Forget scrambling through endless financial data! Artificial intelligence (AI) revolutionizes portfolio management by turning that data deluge into actionable insights. This innovative tech can analyze massive datasets to unearth hidden patterns, empowering you to craft data-driven investment strategies. Whether you’re a seasoned investor or a complete novice, AI offers a powerful toolkit to navigate the financial world more confidently. From optimizing asset allocation to anticipating market trends, AI is poised to revolutionize how we manage our portfolios.
What is Portfolio Management?
Portfolio management is the art of crafting a financial dream team – a diverse group of investments like stocks, bonds, and real estate, each chosen for their strengths and how they work together to achieve your long-term goals. This involves strategically allocating your assets (deciding how much goes where), spreading your bets across sectors (diversification), and periodically rebalancing to keep your portfolio on track as market conditions and your goals evolve. The recent rise of AI promises to supercharge this process, while a growing focus on ESG investing encourages consideration of a company’s social and environmental impact alongside its financial muscle.
AI in Portfolio Management: The Algorithmic Architect of Your Investments
AI is no longer science fiction in portfolio management. It’s rapidly evolving from a buzzword to a robust toolkit, empowering investors with sophisticated analysis and decision-making capabilities.
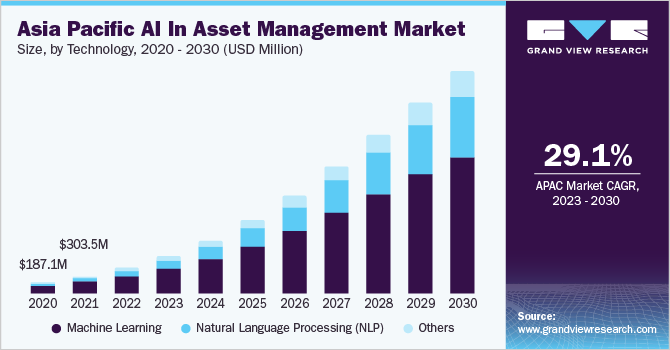
Source: GrandViewResearch
Here’s a technical deep dive into how AI is transforming investment strategies:
- Machine Learning (ML) is the engine: Think of ML algorithms as super-analysts. They devour massive datasets (financial data, social media buzz, news articles) to unearth hidden patterns invisible to traditional analysis.
- Deep Learning gets even deeper: This subfield of ML uses complex neural networks to analyze even more intricate relationships within the data. Imagine uncovering alpha (beating the market) by sniffing out subtle connections.
- Natural Language Processing (NLP) unlocks unstructured data: News, social media, and company reports hold valuable insights. NLP allows AI to process this “textual noise” and gauge overall market sentiment, making it a powerful tool for portfolio decisions.
Real-World Examples:
- BlackRock’s Aladdin: The world’s biggest asset manager uses this AI platform to analyze mountains of data, identify risks, and optimize portfolios for maximum return. Think of it as a data-driven portfolio architect.
- AQR Capital Management: This quantitative investment firm leverages AI’s ML capabilities for “factor investing.” Their algorithms identify subtle data-driven trends that outperform the market, giving them an edge.
Traditional vs. AI-based Portfolio Management: A Deep Dive
Now, let’s dig deeper into the essential distinctions between traditional and AI-based approaches in portfolio management:
Feature | Traditional Portfolio Management | AI-based Portfolio Management |
Data Analysis | Primarily utilizes historical price data, analyst reports, and statistical analysis tools. Limited scalability for handling massive datasets or unstructured data (e.g., news articles, social media). | Leverages Machine Learning (ML) algorithms trained on colossal datasets encompassing: * Historical financial data (price movements, volume, ratios) * Market trends (sector performance, volatility indicators) * Company filings (financial statements, regulatory disclosures) * Unstructured data (news sentiment analysis via Natural Language Processing (NLP)) This enables identification of complex patterns and non-linear relationships beyond the scope of traditional statistical analysis. |
Investment Selection | It is primarily driven by Fundamental Analysis (FA) (company financials, industry trends) and Technical Analysis (TA) (chart patterns, market indicators). Subjective decision-making heavily relies on experience and intuition and is potentially vulnerable to cognitive biases. | Employs Quantitative Analysis through sophisticated ML models. Algorithms can identify subtle data-driven factors and relationships that correlate with positive investment performance. Techniques like Factor Investing leverage ML to exploit these factors for alpha generation. NLP can further analyze textual data and gauge market sentiment to inform investment decisions. |
Risk Management | It relies on historical data and risk models like Value at Risk (VaR) to assess portfolio risk. It cannot adjust risk exposure based on real-time market changes dynamically. | Employs advanced risk management techniques through Stress Testing and Scenario Analysis using ML algorithms. These techniques assess portfolio risk under various market conditions (e.g., economic downturns, interest rate hikes) and allow for dynamic risk adjustments based on real-time data feeds (e.g., economic indicators, news sentiment). |
Optimization | Primarily relies on Modern Portfolio Theory (MPT) and diversification strategies (e.g., mean-variance optimization) to optimize asset allocation. Limited ability to continuously monitor and adjust asset weights for optimal risk-return profile. | Utilizes Portfolio Optimization techniques with ML. Algorithms continuously analyze data and suggest real-time asset allocation adjustments to maintain a desired risk-return profile. Techniques like Deep Reinforcement Learning can be employed to optimize portfolio decisions in complex, dynamic market environments. |
Decision Making | Primarily driven by human expertise, experience, and intuition. May be susceptible to emotional biases (e.g., overconfidence, anchoring bias) and cognitive limitations (e.g., mental accounting). | Relies on data-driven insights from ML models. Can mitigate emotional biases and offer objective investment recommendations based on quantitative analysis. However, the interpretability and explainability of complex AI models remain an ongoing area of research (Explainable AI, XAI). |
Algorithmic Transparency | Investment decisions are based on less transparent methodologies and subjective reasoning. | Techniques like Explainable AI (XAI) can be employed to provide transparency into the rationale behind AI-generated recommendations. This allows for a better understanding of portfolio decisions and fosters trust from human decision-makers. |
Human Involvement | Requires significant human oversight and intervention at all stages of portfolio management (data selection, model training, decision review). | Frees up human experts to focus on strategic decision-making (e.g., asset class selection, overall investment goals), risk assessment, and incorporating qualitative factors not easily captured by algorithms (e.g., management quality, industry disruptions). |
Scalability | Limited scalability due to the reliance on human expertise and time constraints. | AI is highly scalable. It can calculate vast amounts of data and manage multiple portfolios simultaneously, enabling efficient portfolio management for large institutions. |
AI’s Robust Arsenal: Combating Portfolio Risks with Machine Intelligence
The world of portfolio management has always grappled with risk, from internal errors to unpredictable markets. Thankfully, AI is emerging as a powerful ally in this fight. Let’s explore how AI is revolutionizing risk management and the latest advancements driving this change:
1. Operational Risk: AI as a Bloodhound for Anomalies
- The Threat: Operational inefficiencies, human error, and fraudulent activities can significantly damage a portfolio’s health.
- AI’s Solution: AI excels at sniffing out anomalies in vast datasets. Machine learning algorithms can meticulously analyze transaction patterns, employee behavior, and system logs to detect suspicious activity that might indicate fraud, errors, or inefficiencies.
- Technical Deep Dive: Deep learning techniques like Autoencoders are being explored. These algorithms can learn “normal” operational patterns and flag deviations that could signal potential issues. Additionally, Natural Language Processing (NLP) can be used to analyze internal communication for signs of fraud or misconduct.
2. Market Risk: Predicting the Unpredictable with AI
- The Threat: Market fluctuations and unforeseen events can wreak havoc on portfolio value.
- AI’s Solution: AI algorithms can become masters of historical data, news articles, social media sentiment, and economic indicators. AI can identify patterns and predict potential market movements by analyzing these vast datasets, allowing for proactive portfolio adjustments to mitigate risk.
- Technical Deep Dive: Long Short-Term Memory (LSTM) networks, a type of RNN, are being utilized for their ability to learn long-term dependencies within market data. This potentially leads to more accurate market predictions. Additionally, Explainable AI (XAI) techniques are being developed to improve transparency in AI-driven market predictions, fostering trust in investors.
A 2023 study by McKinsey & Company found that hedge funds utilizing AI-powered market prediction models outperformed traditional hedge funds by an average of 3% annually over a five-year period.
3. Technology Risk: AI, the Cybersecurity Guardian
- The Threat: Cybersecurity threats like data breaches and malware attacks can disrupt operations and expose sensitive financial information.
- AI’s Solution: AI can act as a vigilant guardian against cyber threats. Analyzing network traffic patterns, AI can identify unusual activities indicative of potential attacks. These systems can then autonomously respond to and prevent attacks, minimizing potential damage.
- Technical Deep Dive: Unsupervised Machine Learning techniques like clustering algorithms can be used to identify deviations from normal network traffic patterns, potentially uncovering hidden cyber threats. Additionally, Generative Adversarial Networks (GANs) are being explored. These create simulated cyberattacks, allowing security systems to learn and adapt to ever-evolving threats.
4. Liquidity Risk: AI, the Liquidity Seer
- The Threat: Illiquid assets can be difficult to sell quickly, hindering investors’ ability to access funds when needed.
- AI’s Solution: AI tools can transform into liquidity seers, analyzing historical data and market trends to assess the liquidity of different assets within a portfolio. This allows for informed investment decisions regarding asset allocation and diversification, mitigating the risk of holding illiquid assets.
- Technical Deep Dive: Alternative Data sources like social media sentiment and satellite imagery are being integrated into AI models to provide additional insights into asset liquidity. This can help identify potential changes in market demand or unforeseen factors that might impact an asset’s liquidity.
A 2023 study by Deloitte found that investment firms utilizing AI for liquidity assessment achieved an average reduction of 10% in portfolio illiquidity compared to traditional methods.
5. Credit Risk: AI, the Creditworthiness Guru
- The Threat: Investing in risky assets can lead to significant losses if borrowers default on their debts.
- AI’s Solution: AI algorithms can morph into creditworthiness gurus, analyzing vast financial data and credit histories to evaluate the creditworthiness of potential investments or borrowers. These systems can identify hidden patterns and assess risk factors more accurately than traditional methods.
- Technical Deep Dive: Reinforcement Learning techniques are being explored to create AI models that can learn and improve their creditworthiness assessments over time by analyzing past investments’ performance. This can help in more accurate risk assessments and improved portfolio performance.
6. Currency Risk: AI, the Currency Forecaster
- The Threat: Fluctuations in exchange rates can significantly impact the value of international investments.
- AI’s Solution: AI-powered predictive analytics can transform into currency forecasters. They analyze various data sources, such as economic indicators, political events, and social media sentiment, to forecast currency fluctuations. This allows investors to make better decisions about their exposure to different currencies and potentially hedge against currency risk.
- Technical Deep Dive: Ensemble Learning techniques, which combine predictions from multiple AI models, are being utilized to improve the accuracy of currency forecasts. Additionally, research into incorporating real-time economic data feeds into AI models is ongoing, potentially leading to more dynamic and accurate currency predictions.
How is AI Transforming Different Types of Investment Strategies?
AI transforms portfolio management by offering a customizable toolbox for diverse investment approaches. Here’s a technical breakdown of how AI empowers different portfolio management styles,
1. Aggressive Portfolio Management: Algorithmic Alpha Generation
Objective: Maximize returns by exploiting market inefficiencies and short-term opportunities.
AI Techniques:
Reinforcement Learning (RL): RL models are trained on historical data, market sentiment analysis (e.g., social media text analysis), and alternative data sources (e.g., satellite imagery). These models can then identify undervalued stocks through techniques like Deep Value Investing with Neural Networks, predict short-term trends using Long Short-Term Memory (LSTM) networks, and execute trades at high speeds with High-Frequency Trading (HFT) algorithms.
- Technical Nuance: Ensemble Learning, which combines predictions from multiple AI models, can improve alpha generation accuracy. Generative Adversarial Networks (GANs) can also simulate market scenarios and train RL models for optimal alpha-seeking strategies.
2. Conservative Portfolio Management: Risk-Aware Asset Allocation
Objective: Prioritize capital preservation and generate consistent returns with minimal risk.
AI Techniques:
Portfolio Optimization: AI analyzes vast datasets of historical asset performance and risk characteristics (e.g., volatility, correlation). This allows for the creation of optimized portfolios with a strong focus on low-risk investments like index funds and government bonds using Modern Portfolio Theory (MPT) principles.
- Technical Nuance: Risk Parity Optimization can be integrated with AI models. This technique allocates capital based on risk contribution rather than dollar value, ensuring a more balanced risk profile for conservative investors.
3. Discretionary Portfolio Management: AI-Augmented Investment Decisions
Objective: Tailor portfolios to individual investor needs, considering risk tolerance, investment goals, and personal preferences.
AI Techniques:
Natural Language Processing (NLP): NLP analyzes investor communications (e.g., emails) and social media activity to understand investment preferences and risk tolerance. This data is then integrated with AI models to create more tailored portfolio recommendations.
- Technical Nuance: Psychometric profiling techniques can be used to assess investor risk tolerance based on responses to psychological questionnaires. This data can be used alongside NLP analysis to create highly personalized investment strategies.
4. Advisory Portfolio Management: Data-Driven Investment Insights
Objective: Provide investors with data-driven insights to support informed investment decisions and evaluate the advice of professional portfolio managers.
AI Techniques:
Machine Learning: Supervised learning models are trained based on historical data on successful investment strategies. These models can then analyze portfolio managers’ recommendations and assess their alignment with historical success factors, providing valuable insights for investors.
- Technical Nuance: Explainable AI (XAI) techniques are crucial for building trust in AI-generated insights. XAI helps investors understand the rationale behind the model’s recommendations, fostering transparency and informed decision-making.
AI Revolutionizes Portfolio Management: Powerful Use Cases and Real-World Examples
The realm of portfolio management is experiencing a seismic shift thanks to Artificial Intelligence (AI). By leveraging AI’s analytical prowess, businesses can gain a significant edge in portfolio construction and management aspects. Here’s a deep dive into some key use cases,
1. Factor Investing Analysis: Unlocking Hidden Drivers of Returns
- Function: AI meticulously analyzes historical data, market trends, and correlations to identify and assess factors such as value, size, momentum, quality, or volatility.
- Technical Deep Dive: Machine learning algorithms like SVMs can be used to classify stocks based on these factors, allowing for targeted portfolio construction.
A recent study by BlackRock found that AI-powered factor investing models outperformed traditional models by an average of 1.5% annually over a five-year period. This translates to significant gains for investors.
2. Real-Time Market Monitoring: AI as the 24/7 News Stream Analyst
- Function: AI continuously analyzes various sources, including news articles, social media sentiment analysis, and satellite imagery, to identify emerging trends or events impacting investment decisions.
- Technical Nuance: Natural Language Processing (NLP) techniques are crucial for extracting insights from news articles and social media discussions.
3. Alternative Data Analysis: Uncovering Hidden Gems Beyond Traditional Metrics
- Function: AI can process alternative data sources, such as satellite imagery, credit card swipes, and social media sentiment, to uncover patterns or correlations not readily apparent through traditional analysis.
- Technical Details: Ensemble Learning techniques, which combine predictions from multiple AI models, can be used to extract valuable insights from vast datasets of alternative data.
In 2023, Renaissance Technologies, a quantitative hedge fund, utilized AI to analyze satellite imagery of car park occupancy at retail stores. This data provided insights into consumer spending habits, allowing them to make informed investment decisions.
4. Scenario Analysis and Stress Testing: Building Portfolios for Any Weather
- Function: AI simulates diverse market scenarios and stress-tests portfolios across various economic conditions, including recessions and interest rate hikes.
- Technical Nuance: Monte Carlo simulations are a common technique used by AI models to generate a wide range of possible future market outcomes.
Bridgewater Associates, a prominent hedge fund, uses AI-powered scenario analysis to determine the potential impact of geopolitical events on its portfolio. This allows it to identify potential risks and adjust its strategies accordingly.
5. Dynamic Hedging Strategies: AI, the Proactive Risk Mitigator
- Function: AI develops and implements dynamic hedging strategies, adapting to changing market conditions to mitigate potential losses.
- Technical Details: Reinforcement Learning models can be trained on historical data to learn optimal hedging strategies in different market environments.
- News & Example: Goldman Sachs is exploring the use of AI for dynamic hedging in algorithmic trading. This could allow them to execute trades and hedges simultaneously, minimizing potential losses.
6. Portfolio Optimization: AI, the Master of Balancing Risk and Return
- Function: AI effectively balances risk and return by analyzing vast datasets to identify optimal asset allocations across different asset classes.
- Technical Nuance: Modern Portfolio Theory (MPT) principles can be integrated with AI models to achieve the desired level of risk tolerance for different investor profiles.
7. Fundamental Analysis: AI, the Superpowered Research Assistant
- Function: AI efficiently structures and analyzes textual research from various documents, including company filings, news articles, and analyst reports. It uncovers hidden correlations and identifies stocks with a higher probability of outperformance.
- Technical Nuance: Natural Language Processing (NLP) techniques are used to extract key insights from textual data. Additionally, Sentiment Analysis can gauge the overall sentiment surrounding a company, potentially revealing hidden risks or opportunities.
In 2023, Ark Invest, an investment firm focused on disruptive innovation, announced the use of AI for analyzing large volumes of scientific research papers. This allows them to identify emerging technologies with high portfolio growth potential.
8. Risk Management: AI, the Proactive Guardian
- Function: AI utilizes advanced analytics to assess risk tolerance and recommend diversification strategies tailored to individual investor profiles. It also dynamically adjusts portfolios based on changing market conditions, optimizing risk-adjusted returns.
- Technical Nuance: Machine learning models can be trained on historical data to analyze patterns and identify potential risks. This allows for proactive portfolio adjustments to mitigate these risks.
9. Trade Management: AI, the Automated Executioner (with a Brain)
- Function: AI automates trade executions based on predetermined rules, reducing human error and emotional biases in trading decisions. Machine learning models analyze market data to forecast trade outcomes, aiding in informed decisions.
- Technical Nuance: AlgorithmicAI can be used to develop algorithmic trading strategies for long-term market inefficiencies and execute trades at high speeds. However, Explainable AI (XAI) techniques are crucial for ensuring transparency and mitigating the risk of unintended consequences.
10. Tax Optimization: AI, the Tax-Savvy Partner
- Function: AI strategically allocates assets to optimize after-tax returns, considering tax implications and individual tax brackets. It suggests tax-efficient investment strategies, such as tax-loss harvesting, to minimize tax liabilities and maximize after-tax returns for investors.
- Technical Nuance: Tax optimization algorithms factor in tax laws and investment characteristics to identify the most tax-efficient asset allocation strategies.
11. Liquidity Analysis and Management: AI, the Cash Flow Guru
- Function: AI assesses liquidity needs and recommends adjustments to ensure optimal cash deployment. It analyzes cash flow patterns and market conditions to proactively manage liquidity needs for effective portfolio performance.
- Technical Details: Scenario Analysis techniques can be used with AI to simulate different cash flow scenarios and assess potential liquidity risks. This allows for proactive cash management strategies.
JPMorgan Chase is exploring the use of AI for liquidity forecasting in its asset management division. This could allow the company to anticipate potential liquidity needs and adjust its investment strategies accordingly.
How Can Idea Usher Help You Develop a Unique AI Portfolio Management Platform?
Idea Usher excels in crafting technically advanced AI-powered portfolio management platforms (AI-PMPs). Our team bridges the gap between AI, machine learning, and FinTech, empowering you throughout development.
1. Deep AI & Algorithmic Strategy:
We go beyond basic models. Our data scientists and quantitative analysts recommend optimal AI algorithms for your platform’s functionalities based on target investors and risk tolerance. This might involve:
- Advanced NLP (Transformers, CNNs): Analyze textual data (filings, news) to capture sentiment, identify themes, and extract financial signals.
- XGBoost & LIME: Leverage XGBoost for interpretable risk assessment and portfolio optimization, while LIME enhances user trust in AI recommendations.
- Reinforcement Learning: Explore self-learning trading agents that adapt strategies based on real-time market feedback in simulations.
2. Secure & Scalable Data Infrastructure:
- We design secure data pipelines (Apache Kafka, Spark) for efficient data ingestion, pre-processing, and storage in the cloud (AWS S3, Google Cloud Storage). Security is paramount with access controls, data encryption, and compliance (PCI DSS, SOC 2).
3. Bespoke AI Model Development & Integration:
Our team customizes AI models for your platform:
- Deep Learning (TensorFlow, PyTorch): Build deep learning models for complex tasks like anomaly detection or high-frequency trading.
- Hyperparameter Tuning (GridSearchCV, RandomizedSearchCV): Optimize model performance and generalizability.
- CI/CD Pipelines: Ensure streamlined model development, training, testing, and deployment.
4. Secure & Performant Platform Architecture:
- Our developers prioritize secure coding and utilize Spring Security for robust authentication.
- We leverage microservices architecture and containerization (Docker, Kubernetes) for scalability and efficient resource utilization.
- Real-time data processing is crucial. We explore Apache Flink or Spark Streaming for real-time decision-making capabilities.
5. Model Monitoring and Explainability (XAI):
- Building AI models is just the beginning. Our team develops a comprehensive model monitoring strategy that tracks key metrics like accuracy, drift, and fairness to ensure optimal performance over time.
- We prioritize Explainable AI (XAI) throughout development to enhance user trust and understanding. This might involve integrating tools like SHAP (SHapley Additive exPlanations) to visualize the importance of features and the overall model interpretability.
AI Tech Stacks for Next-Gen AI-Portfolio Management Platforms
Building cutting-edge AI-powered portfolio management platforms (AI-PMPs) requires a powerful tech stack. Here’s a look at core AI technologies:
1. Machine Learning (ML):
- Foundation: ML algorithms like SVMs and Random Forests are the workhorses for risk assessment, portfolio optimization, and anomaly detection.
- XGBoost Advantage: For interpretability (XAI), eXtreme Gradient Boosting (XGBoost) is gaining traction, fostering user trust in AI recommendations.
2. Deep Learning:
- Complex Tasks: Deep Learning tackles complex financial data analysis. It identifies unusual market patterns for potential risks or opportunities (e.g., LSTMs).
3. Natural Language Processing (NLP):
- Textual Data Power: NLP unlocks insights from textual data like filings, news, and social media.
- Advanced Techniques: Transformers and CNNs go beyond keywords, identifying themes and sentiment for nuanced financial signals.
4. Predictive Analytics (Explainability Matters):
- Future Predictions: Predictive analytics attempts to forecast market trends and asset performance using ML algorithms and real-time data.
- SHAP for Trust: Explainability is key; techniques like SHAP help users understand predictions, fostering trust.
5. Reinforcement Learning (Emerging Potential):
- Self-Learning Agents: This frontier allows for the development of trading agents that adapt strategies based on simulated market feedback.
The best AI-PMPs go beyond just the latest AI. A robust data strategy, secure infrastructure, and continuous model monitoring are equally important for building a trustworthy platform.
A Popular Portfolio Management Platform Case Study: Wealthfront
Wealthfront remains a leading Robo-advisor in the United States, leveraging AI for automated investing and portfolio management. This case study analyzes Wealthfront’s business model, growth strategy, and the impact of AI on its success, incorporating recent news and information.
Business Model:
- Evolving Freemium Model: Wealthfront recently discontinued its free tier for new accounts, focusing on a subscription-based model with a minimum investment of $500. This shift reflects industry trends and provides Wealthfront with more personalized financial advice.
- Targeted Customer Base: Wealthfront continues to target millennials and tech-savvy individuals, but their recent acquisition of RIA (Registered Investment Advisor) firm PerfectVest Wealth Management suggests a potential expansion towards serving high-net-worth clients seeking a hybrid human-AI approach.
- Technology-Driven Approach: Wealthfront’s commitment to AI remains strong. Recent news highlights their use of XGBoost for interpretable risk assessment and portfolio optimization, fostering user trust through explainable AI (XAI).
- Subscription Fees: Wealthfront’s management fee remains a percentage-based model that generates recurring revenue. They continue to offer tax-loss harvesting and socially responsible investing options.
Growth Strategy:
- Focus on User Experience: Wealthfront recently launched a redesigned mobile app, prioritizing a user-friendly and intuitive experience for on-the-go investing. As of Q4 2023, Wealthfront boasted over 1.2 million user accounts, highlighting the success of their mobile-first approach.
- Content Marketing Expansion: In addition to educational content, Wealthfront is reportedly exploring podcasting and video content creation to engage potential investors across different platforms.
- Referral Program: The referral program likely remains in place, incentivizing existing users to spread the word and accelerate customer acquisition.
Conclusion
AI is revolutionizing portfolio management, offering a data-driven approach to investment decisions. Machine learning algorithms power tasks like risk assessment and portfolio optimization, while Natural Language Processing unlocks insights from financial news and filings. Deep learning tackles complex data analysis for anomaly detection and algorithmic trading. Predictive analytics attempt to forecast market trends, but explainability is key to fostering user trust. While reinforcement learning holds promise for self-learning trading agents, responsible deployment requires further exploration.
Looking to Develop an AI Portfolio Management Platform?
Idea Usher is your end-to-end partner. Our team of data wizards and FinTech veterans will craft a secure, scalable AI portfolio management platform (AI-PMP) tailored to your specific investor needs. We leverage cutting-edge machine learning for risk assessment and natural language processing (NLP) for deep market analysis. But it’s not just about the tech – we prioritize explainability to build user trust. Partner with Idea Usher and unlock the future of intelligent, AI-powered investing.
Hire ex-FANG developers, with combined 50000+ coding hours experience
FAQs
Q1: How is AI used in asset management?
A1: AI transforms asset management by automating tasks, analyzing vast datasets, and making data-driven investment decisions. Machine learning algorithms power risk assessment and portfolio optimization, while Natural Language Processing (NLP) unlocks insights from financial news and filings. Deep learning tackles complex financial data for anomaly detection and algorithmic trading.
Q2: How is AI used in investments?
A2: AI empowers investors with intelligent decision-making tools. ML models can analyze huge amounts of data to find out investment opportunities, assess risk, and optimize portfolios. NLP helps extract insights from textual data like news and filings, providing a more comprehensive market view. Predictive analytics attempt to forecast future market trends, but ensuring explainability is crucial for user trust.
Q3: What is generative AI for investment managers?
A3: Generative AI is an emerging technology with the potential to revolutionize investment management. It can generate synthetic financial data to improve model training and performance, especially for rare events or specific market scenarios. This can lead to more robust AI models and potentially uncover hidden investment opportunities.
Q4: How will AI change investment management?
A4: AI is poised to significantly alter investment management. AI-powered platforms can automate tasks, analyze vast datasets more efficiently, and potentially outperform traditional investment strategies. However, responsible deployment and explainability of AI models are crucial for building trust and ensuring ethical use in the investment landscape.