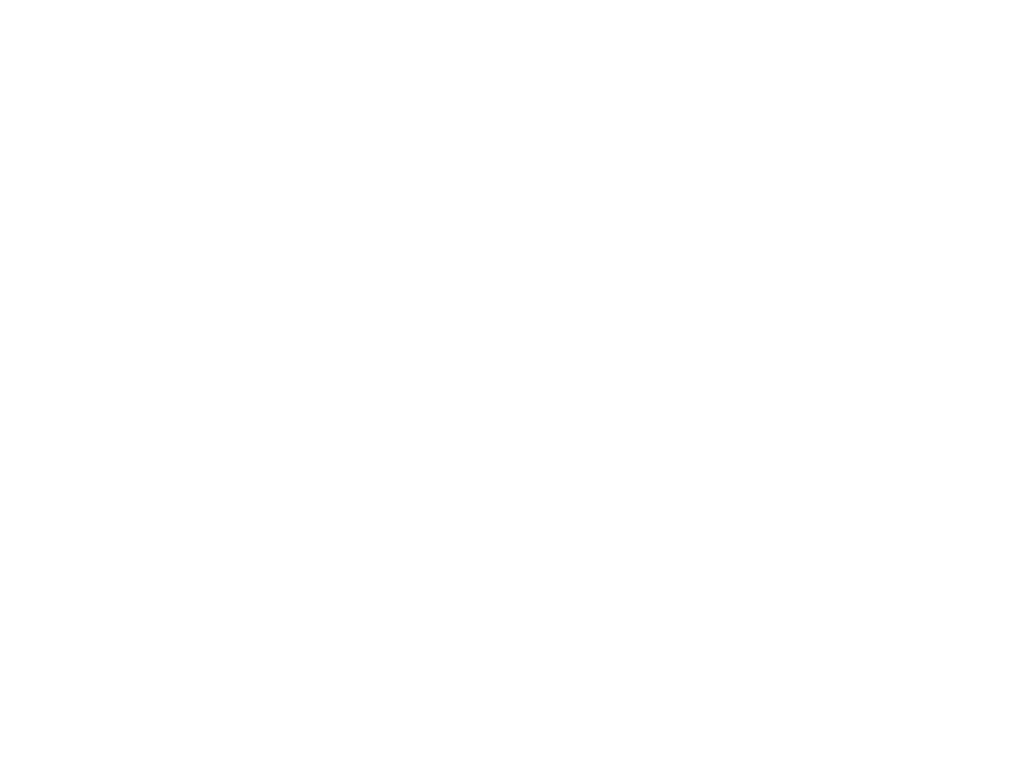
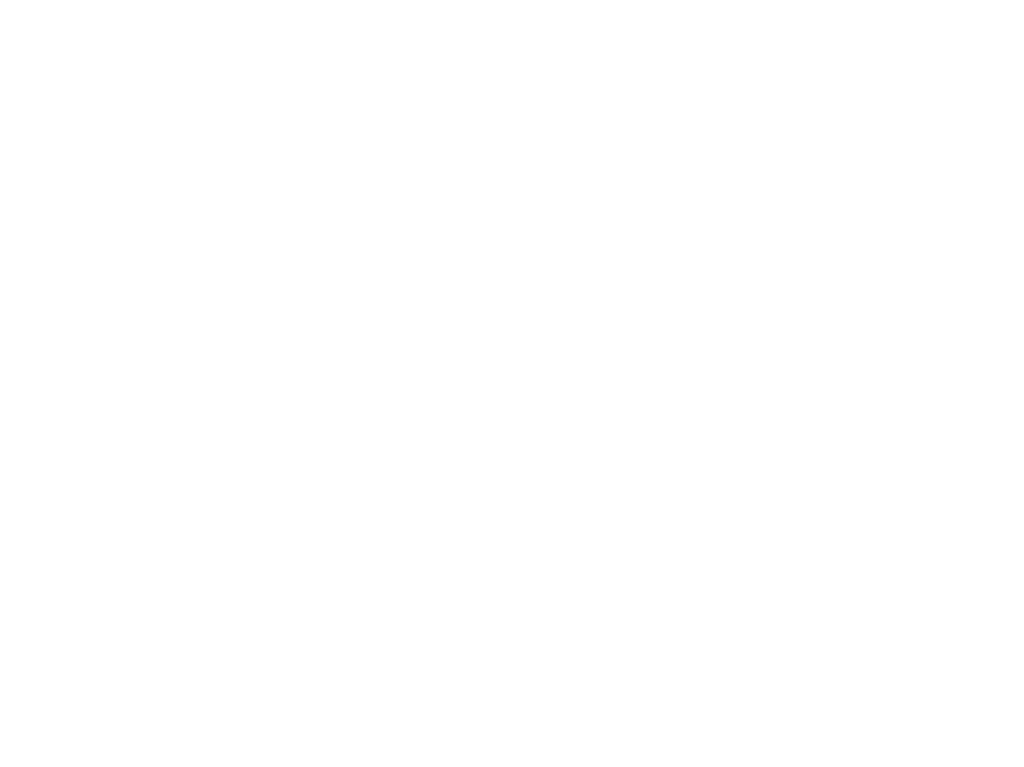
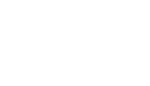
Contact Idea Usher
FAQs
Q. What is Next Best Action AI, and how does it work?
A. Learn about the fundamental principles of Next Best Action AI and its mechanisms for making intelligent recommendations.
Q. How is Next Best Action AI transforming industries?
A. Explore real-world examples of how businesses across various sectors are leveraging Next Best Action AI to enhance customer experiences and drive efficiency.
Q. What are the key challenges in implementing Next Best Action AI solutions?
A. Delve into the potential hurdles and considerations when integrating Next Best Action AI systems into your organization.
Q. What ethical concerns surround the use of Next Best Action AI?
A. Discuss the ethical implications of AI-driven decision-making and the importance of responsible AI deployment.