In today’s fast-paced business environment, corporations are always competing for dominance while managing market changes and uncertain customer preferences.
Demand forecasting becomes a crucial strategic tool in this competitive environment, giving businesses an insight into upcoming trends and helping them stay ahead of the competition.
Traditional demand forecasting methods, while valuable, often fall short in accuracy and scope. This is where Artificial Intelligence (AI) and Machine Learning (ML) come into play, revolutionizing forecasting by processing vast amounts of data at incredible speeds.
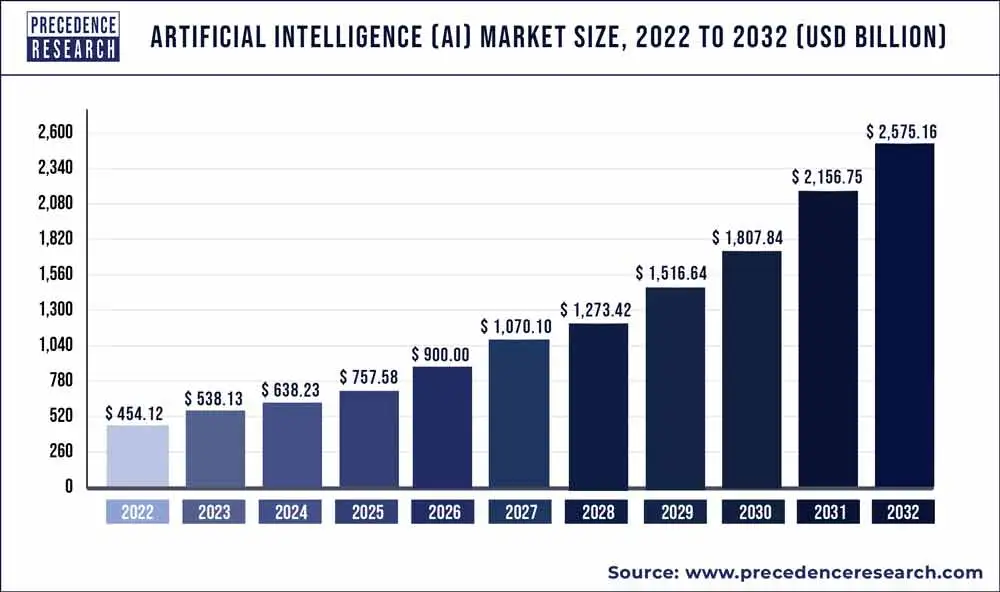
Source: Precedence Research
With companies realizing the benefits of accurate demand forecasting for improving supply chain management and operational effectiveness, the expanding artificial intelligence (AI) market offers experts in the field a vital toolkit for success in a dynamic business environment.
This article delves into the intersection of technology, business, and human behavior, exploring the advantages, and future direction of AI-driven demand forecasting.
What Is AI-Based Demand Forecasting?
AI-enabled demand forecasting is a revolutionary method that combines technology and strategy to enhance inventory management in today’s digital era. Unlike traditional approaches that rely solely on historical order data, AI in demand forecasting utilizes artificial intelligence to analyze a broader range of factors including market trends, social media activity, and online reviews.
By mining and identifying patterns within this diverse dataset, AI offers predictive insights that surpass the capabilities of conventional models.
Moreover, integrating machine learning into supply chain management further enhances these benefits. Unlike traditional models that rely solely on historical data, machine learning algorithms adapt and evolve in real-time, incorporating factors such as demographics, weather patterns, and social media trends to generate highly accurate forecasts.
One notable advantage of machine learning in demand forecasting is its ability to address challenges posed by new products. While traditional models struggle due to a lack of historical data, machine learning algorithms can identify similar product clusters, analyze their lifecycle patterns, and use this information to predict the trajectory of new products.
Overall, AI-enabled demand forecasting represents a paradigm shift in inventory management, combining advanced technology with strategic foresight to navigate the complexities of modern supply chains with confidence and precision.
Difference Between AI-Powered vs Traditional Demand Forecasting
AI-powered demand forecasting utilizes machine learning algorithms to analyze vast amounts of data, adapt to changing patterns, and provide more accurate predictions compared to traditional methods that rely on historical data and statistical models. Here are the differences in detail:
1. Data Handling
AI Powered Demand Forecasting: Artificial Intelligence (AI) systems excel in processing vast and heterogeneous datasets efficiently. Leveraging advanced algorithms, they can seamlessly handle diverse data types, including unstructured sources such as social media feeds, weather reports, and customer reviews. AI-driven approaches automate data preprocessing tasks, including cleaning, normalization, and feature extraction, streamlining the process and ensuring data quality.
Traditional Demand Forecasting: Traditional demand forecasting methods may struggle with large and complex datasets, often requiring manual effort for data preparation and cleansing. They typically rely on structured data sources and may encounter difficulties integrating unstructured data, which can limit their ability to capture nuanced market trends and dynamics.
2. Accuracy
AI Powered Demand Forecasting: AI-powered demand forecasting algorithms harness the power of machine learning and predictive analytics to deliver highly accurate forecasts. These algorithms can discern intricate patterns and relationships within historical data, enabling them to capture subtle market dynamics and anticipate demand fluctuations with precision.
Traditional Demand Forecasting: The accuracy of traditional demand forecasting methods may vary depending on factors such as the quality and representativeness of historical data, the choice of forecasting models, and the expertise of forecasters. While statistical techniques like time series analysis and regression can yield reasonably accurate predictions, they may struggle to capture complex nonlinear relationships and sudden shifts in demand patterns.
3. Adaptability
AI Powered Demand Forecasting: AI-driven demand forecasting systems exhibit remarkable adaptability to changing market conditions and evolving business landscapes. These systems can dynamically incorporate new data sources and adjust forecasting models in real-time, enabling organizations to respond swiftly to emerging trends, competitor actions, and external factors such as economic shifts or geopolitical events.
Traditional Demand Forecasting: Traditional forecasting approaches may lack the agility and responsiveness of AI-powered systems. Adjusting models to accommodate changing conditions often involves manual intervention, requiring forecasters to reevaluate assumptions, recalibrate parameters, and possibly overhaul forecasting methodologies.
4. Forecasting Speed
AI Powered Demand Forecasting: AI-driven demand forecasting solutions leverage parallel processing capabilities and advanced computational techniques to generate forecasts rapidly. By harnessing the computational power of modern hardware and distributed computing architectures, AI algorithms can crunch through massive datasets and execute complex analyses in a fraction of the time required by traditional methods.
Traditional Demand Forecasting: Forecasting with traditional methods may entail longer processing times, particularly when dealing with large datasets or computationally intensive models. The sequential nature of many traditional forecasting algorithms and the reliance on manual data manipulation contribute to slower turnaround times compared to AI-powered approaches.
5. Human Intervention
AI Powered Demand Forecasting: AI-enabled demand forecasting systems streamline forecasting workflows and minimize the need for human intervention. These systems automate routine tasks such as data preprocessing, model selection, and parameter tuning, freeing up human analysts to focus on higher-level decision-making and strategic initiatives. While human oversight remains essential for validating forecasts, interpreting results, and providing domain expertise, AI technologies reduce the overall cognitive load on forecasters.
Traditional Demand Forecasting: Traditional forecasting methods often rely heavily on human expertise and judgment throughout the forecasting process. Forecasters may need to manually inspect data for anomalies, select appropriate forecasting models, and fine-tune model parameters based on domain knowledge and intuition.
6. Scalability
AI Powered Demand Forecasting: AI-driven demand forecasting solutions offer inherent scalability, capable of handling large volumes of data and forecasting tasks across diverse product lines, markets, and geographic regions. These systems leverage parallel processing architectures and cloud-based infrastructure to scale resources dynamically in response to changing demand or computational requirements, ensuring optimal performance and responsiveness.
Traditional Demand Forecasting: Scaling traditional forecasting methods may pose challenges, particularly as data volumes and forecasting complexity increase. Traditional approaches often rely on single-threaded processing and on-premises infrastructure, which may struggle to accommodate growing workloads or support real-time forecasting needs. Scaling traditional methods may require significant investments in hardware, software, and human resources.
7. Cost Efficiency
AI Powered Demand Forecasting: While the initial setup costs of implementing AI-powered demand forecasting solutions may be higher than traditional methods, they offer long-term cost efficiency through automation, accuracy improvements, and operational savings. By reducing manual effort, minimizing forecast errors, and enabling more informed decision-making, AI-driven systems deliver tangible return on investment (ROI) over time, outweighing their upfront expenses.
Traditional Demand Forecasting: Traditional demand forecasting methods may appear cost-effective in the short term due to their lower initial implementation costs. However, over time, the cumulative costs associated with manual labor, data processing inefficiencies, and suboptimal forecasts can add up, potentially exceeding the total cost of ownership (TCO) of AI-powered alternatives.
8. Customization and Flexibility
AI Powered Demand Forecasting: AI-driven demand forecasting solutions offer unparalleled customization and flexibility, empowering organizations to tailor forecasting models and methodologies to their specific business needs and industry requirements. These systems support a wide range of machine learning algorithms, optimization techniques, and model architectures, allowing forecasters to experiment with different approaches and adapt strategies on the fly.
Traditional Demand Forecasting: Traditional demand forecasting methods may offer limited customization and flexibility, often relying on standard statistical techniques and predefined forecasting models. While forecasters may have some latitude to adjust model parameters or select alternative methodologies, the scope for customization is typically constrained by the inherent limitations of traditional approaches.
Advantages Of AI In Demand Forecasting
In an era of fast industry change and impending uncertainty, businesses are rapidly recognizing the need for more sophisticated demand forecasting techniques. Though historically dependable, traditional approaches can lack flexibility and reactivity. AI and machine learning provide a solid alternative. Let’s take a look at the actual benefits of AI in demand forecasting:
1. Enhanced Precision
AI algorithms excel in analyzing extensive datasets and historical trends, resulting in more precise demand forecasts. This diminishes forecasting inaccuracies and enhances overall precision, providing businesses with a competitive edge in anticipating market needs with greater accuracy and reliability, leading to improved resource allocation and operational optimization.
2. Instantaneous Insights
AI empowers businesses with the capability to analyze real-time factors impacting demand, like market trends and social media activity. This furnishes companies with immediate insights, enabling prompt responses to market fluctuations, thereby allowing businesses to stay agile and responsive in dynamic market environments, ultimately driving better strategic decision-making and capitalizing on emerging opportunities swiftly.
3. Scalability Boost
AI systems possess the capacity to manage substantial data volumes, making them adaptable for businesses of various sizes and complexities. As data quantities increase, AI-driven forecasting models can adjust and sustain dependable predictions, ensuring scalability and resilience in meeting evolving business demands, facilitating growth and expansion without compromising forecasting accuracy or efficiency.
4. Tailored Adaptability
AI models can be personalized to accommodate specific industry intricacies, seasonal patterns, and other demand-affecting factors. This adaptability permits businesses to tailor forecasting approaches to their individual needs and navigate evolving market landscapes effectively, ensuring relevance and applicability in diverse business environments, thus enhancing adaptability and competitiveness in fluctuating market conditions.
5. Decision Time
AI-powered demand forecasting significantly cuts down decision-making lead times. By delivering swifter and more precise predictions, businesses can streamline supply chain management, curtail inventory imbalances, and enhance overall operational efficiency, leading to quicker and more informed decision-making processes, thereby improving agility and responsiveness in addressing market demands and opportunities.
6. Economical Gains
Through optimized inventory levels and minimized stock discrepancies, businesses can realize cost efficiencies. AI-based forecasting assists in synchronizing production and supply chain activities with actual demand, thereby reducing holding costs and averting lost sales opportunities, ultimately contributing to improved profitability and financial performance, enabling businesses to allocate resources more efficiently and invest in strategic initiatives for long-term growth.
7. Customer Satisfaction
Accurate demand forecasting ensures product availability aligns with customer preferences, fostering enhanced satisfaction. This allows businesses to better meet customer demands and circumvent instances of product unavailability, nurturing long-term customer loyalty and retention, thereby strengthening brand reputation and market competitiveness, and driving positive word-of-mouth referrals and repeat business.
8. Data-Driven Decisioning
AI harnesses data-driven insights to support decision-making processes, enabling businesses to make informed and strategic choices grounded in historical and real-time data analysis rather than intuition alone, thereby increasing the likelihood of successful outcomes and minimizing risks associated with subjective decision-making, fostering a culture of evidence-based decision-making and continuous improvement throughout the organization.
9. Risk Management
AI models are adept at pinpointing potential demand-related risks, aiding businesses in proactively addressing and mitigating these uncertainties. This encompasses responding to external influences like economic downturns, natural calamities, or shifts in consumer behavior, enabling businesses to anticipate and mitigate potential disruptions effectively, thereby safeguarding business continuity and minimizing financial losses, enhancing resilience and adaptability in volatile market conditions.
10. Continuous Evolution
AI systems continuously refine their forecasting models through ongoing learning from new data, ensuring relevance and efficacy amidst evolving market conditions, thereby providing businesses with a sustainable competitive advantage through adaptive and responsive forecasting capabilities, facilitating continuous improvement and innovation, and enabling businesses to stay ahead of market trends and maintain leadership positions in their respective industries.
Use Cases Of AI In Demand Forecasting For Different Industries
AI-powered demand forecasting revolutionizes industries by accurately predicting consumer needs. From retail to healthcare, its applications optimize inventory management, resource allocation, and production planning, enhancing efficiency and profitability. The use cases of AI in demand forecasting for different industries are as follows:
I. Retail
AI demand forecasting in retail helps optimize inventory management, reduce stockouts, and improve customer satisfaction by predicting consumer preferences and behaviors.
1. Historical Sales Data Analysis
Utilizing AI to scrutinize historical sales data incorporates considerations such as seasonality, holidays, and promotions to discern patterns and trends. This analysis aids retailers in comprehending past consumer behavior, facilitating more informed predictions for future demand.
2. Predictive Analytics for Inventory Management
AI algorithms analyze real-time data from diverse sources like point-of-sale systems, online transactions, and social media to predict future demand accurately. This enables retailers to optimize inventory levels, preventing stockouts or overstock situations.
3. Monitoring Market Trends and External Factors
AI continuously monitors and analyzes external factors such as economic indicators, weather patterns, and social trends. By integrating these insights into forecasting models, retailers can adapt predictions based on factors influencing consumer behavior.
4. Customer Segmentation Analysis
AI examines customer data to segment the market by preferences, buying behavior, and demographics. Understanding distinct needs of different segments enables retailers to tailor inventory and marketing strategies to meet specific demand patterns.
5. Dynamic Pricing Optimization
AI-powered pricing algorithms adjust prices dynamically based on real-time demand fluctuations, competitor pricing, and other market variables. This allows retailers to maximize revenue and promptly respond to changes in consumer behavior.
6. Promotion Planning and Optimization
AI aids in planning and optimizing promotional activities by predicting the impact of promotions on demand. Retailers can identify effective promotional strategies and allocate resources to drive sales without overcommitting inventory.
7. Supply Chain Optimization
AI enhances demand forecasting by integrating with supply chain systems. Considering factors such as lead times, transportation constraints, and supplier reliability, retailers can ensure a synchronized supply chain aligning with predicted demand patterns.
II. Supply Chain and Logistics
AI enables accurate demand forecasting, enhancing supply chain efficiency through optimized inventory levels, transportation planning, and distribution strategies, reducing costs and improving delivery reliability.
1. Transportation Network Optimization Through Demand Forecasting
A logistics company seeks to enhance its transportation network efficiency by anticipating demand variations across different routes and transport modes. Utilizing demand forecasting models powered by AI, the company can analyze historical shipment data, customer orders, and market trends to predict future transportation needs accurately. This empowers the company to optimize routes, allocate resources effectively, and ensure timely deliveries.
2. Maximizing Warehouse Space Efficiency
Warehouse management aims to optimize space utilization by predicting changes in product demand. Leveraging AI-driven demand forecasting models, warehouse managers can analyze SKU-level data, order history, and market trends to anticipate which products will experience heightened demand. Armed with this information, they can adjust storage configurations, allocate space efficiently, and enhance overall inventory management strategies.
3. Enhancing Supplier Collaboration and Production Planning
An electronics manufacturer, reliant on multiple suppliers for components, endeavors to synchronize production with fluctuating demand for finished goods. AI tools analyze historical order data, production lead times, and supplier performance metrics to accurately forecast component demand. This facilitates collaboration with suppliers, streamlines production planning processes, and mitigates risks of shortages or excess inventory throughout the supply chain.
4. Optimized Inventory Replenishment Through Demand Forecasting
A retail chain operating across multiple locations aims to replenish inventory at each store based on local demand dynamics. AI algorithms leverage sales data, historical inventory levels, and external factors such as local events or promotions to forecast demand at individual stores. This enables the implementation of tailored inventory replenishment strategies, minimizing stockouts, and reducing excess inventory carrying costs effectively.
5. Efficient Management of Reverse Logistics
Companies endeavor to efficiently manage returns by accurately forecasting the volume of products to be returned. AI-powered analytics models scrutinize historical return data, customer behavior, and product attributes to predict future returns. This insight aids in optimizing reverse logistics processes, reducing processing times, and managing returned inventory more effectively.
6. Streamlining Cross-Border Logistics Through Demand Forecasting
International logistics providers seek to optimize customs clearance procedures and shipping routes by anticipating fluctuations in demand for cross-border shipments. AI algorithms analyze global trade data, regulatory changes, and historical shipping patterns to forecast demand for specific international routes accurately. This enables logistics providers to streamline customs clearance processes, allocate resources efficiently, and enhance overall cross-border logistics efficiency.
III. Manufacturing
AI demand forecasting optimizes production schedules, resource allocation, and inventory management, ensuring efficient operations, reducing waste, and meeting customer demands effectively.
1. Customer Behavior Analysis Utilization
Through the utilization of customer behavior analysis, AI harnesses data from various channels such as online platforms and customer service interactions. Predictive models meticulously identify patterns and trends in customer preferences, providing manufacturers with invaluable insights. Equipped with this knowledge, manufacturers can strategically adjust production volumes and refine product offerings, ensuring a proactive response to anticipated changes in customer demand and fostering a more agile and customer-centric manufacturing approach.
2. Management of Seasonal Demand Fluctuations
Leveraging AI models that meticulously analyze historical sales data across different seasons, predictive analytics becomes a potent tool for manufacturers to precisely forecast seasonal demand fluctuations. Armed with these insights, manufacturers can strategically optimize their production schedules, fine-tune inventory levels, and efficiently manage staffing resources. This proactive approach enables businesses to adeptly meet peak demand during specific seasons, ensuring operational efficiency and customer satisfaction.
3. Implementation of Dynamic Pricing Strategies
AI plays a pivotal role in the manufacturing sector by seamlessly integrating market conditions, competitor pricing, and historical sales data. Through predictive models, it intelligently recommends optimal pricing strategies aligned with precise demand forecasts. This dynamic pricing approach empowers manufacturers to adapt swiftly, ensuring they can maximize revenue by aligning prices with current market dynamics and efficiently meeting customer demand.
4. Forecasting Demand for Customized Products
Leveraging data on customized product orders and customer preferences, AI employs predictive models to accurately forecast demand for specific configurations and customizations in the manufacturing sector. By harnessing this insight, manufacturers gain the ability to optimize production processes, ensuring they can efficiently meet the dynamic demand for personalized products. This tailored approach not only enhances customer satisfaction but also streamlines production resources, contributing to a more agile and responsive manufacturing ecosystem.
IV. Finance and Banking
AI-powered demand forecasting in finance and banking aids in predicting market trends, optimizing investment strategies, managing risk, and improving decision-making processes for better financial performance.
1. AI-Powered Portfolio Management
AI plays a pivotal role in investment portfolio management by meticulously analyzing historical market data, economic indicators, and sentiments expressed in news sources. Utilizing this wealth of information, predictive models crafted by AI technologies forecast the potential performance of diverse securities and investment instruments. Armed with these insights, investment managers are empowered to proactively optimize and rebalance portfolios, strategically navigating anticipated market shifts.
2. Customer-Centric Product Preferences Analysis
Banks leverage artificial intelligence to meticulously scrutinize customer transaction histories, behavior patterns, and interactions with a spectrum of banking products. Through predictive modeling, these institutions anticipate the demand for specific financial products, ranging from loans and credit cards to savings accounts. Armed with these insights, banks adeptly tailor their marketing strategies and product offerings, aligning them with individual customer preferences.
3. AI-Enhanced ATM and Branch Optimization
Banks harness the power of AI to analyze historical transaction data and foot traffic patterns at ATMs and branches, enhancing operational efficiency. Through predictive models, these institutions accurately forecast peak usage times and strategic locations for their ATMs and branches. Armed with this foresight, banks can proactively optimize resource allocation, ensuring sufficient staffing and availability of cash resources during high-demand periods. This intelligent approach to resource management allows banks to enhance customer service, minimize wait times, and streamline operations based on real-time demand insights.
4. Personalized Banking Services Through AI
Banks delve into the intricacies of individual customer data, encompassing spending habits, financial aspirations, and significant life events, with the power of AI. Utilizing sophisticated predictive models, these institutions foresee and understand personalized banking service preferences. This foresight empowers banks to deliver tailor-made services, ranging from bespoke financial planning and investment advice to personalized loan options, aligning seamlessly with the unique needs and objectives of each customer.
V. Automotive
AI-driven demand forecasting assists automotive companies in predicting consumer demand for vehicles and parts, optimizing production schedules, managing inventory, and adapting to market fluctuations efficiently.
1. Predictive Maintenance Revolutionizes Automotive Operations
AI algorithms harness historical vehicle maintenance data to forecast component failures and replacement cycles. This proactive approach enables automakers to schedule replacement part production efficiently, reducing downtime and enhancing operational efficiency. Ultimately, this predictive maintenance strategy ensures timely component availability, fostering improved customer satisfaction and cost-effective maintenance protocols.
2. Streamlining Supply Chains Through AI Integration
AI plays a pivotal role in demand forecasting within the automotive industry, analyzing factors like raw material availability and production bottlenecks. By anticipating potential disruptions, AI systems assist automakers in optimizing their supply chain, aligning production capabilities with forecasted demand accurately. This integration enhances operational efficiency by ensuring a seamless match between production and market needs.
3. Insightful Market Analysis with AI Tools
AI tools leverage social media, online reviews, and customer feedback to discern emerging market trends and consumer preferences. By extracting insights from diverse data sources, automakers gain a nuanced understanding of current and future market demands. This knowledge enables dynamic adjustments to production plans, ensuring product alignment with consumer expectations and enhancing competitiveness.
4. Personalized Production with AI Predictive Capabilities
AI’s ability to analyze individual customer preferences enables automakers to predict demand for specific vehicle configurations and features. This predictive capability facilitates customized production processes, catering to the demand for personalized vehicles. Consequently, automakers can offer a diverse range of products that meet customers’ specific desires, potentially gaining a competitive advantage.
5. Optimizing Dealer Inventories Through AI Insights
AI optimizes dealer inventories by analyzing sales data, regional demand patterns, and seasonal variations. By providing actionable insights into high-demand vehicle models and configurations, AI empowers automakers and dealers to manage inventory effectively. This proactive approach minimizes excess inventory, ensuring the alignment of supply with localized demand dynamics.
VI. Healthcare
AI demand forecasting in healthcare assists in predicting patient admission rates, medication needs, and resource allocation, optimizing healthcare services, reducing operational costs, and improving patient care and satisfaction.
1. Medication and Vaccine Demand Forecasting
Utilizing historical prescription data, disease prevalence, and vaccination trends, AI employs predictive models to anticipate the demand for specific medications and vaccines. This foresight equips healthcare providers to optimize their inventory, production processes, and distribution channels effectively, ensuring timely and sufficient availability of essential pharmaceuticals to meet anticipated demand. This application of AI streamlines healthcare operations, enhancing responsiveness to the dynamic healthcare landscape.
2. Hospital Bed and Staffing Optimization
By analyzing historical patient admissions, seasonal trends, and patterns of disease outbreaks, AI forecasts the demand for hospital beds and staffing needs. This foresight enables hospitals to proactively reallocate resources, ensuring optimal facilities and healthcare personnel availability during periods of heightened demand. This AI-driven approach enhances the efficiency of healthcare resource management, ultimately improving patient care and response capabilities during peak demand scenarios.
3. Medical Equipment and Supplies Forecasting
Through scrutiny of usage patterns, maintenance records, and external influences on medical equipment, AI conducts predictive modeling to anticipate demand for diverse medical devices and supplies. This enables healthcare facilities to streamline procurement and storage processes, mitigating the risk of shortages or excess inventory. The result is a more cost-effective and efficient management of resources in healthcare settings.
4. Personalized Medicine Demand Forecasting
AI facilitates personalized medicine demand forecasting by analyzing genetic data, patient records, and treatment outcomes. Through predictive models, the technology anticipates the demand for individualized therapies and targeted treatments. This empowers healthcare providers to tailor treatment plans according to expected patient needs and advancements in personalized medicine, ensuring a more precise and patient-centric approach to medical care.
How To Implement AI In Demand Forecasting?
From establishing clear objectives to continual improvement strategies, each phase plays a vital role in harnessing AI’s potential for accurate and efficient demand prediction. Let’s explore the key elements that constitute a successful integration of AI into demand forecasting.
1. Establishing Objectives and Scope
Begin by thoroughly articulating the objectives and scope of integrating AI into demand forecasting. This involves delineating specific areas, products, or services where AI will be applied and establishing clear, measurable goals and key performance indicators (KPIs) to gauge the effectiveness of the implementation.
2. Data Gathering and Preparation
Initiate the process by meticulously gathering historical data pertaining to relevant variables, encompassing sales figures, customer behavioral patterns, prevailing market trends, and external influences. Subsequently, focus on meticulously cleaning and preprocessing the amassed data to rectify inaccuracies and inconsistencies, addressing issues such as missing values and outliers.
3. Selecting Appropriate AI Algorithms
Deliberate over the selection of suitable AI algorithms tailored to the nuances of the demand forecasting challenge at hand. This may entail considering various options, including but not limited to linear regression, decision trees, neural networks, and time series models like ARIMA or LSTM, based on their applicability and efficacy.
4. Conducting Feature Engineering
Undertake a thorough exploration to identify and formulate pertinent features that can augment the predictive capabilities of the AI models. This could involve the transformation or amalgamation of existing variables to furnish more insightful inputs conducive to accurate forecasting.
5. Model Training Process
Segment the historical data into distinct training and validation sets to facilitate the training of the AI model. Proceed to conduct rigorous training using the designated training set, followed by an assessment of its performance on the validation set. Fine-tune hyperparameters such as learning rates or hidden layer dimensions to optimize the model’s predictive accuracy.
6. Integration with IT Infrastructure
Ensure seamless integration of the AI model with the existing IT infrastructure, necessitating close collaboration between data scientists and IT specialists. This may encompass deploying the model on organizational servers or cloud platforms in a manner that aligns with operational requirements.
7. Real-time Data Integration Mechanisms
Implement robust mechanisms to facilitate the seamless incorporation of real-time data streams into the AI model. This could entail establishing robust data pipelines or leveraging APIs to ensure that the model remains updated with the latest information essential for demand forecasting.
8. Validation and Testing Procedures
Validate the accuracy and efficacy of the AI model by subjecting it to rigorous testing using historical data not utilized during the training phase. Evaluate its performance under diverse scenarios to ascertain its resilience and reliability in real-world conditions.
9. Continual Improvement Strategies
Embrace a culture of continuous improvement by actively monitoring the performance of the AI model and soliciting feedback from stakeholders. Utilize this feedback to iteratively enhance the model’s capabilities over time, fostering its continual evolution and refinement.
How Does AI Affect Demand Forecasting?
AI, particularly machine learning, enhances demand forecasting via dimensionality, scalability, and feedback loops. It doesn’t aim for AGI but leverages advanced algorithms for speed and scale, transforming traditional processes effectively. Explore how AI influence demand forecasting
1. Enriching Prediction Depth
Demand forecasting traditionally relies on past sales data, but AI and machine learning models, especially those leveraging deep learning neural networks, offer a unique advantage in handling a wide range of variables or “features.” This dimensionality allows AI models to consider unconventional data types, such as textual data processed through techniques like word embeddings, significantly enhancing their understanding and analysis capabilities beyond traditional methods.
2. Scalability
In today’s digital landscape, data is generated at an unprecedented rate, necessitating scalable solutions for demand forecasting. AI-powered systems efficiently process vast volumes of data, spanning terabytes or even petabytes, thanks to advancements in cloud computing and data collection. This scalability enables AI to provide insights and efficiency that traditional methods cannot match, ensuring businesses can effectively handle the immense amount of data generated in modern markets.
3. Feedback Loop
Enhanced forecasting accuracy relies on a feedback loop where forecast models are regularly adjusted based on actual sales and customer behaviors. AI accelerates this process by autonomously processing vast data streams, identifying trends and patterns that may elude human analysts. This AI-driven feedback loop enables businesses to develop agile demand forecasting systems, constantly adapting to market shifts and improving accuracy over time.
4. Adaptive Models
Unlike static forecasting models, AI systems can adapt and self-improve over time. Through techniques like reinforcement learning, these models can adjust their predictions based on feedback from actual sales data, leading to continuous optimization and better forecasting accuracy.
5. Personalized Forecasting
AI algorithms can incorporate individual customer preferences and behavior data to create personalized demand forecasts. By understanding each customer’s unique purchasing patterns, businesses can tailor their inventory management strategies and marketing efforts more effectively.
Cost Affecting Factors To Consider To Implement AI In Demand Forecasting
Implementing AI in demand forecasting involves various factors that can affect costs. Here are few factors to consider
1. Data Quality and Availability
The quality and availability of data are paramount for accurate demand forecasting. Poor data quality or limited availability may require additional resources for data cleaning, integration, or augmentation, thus increasing costs.
2. Model Complexity and Development
The complexity of AI models used for demand forecasting, such as deep learning models or advanced machine learning algorithms, can significantly impact costs. Developing and fine-tuning complex models often require specialized skills and computational resources, which can increase expenses.
3. Infrastructure and Computing Resources
AI models for demand forecasting often require substantial computing resources, especially for training large-scale models or handling big data. Costs associated with cloud computing services, hardware procurement, or maintaining in-house infrastructure should be considered.
4. Training and Expertise
Building and maintaining AI capabilities within the organization necessitates investments in training and expertise. Costs may include hiring data scientists, AI specialists, or investing in employee training programs to ensure the effective implementation and utilization of AI for demand forecasting.
5. Integration with Existing Systems
Integrating AI-driven demand forecasting systems with existing IT infrastructure, including enterprise resource planning (ERP) systems or customer relationship management (CRM) platforms, can incur costs. Compatibility issues, customization requirements, and integration with legacy systems may require additional resources and investments.
6. Maintenance and Updates
Continuous monitoring, maintenance, and updates of AI models and systems are essential to ensure their effectiveness and relevance over time. Costs associated with regular maintenance, model retraining, software updates, and adapting to changing business requirements should be factored into the overall cost of implementing AI in demand forecasting.
Conclusion
The convergence of AI and demand forecasting marks the onset of a revolutionary era across industries worldwide. Positioned at technological advancement, AI’s capabilities in pattern recognition, adaptability to market shifts, and provision of unparalleled insights are poised to redefine forecasting fundamentally.
Future developments are anticipated to introduce more sophisticated AI algorithms, deeper integration of real-time data, and an emphasis on predictive analytics that not only respond to but also influence market dynamics. For businesses, keeping pace with these evolving AI trends in demand forecasting isn’t just about gaining a competitive edge—it’s essential for future success. Embracing AI is not only strategic, but also necessary for organizations seeking to lead in the changing market scenario.
Looking To Make a AI Project ? We Can Help
Idea Usher’s expertise empowers businesses to make informed decisions, mitigate risks, and capitalize on opportunities, driving sustained growth and competitive advantage in today’s dynamic market landscape.
Contact us today to know more about how we can help with our AI & ML development services.
Hire ex-FANG developers, with combined 50000+ coding hours experience
FAQ
Q. What are the key benefits of adopting AI for demand forecasting?
A. AI-driven demand forecasting offers several advantages such as improved accuracy, enhanced efficiency through automation, better inventory management leading to reduced stockouts or excess inventory, and the ability to respond quickly to changing market dynamics.
Q. How scalable is AI-driven demand forecasting for businesses of different sizes?
A .AI-driven demand forecasting solutions can be scalable and adaptable to businesses of various sizes. Whether a small startup or a large corporation, customizable AI tools and platforms are available to suit specific needs and budgets.
Q. How does AI improve demand forecasting accuracy?
A. AI utilizes advanced algorithms and machine learning techniques to analyze vast amounts of historical data, market trends, and external factors. By detecting patterns and correlations that humans may overlook, AI enhances the accuracy of demand forecasts, leading to more informed decision-making.
Q. What data is required for AI-powered demand forecasting?
A. AI algorithms rely on diverse datasets, including historical sales data, market trends, economic indicators, weather patterns, and even social media sentiment analysis. By incorporating a wide range of relevant data sources, AI can generate more comprehensive and accurate forecasts.
Q. How does AI handle demand forecasting for new products or markets?
A. AI excels at handling demand forecasting for new products or markets by leveraging techniques like probabilistic modeling and similarity analysis. By comparing the characteristics of new products or markets to existing data, AI can provide valuable insights into potential demand patterns and market behavior.
Q. What are the benefits of integrating AI into demand forecasting processes?
A. Integrating AI into demand forecasting processes offers several benefits, including improved forecast accuracy, reduced forecasting errors, optimized inventory management, enhanced customer satisfaction through better product availability, and ultimately, increased profitability.
Q. Is AI in demand forecasting scalable for businesses of all sizes?
Yes, AI-powered demand forecasting is scalable and adaptable for businesses of all sizes. Whether you’re a small startup or a large enterprise, AI solutions can be tailored to meet your specific needs and budget constraints. Additionally, many AI platforms offer scalable cloud-based solutions, making them accessible and cost-effective for businesses at any scale.