Businesses across various sectors, including finance, healthcare, cybersecurity, and supply chain management, are encountering increasingly complex risks. AI-powered risk mitigation can help address issues like financial fraud, data breaches, regulatory non-compliance, and operational disruptions, which pose significant threats, often resulting in financial losses and reputational damage.
So many enterprises are shifting towards Artificial intelligence, which transforms risk prediction and mitigation through real-time threat detection, predictive analytics, and automated decision-making. Unlike traditional systems, AI solutions learn from vast datasets, enhancing accuracy and adaptability to emerging risks. Given the increasing complexity of threats, businesses must adopt AI-powered risk tools to improve security, ensure compliance, and maintain resilience.
In this blog, we will talk about the step-by-step process of implementing AI for risk mitigation, potential cost, and affecting factors. Also, the key use cases of AI-powered risk mitigation and the future trends of this implementation.
The Growing Market for AI in Risk Management
According to Grand View Research, the global AI trust, risk, and security management market was valued at $1.96 billion in 2023 and is expected to grow at a CAGR of 21.3% from 2024 to 2030.
Adoption Trends Across Industries
- Finance: AI-powered fraud detection, algorithmic trading, and credit risk analysis are becoming industry standards.
- Healthcare: Predictive analytics in patient diagnostics and automated risk assessments enhance patient safety.
- Supply Chain: AI-driven demand forecasting and real-time risk monitoring help businesses respond to disruptions effectively.
Why Businesses Are Moving Away from Rule-Based Systems?
Traditional rule-based risk management systems rely on predefined conditions and static algorithms. However, these systems struggle to adapt to evolving threats, making them less effective in dynamic environments. In contrast, AI-driven risk mitigation solutions offer several advantages:
- Continuously learning from data to identify emerging risks.
- Reducing false positives by analyzing complex patterns and contextual information.
- Providing real-time risk assessment, enabling faster and more informed decision-making.
As AI-driven risk management solutions demonstrate greater accuracy and adaptability, businesses are increasingly adopting these technologies to enhance operational security and long-term resilience.
What Is AI-Powered Risk Mitigation?
AI-powered risk mitigation refers to the use of artificial intelligence to identify, assess, and reduce risks across industries. Unlike traditional risk management systems, AI-driven solutions continuously learn from data, detect hidden patterns, and make automated decisions to minimize threats.
Types of AI Used in Risk Mitigation
AI utilizes advanced algorithms to detect, assess, and mitigate risks across various domains, ensuring proactive threat management.
Machine Learning
- Supervised Learning: Utilizes labeled risk data to train models for fraud detection, credit scoring, and cybersecurity threat identification.
- Unsupervised Learning: Identifies anomalies in large datasets, helping detect fraudulent transactions, system breaches, or operational risks.
- Reinforcement Learning: Continuously improves risk responses by learning from past actions and adapting to emerging threats.
Natural Language Processing
- Analyzes contracts and regulatory documents to ensure compliance by scanning legal and policy documents for discrepancies, regulatory violations, and non-compliant clauses, reducing legal risks.
- Monitors communications for signs of security breaches or policy violations by analyzing emails, messages, and other communications to detect suspicious activities, insider threats, or potential breaches, ensuring organizational security.
Computer Vision
- Enhances workplace safety by analyzing video feeds to detect hazardous conditions, ensuring timely interventions and reducing the risk of accidents.
- Monitors infrastructure integrity in industries such as construction and energy by identifying structural weaknesses, equipment failures, or maintenance needs, preventing potential hazards.
How Does AI Improve Risk Mitigation?
AI enhances risk mitigation by leveraging advanced algorithms to detect, predict, and respond to threats with greater speed and accuracy.
- Real-Time Data Processing: AI analyzes vast amounts of structured and unstructured data instantly, identifying risks before they escalate.
- Predictive Risk Modeling vs. Reactive Approaches: Unlike traditional systems that react to risks after they occur, AI predicts potential threats and prevents them proactively.
- Automated Decision-Making: AI-powered systems detect and block fraudulent transactions, flag suspicious activity, and trigger security protocols without human intervention.
- Reduced False Positives and Negatives: AI enhances accuracy by distinguishing genuine threats from false alarms, ensuring a more effective risk management approach.
Use Cases of AI in Risk Mitigation
These are some real-life cases of how AI can handle these traditional risks and empower businesses to stay ahead of risks. By integrating AI into risk management, companies can reduce financial losses, enhance decision-making, and ensure long-term sustainability.
1. Fraud Detection and Prevention in Banking
The use of AI-powered risk mitigation has become crucial for banks dealing with fraud. By utilizing AI-powered fraud detection systems, financial organizations can scrutinize and evaluate every transaction with great accuracy. Imagine a situation where AI algorithms identify unusual spending patterns on a customer’s credit card, such as a sudden surge in spending or a purchase made in an unexpected location.
The fraud detection system identifies this as a potential fraudulent activity and promptly notifies the security team, allowing them to verify the transaction’s authenticity and safeguard the customer’s assets. The strength of AI is found in its capacity to learn from every interaction, consistently refining its algorithms to detect new patterns of fraud.
As a result, banks are able to predict and prevent scams, which lowers the rate of fraud and builds customer trust and financial security. This forward-thinking approach has greatly changed the way financial risk is managed.
2. Anti-Money Laundering (AML) Compliance
Money laundering remains a significant challenge for financial institutions, with criminals constantly evolving their tactics. AI-powered AML systems analyze vast transaction datasets to detect suspicious financial activities that might go unnoticed by traditional methods.
For example, an AI system monitoring a bank’s transactions identifies an unusual pattern where multiple small deposits are made, followed by a large withdrawal. Recognizing this as a potential sign of money laundering, the AI system immediately notifies compliance officers for further investigation.
By implementing AI-driven AML solutions, banks and financial institutions can enhance regulatory compliance, prevent financial crimes, and avoid hefty fines associated with non-compliance.
3. Credit Scoring and Assessment for Loan Disbursements
Traditional credit scoring models often rely on outdated financial data, leading to inaccurate risk assessments. AI has revolutionized this process by analyzing alternative data points such as transaction behavior, employment history, and even online activities. Imagine a lender evaluating a loan application for an entrepreneur with limited credit history.
Instead of solely relying on past credit scores, AI examines spending habits, income consistency, and financial commitments to determine creditworthiness. If AI detects stable income flows and responsible spending, it assigns a favorable credit score, allowing the entrepreneur to secure funding.
By adopting AI-driven credit scoring, financial institutions can expand their lending reach while minimizing default risks. This ensures that creditworthy applicants receive fair assessments, improving financial inclusion and profitability for lenders.
4. Risk Management in Insurance
AI-driven risk assessment models enable insurers to evaluate policyholder risks with greater accuracy. By analyzing vast amounts of historical data, AI can identify risk factors that traditional methods might overlook, leading to more precise underwriting decisions.
Consider an insurance company using AI to analyze customer health data. If AI predicts a high-risk profile based on medical history and lifestyle habits, it adjusts policy pricing accordingly. This ensures that pricing reflects an individual’s actual risk level rather than relying solely on general demographic factors.
By incorporating AI, insurance firms can personalize premiums, reduce fraudulent claims, and optimize risk management. This not only improves financial stability for insurers but also enhances customer satisfaction by offering fair and transparent pricing.
5. Market Risk Analysis
Investment firms and financial institutions constantly face market risks due to economic fluctuations, political instability, and global crises. AI-driven market risk analysis helps businesses stay ahead by analyzing historical trends, economic indicators, and real-time market data.
Imagine an investment firm monitoring stock market trends. AI algorithms detect an impending downturn based on factors such as rising inflation and declining consumer spending. The system recommends portfolio adjustments to minimize potential losses, allowing investors to shift funds to more stable assets.
By leveraging AI for market risk analysis, businesses can make informed investment decisions, protect their assets, and adapt to economic changes with agility.
6. Algorithmic Trading
The financial markets operate at lightning speed, where even a fraction of a second can determine profit or loss. AI-powered algorithmic trading systems execute trades in real time by analyzing vast amounts of market data, news sentiment, and investor behavior.
Consider a hedge fund using AI for stock trading. The AI system detects a bullish trend in a tech company based on increased social media mentions and positive earnings reports. It instantly places a buy order, maximizing profit before human traders even react.
By leveraging AI-driven trading strategies, financial firms can execute high-frequency trades, minimize human error, and optimize investment portfolios for better returns.
7. Cybersecurity Threat Detection
Cyberattacks are becoming more sophisticated, posing significant risks to businesses worldwide. AI-driven cybersecurity systems provide proactive protection by continuously monitoring network activity and detecting anomalies.
Imagine a corporate IT security team receiving an AI alert about an unusual login attempt from an unrecognized location. The AI system instantly flags the activity and restricts access, preventing a potential data breach.
By integrating AI in cybersecurity, businesses can safeguard sensitive information, prevent financial losses, and maintain trust among customers and stakeholders.
8. Supply Chain Risk Prediction
Supply chain disruptions can cripple businesses, leading to production delays and financial losses. AI predicts supply chain risks by analyzing historical data, weather forecasts, and geopolitical events.
For example, an AI-driven system detects an approaching hurricane that could impact a key supplier. The system advises businesses to reroute shipments or source materials from alternative suppliers, preventing costly disruptions.
By leveraging AI for supply chain risk management, businesses can maintain operational efficiency and ensure seamless production cycles.
9. Food Safety Risk Prediction
Foodborne illnesses can damage a brand’s reputation and lead to severe financial penalties. AI-driven food safety risk prediction systems analyze environmental conditions, transportation delays, and contamination reports to ensure product safety.
Consider a food distributor using AI to monitor temperature-sensitive shipments. If AI detects a temperature deviation that could compromise food quality, it sends instant alerts, allowing corrective action before the products reach consumers.
By implementing AI in food safety, businesses can ensure regulatory compliance, prevent recalls, and build consumer trust.
10. Drug Safety
Pharmaceutical companies rely on AI to assess drug safety by analyzing clinical trial data and predicting potential side effects.
For example, AI detects an adverse reaction pattern among trial participants, prompting researchers to modify drug formulations before mass production.
By integrating AI-powered risk mitigation, pharmaceutical companies can accelerate drug development while ensuring patient safety and regulatory compliance.
11. Vehicle Diagnostics
AI-powered vehicle diagnostics help businesses in the automotive and transportation industries predict and prevent mechanical failures. By analyzing real-time data from sensors embedded in vehicles, AI can detect potential issues before they escalate into costly repairs or accidents.
For example, an AI-driven fleet management system monitors engine performance, brake conditions, and fuel efficiency across a logistics company’s trucks. If the system detects abnormal engine heat in one of the vehicles, it alerts the maintenance team to inspect and fix the issue before a breakdown occurs.
By implementing AI-driven vehicle diagnostics, businesses can reduce maintenance costs, enhance vehicle performance, and ensure fleet reliability. This not only improves operational efficiency but also increases safety for drivers and passengers.
12. Autonomous Vehicle Safety
As self-driving technology continues to evolve, AI plays a crucial role in ensuring the safety of autonomous vehicles. AI-powered systems process data from cameras, LiDAR, and radar sensors to detect obstacles, predict pedestrian movements, and make split-second driving decisions.
Imagine an autonomous car navigating a busy intersection. AI detects a pedestrian stepping onto the road unexpectedly and immediately adjusts the vehicle’s speed to prevent a collision. In another scenario, AI identifies icy road conditions and modifies the vehicle’s braking system to maintain control.
By leveraging AI for autonomous vehicle safety, manufacturers and fleet operators can enhance accident prevention, improve passenger safety, and comply with regulatory standards. This technology is critical for building trust in self-driving vehicles and ensuring a safer future for transportation.
13. Customer Churn Prediction
Losing customers directly impacts revenue, but AI helps businesses identify at-risk customers before they leave.
For instance, an AI system analyzing customer behavior detects reduced engagement and missed payments, signaling a potential churn risk. The company responds with personalized retention strategies, such as discounts or improved customer service.
By using AI-driven churn prediction, businesses can improve customer retention and maximize long-term revenue.
14. Predictive Maintenance for Assets in Manufacturing
Manufacturers face significant financial losses when equipment fails unexpectedly. AI-powered predictive maintenance analyzes real-time sensor data from machinery to detect early signs of wear and tear.
Imagine a factory using AI-driven systems to monitor its production line. The system identifies irregular vibrations in a critical machine, signaling a potential failure. Instead of waiting for a breakdown, the maintenance team receives an alert to perform necessary repairs, preventing downtime and expensive emergency fixes.
By implementing AI-driven predictive maintenance, businesses can extend the lifespan of assets, reduce maintenance costs, and ensure uninterrupted production.
15. Natural Disaster Risk Assessment
AI helps businesses and governments assess the risk of natural disasters by analyzing weather patterns, historical data, and satellite imagery.
For instance, an AI model predicts an impending hurricane by detecting rapid temperature changes in the ocean. Governments and businesses in the affected region receive early warnings, allowing them to implement evacuation plans and secure infrastructure.
By leveraging AI for disaster risk assessment, companies can minimize financial losses, enhance preparedness, and protect both assets and human lives.
16. Portfolio Risk Optimization
Investment firms use AI to optimize portfolios by analyzing financial markets, economic trends, and investor sentiment in real time.
Consider a hedge fund managing a diversified portfolio. AI detects increased market volatility and recommends rebalancing investments by shifting assets to lower-risk sectors. This automated strategy helps mitigate potential losses while maintaining profitability.
By using AI for portfolio risk management, investors can make data-driven decisions, maximize returns, and minimize exposure to unpredictable market fluctuations.
17. Data Privacy
With increasing concerns over data breaches and regulatory compliance, AI-driven data privacy solutions help businesses safeguard sensitive information.
For example, an AI system in a healthcare organization automatically detects unauthorized access to patient records and blocks potential breaches before they occur. The system also ensures compliance with data protection regulations like GDPR and HIPAA.
By implementing AI-powered data privacy solutions, businesses can enhance cybersecurity, maintain regulatory compliance, and build customer trust.
18. Third-Party Vendor Risk Evaluation
Companies often rely on third-party vendors for services, but these partnerships come with risks such as financial instability, security vulnerabilities, and regulatory non-compliance. AI automates vendor risk assessment by analyzing historical performance, credit ratings, and cybersecurity practices.
Imagine an enterprise using AI to evaluate a potential IT outsourcing partner. The system flags concerns about the vendor’s history of data breaches, allowing decision-makers to reconsider the partnership before signing a contract.
By leveraging AI for third-party risk evaluation, businesses can ensure secure and reliable collaborations while mitigating operational risks.
19. Employee Misconduct Detection
AI helps organizations detect unethical behavior, fraud, and policy violations within the workplace by analyzing communication patterns, transactional data, and employee behavior.
For example, an AI system in a corporate setting notices an employee frequently accessing confidential files outside of regular working hours. The system flags this behavior as suspicious, prompting an internal investigation that prevents potential data theft.
By implementing AI-driven misconduct detection, companies can maintain ethical standards, prevent internal fraud, and protect their reputation.
20. Biometric Security
Traditional authentication methods like passwords are vulnerable to cyber threats. AI-powered biometric security enhances access control by using fingerprint recognition, facial scanning, and voice authentication.
Consider a financial institution using AI-driven facial recognition for secure account access. Customers can log in to their banking apps with a simple facial scan, eliminating password-related security risks.
By integrating AI-based biometric security, businesses can strengthen identity verification, prevent fraud, and enhance overall cybersecurity.
Step-by-Step Process to Develop and Implement AI for Risk Mitigation
Building an AI-powered risk mitigation system requires a structured approach, from defining objectives to continuously improving the model. Below is a detailed step-by-step breakdown.
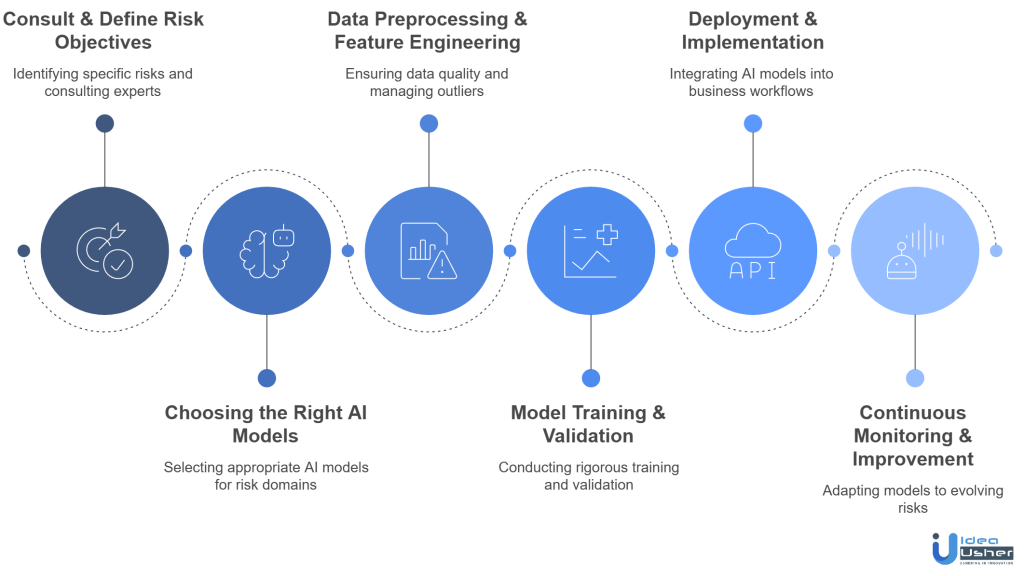
1. Consult & Define Risk Objectives
Before developing an AI model, it is crucial to define the specific risks it will mitigate, such as fraud, compliance violations, or cybersecurity threats. Consulting experts like IdeaUsher’s developers and market researchers helps refine risk objectives and design tailored AI solutions. Diverse data sources such as IoT sensors, CRM databases, financial logs, APIs, and security logs enhance accuracy, while technologies like Apache Kafka and AWS Glue streamline data processing for optimal performance.
2. Choosing the Right AI Models
The choice of AI models depends on the risk domain:
- Random Forest & Decision Trees: Used for financial fraud detection and credit risk scoring due to their ability to handle structured data efficiently.
- Neural Networks & Deep Learning: Effective for pattern recognition in cybersecurity and healthcare risk prediction, utilizing frameworks like TensorFlow and PyTorch.
- NLP Transformers (BERT, GPT): Applied in contract analysis, compliance monitoring, and regulatory risk assessment by leveraging language models like OpenAI’s GPT or Google’s BERT.
3. Data Preprocessing & Feature Engineering
Data quality directly impacts AI model performance. Managing missing data is crucial, as gaps can distort risk assessments. Techniques such as imputation (filling in missing values) or model-based estimation can help maintain data integrity. Outlier detection is another essential step, particularly in fraud detection and cybersecurity. AI models must identify anomalies without overfitting to unusual patterns. Techniques like Isolation Forest and Autoencoders are commonly used for this purpose.
4. Model Training & Validation
To ensure reliability, AI models must undergo rigorous training and validation:
- Cross-validation: Divides data into training and testing sets to improve generalization and prevent overfitting. Commonly used techniques include k-fold cross-validation and stratified sampling, implemented using Scikit-learn, TensorFlow, and PyTorch.
- AUC-ROC Metrics: Assesses the model’s ability to distinguish between high and low-risk scenarios. The ROC (Receiver Operating Characteristic) curve evaluates performance at various thresholds, and AUC (Area Under the Curve) indicates overall accuracy. A higher AUC denotes improved detection of real threats and fewer false alarms. This metric is calculated using Scikit-learn, TensorFlow, and XGBoost to evaluate accuracy in fraud detection, cybersecurity, and compliance monitoring.
5. Deployment & Implementation
AI models must be seamlessly integrated into business workflows to provide real-time risk mitigation.
- API-Based Deployment: AI models are deployed as REST APIs, allowing businesses to integrate risk assessment capabilities into their existing platforms.
- Cloud Deployment: Deploying in cloud servers such as AWS, Azure, GCP, etc. offers scalability and cost efficiency, making it ideal for businesses with dynamic risk mitigation needs.
- On-Premise Deployment: Preferred by industries with strict regulatory requirements, such as finance and healthcare, to ensure data security and compliance.
6. Continuous Monitoring & Improvement
AI models must adapt to evolving risks through continuous monitoring and updates.
- Retraining AI Models: Periodic model retraining with fresh data ensures that risk assessments remain accurate over time.
- Explainable AI (XAI): Enhances transparency by providing insights into AI-driven risk decisions, helping businesses comply with regulatory requirements and build trust.
Monitoring tools such as MLflow and AI Explainability 360 assist in tracking model performance and maintaining transparency in AI-driven risk mitigation systems.
Work with Ex-MAANG developers to build next-gen apps schedule your consultation now
Cost of Developing an AI for Risk Mitigation
Developing an AI system for risk mitigation requires careful planning and investment. The cost varies based on the complexity of the solution, the AI models used, and regulatory compliance requirements.
Estimated Cost Breakdown
App Complexity | Estimated Cost | Key Features Included |
MVP (Basic fraud detection) | $10,000 – $30,000 | Anomaly detection, basic machine learning models, real-time alerts, API integration |
Mid-tier (Supply chain + NLP) | $30,000 – $70,000 | Predictive analytics, NLP for compliance monitoring, automated reporting, cloud-based deployment |
Enterprise (Multi-risk AI) | $70,000 – $100,000 | Advanced AI models (deep learning, NLP transformers), multi-risk analysis, real-time decision automation, on-premise/cloud hybrid deployment, regulatory compliance tools |
Factors Influencing The Cost of AI-powered Risk Mitigation Development
Several factors impact the overall cost of developing an AI system for risk mitigation, including the scope of the solution, AI models used, data complexity, deployment model, and regulatory requirements.
1. Scope of the Solution
A basic anomaly detection system costs less than a multi-risk enterprise platform with AI-driven compliance, fraud detection, and security features. The more complex the solution, the more resources are needed for data processing, model training, and system integration, increasing overall costs.
2. AI Models Used
Pre-trained models (like OpenAI’s GPT or Google’s BERT) are more affordable. Custom AI models require more development effort, especially deep learning and NLP-based solutions, adding 20–30% to costs. The complexity of model architecture and the need for continuous updates further influence development expenses.
3. Data Complexity
Structured data (financial transactions, logs) is easier to process. Unstructured data (emails, reports, audio/video analytics) requires additional cleaning and feature engineering, increasing development expenses. The need for high-quality labeled data and extensive preprocessing efforts also contributes to overall costs.
4. Deployment Model
Cloud-based solutions (AWS, Azure, Google Cloud) are cost-efficient and scalable. On-premise deployments require additional infrastructure and maintenance costs, making them more expensive upfront. The choice between cloud, on-premise, or a hybrid model depends on business needs, affecting long-term operational costs.
5. Regulatory Compliance Needs
Industries handling sensitive data (healthcare, finance, insurance) must comply with HIPAA, GDPR, or PCI-DSS, leading to higher costs due to additional security and audit requirements. Ensuring regulatory adherence requires investment in encryption, audit logs, and compliance monitoring systems, adding to the overall budget.
Challenges and Solutions in AI-Powered Risk Mitigation
While AI-powered risk mitigation provides significant advantages, businesses must address key challenges to ensure trust, accuracy, and compliance.
1. Data Privacy and Regulatory Compliance
Challenge: AI systems often process sensitive data, making it essential to comply with regulations such as the General Data Protection Regulation (GDPR) in Europe and the Health Insurance Portability and Accountability Act (HIPAA) in the United States. Failure to meet these standards can result in legal repercussions and financial penalties.
Solution:
- Implement data encryption and anonymization to safeguard sensitive information.
- Follow privacy-by-design principles to ensure AI systems collect only the necessary data.
- Conduct regular compliance audits to align with evolving regulatory requirements.
2. Bias Mitigation in AI Models
Challenge: AI models may inherit biases from training data, leading to unfair or inaccurate risk assessments, particularly in areas such as credit scoring, fraud detection, and insurance underwriting.
Solution:
- Utilize diverse and representative datasets to improve model fairness.
- Apply bias detection and fairness testing tools, such as IBM’s AI Fairness 360, to identify and mitigate biases.
- Implement explainable AI (XAI) to ensure transparency and accountability in AI-driven risk assessments.
3. Handling False Positives in Risk Detection
Challenge: High false positive rates in fraud detection, cybersecurity alerts, and compliance monitoring can overwhelm teams, leading to inefficiencies and operational bottlenecks.
Solution:
- Use advanced anomaly detection techniques, such as unsupervised learning, to enhance accuracy.
- Incorporate reinforcement learning so that AI models can continuously refine their risk predictions based on new data.
- Implement human-in-the-loop (HITL) systems, allowing experts to review flagged risks before further action is taken.
Future Trends in AI-powered Risk Mitigation
As AI continues to evolve, new technologies are enhancing risk mitigation strategies. Here are the key trends shaping the future of AI-powered risk management:
1. Generative AI for Synthetic Risk Scenarios
Generative AI can simulate potential risks by creating synthetic fraud cases, cyberattack patterns, or financial anomalies. This helps businesses train AI models on rare but critical threats, improving risk preparedness without relying solely on historical data.
2. Federated Learning for Secure Data Sharing
Traditional AI models require centralized data storage, which can pose privacy risks. Federated learning allows multiple organizations (e.g., banks, healthcare providers) to train AI models without sharing raw data, enhancing security and compliance with regulations like GDPR.
3. Explainable AI (XAI) for Transparency
As AI-driven decisions become more complex, businesses need greater transparency. Explainable AI (XAI) is gaining traction, enabling organizations to understand why an AI system flagged a transaction as fraudulent or detected a compliance risk. This is crucial for regulatory approval and trust-building.
4. AI-Augmented Human Decision-Making
AI will shift from fully automated risk management to AI-assisted decision-making, where human experts review AI-generated insights before taking action. This hybrid approach improves accuracy and reduces risks associated with AI bias or over-reliance on automation.
5. AI-Driven Quantum Security
With the rise of quantum computing, traditional encryption methods may become obsolete. AI-powered quantum-resistant security models will help organizations protect sensitive data against quantum cyber threats, ensuring long-term resilience.
6. Real-Time Edge AI for Risk Detection
Edge AI enables real-time risk mitigation by processing data locally on devices rather than relying on cloud-based AI models. This is particularly useful for IoT-driven security systems, fraud prevention in mobile banking, and industrial risk monitoring.
Conclusion
AI is revolutionizing risk management by enabling businesses to predict, prevent, and respond to threats in real time. From fraud detection and cybersecurity to healthcare risk prediction and supply chain resilience, AI-powered solutions offer unparalleled accuracy, efficiency, and scalability.
However, risks are constantly evolving, and traditional rule-based systems are no longer sufficient. To stay ahead, businesses must embrace AI-driven risk mitigation strategies that adapt to emerging threats, ensure compliance, and enhance decision-making.
AI-driven risk mitigation systems leverage machine learning, NLP, and computer vision to refine models in real time, reducing false positives and improving predictions. As risks grow more complex, integrating AI is essential for long-term security and resilience.
Develop an AI-powered Risk Mitigation Solution with IdeaUsher!
Our team of expert engineers specializes in AI, machine learning, and enterprise security, developing advanced risk mitigation solutions tailored to various industries. IdeaUsher is a leader in AI-driven risk management, delivering real-time threat detection, predictive analytics, and automated compliance monitoring for enhanced security and operational resilience.
With experienced ex-MAANG/FAANG engineers, we integrate cutting-edge features such as AI-powered fraud detection, anomaly detection, behavioral risk analysis, and regulatory compliance automation. Our solutions ensure scalability, high-performance data processing, and seamless integration with enterprise security systems, financial platforms, and regulatory frameworks.
Partner with IdeaUsher to build a robust, AI-driven risk mitigation solution that safeguards your business, minimizes vulnerabilities, and ensures compliance in an evolving risk landscape.
Check out our portfolio of development solutions we gave to other reputed enterprises!
Work with Ex-MAANG developers to build next-gen apps schedule your consultation now
FAQs
Q.1. What is the use case of AI in risk management?
AI is widely used in risk management for fraud detection, cybersecurity threat prevention, regulatory compliance, supply chain risk assessment, and healthcare risk prediction. It helps organizations analyze vast datasets in real time, detect anomalies, and automate decision-making to prevent potential threats before they escalate.
Q.2. What are the four commonly used risk mitigation processes?
The four primary risk mitigation strategies include:
- Avoidance: Eliminating activities or processes that pose significant risks.
- Reduction: Implementing controls to minimize the likelihood or impact of risks.
- Sharing: Transferring risk through insurance, outsourcing, or partnerships.
- Acceptance: Acknowledging and preparing for risks that cannot be eliminated.
Q.3. How do you mitigate risks associated with AI?
Mitigating AI-related risks involves ensuring data privacy compliance (GDPR, HIPAA), reducing model bias, enhancing explainability (XAI), and continuously monitoring AI performance. Implementing ethical AI practices and robust validation techniques helps improve reliability and fairness in AI-driven decisions.
Q.4. How is AI used in operational risk management?
AI enhances operational risk management by automating risk assessments, predicting system failures, monitoring compliance, and analyzing workplace safety hazards. It enables businesses to detect inefficiencies, prevent disruptions, and ensure seamless operations through real-time analytics and predictive modeling.