In the ever-evolving digital landscape, threats can lurk around every byte. From fraudulent transactions to cyberattacks, staying ahead of these dangers is paramount. Here, Artificial Intelligence emerges as a powerful guardian, wielding the power of anomaly detection to identify real-time threats with unparalleled precision. This innovative technology empowers us to sift through massive datasets, uncovering hidden patterns and deviations that might signal imminent trouble.
In this blog, we’ll explore the different types of anomalies and how AI excels at finding them. We’ll also showcase real-world examples of AI-powered anomaly detection across various industries, highlighting its transformative impact on security, operational efficiency, and data-driven decision-making.
What are Anomalies?
In data analysis, anomalies are data points or events that deviate significantly from the established pattern within a dataset. They’re the unexpected variations – sudden spikes, dips, unusual values – that signal a departure from the predictable and often reveal hidden insights. Understanding and identifying anomalies is crucial in numerous fields, as they can expose risks, inefficiencies, or valuable new opportunities.
Let’s break down common types of anomalies:
Outliers (Point Outliers)
These are isolated data points that stand in stark contrast to the overall dataset. Outliers can result from errors during data entry, unexpected malfunctions, or even malicious activity. For instance, an extremely high-value financial transaction conducted outside of normal business hours might represent a potential instance of fraud.
Changes in Events
This type of anomaly involves a sudden, noticeable shift in a previously established pattern. A rapid change in sensor readings within a manufacturing process could indicate a developing equipment failure, requiring prompt intervention.
Drifts
Drifts are less dramatic, representing gradual, long-term variations within a dataset. They might signal emerging trends in market behavior, evolving customer preferences, or subtle changes in system performance. Tracking these subtle shifts helps organizations adapt and make proactive adjustments.
Types of Anomalies
Here’s a breakdown of the main types of anomalies and examples:
Global outliers
Global outliers represent extreme deviations from the expected pattern within a dataset, standing out conspicuously regardless of context. They often arise from measurement errors, data entry mistakes, unusual events, or even malicious activities. Examples include
- An industrial sensor reads a temperature far outside its normal operating range, potentially signaling equipment failure.
- A surge in network traffic exceeds typical usage by orders of magnitude, which could indicate a system overload or an attempted cyberattack.
Contextual outliers
The nature of Contextual outliers depends heavily on the surrounding circumstances or established norms. A data point considered typical in one situation might raise red flags in another. Examples include:
- A financial transaction for a large amount occurring outside of standard business hours or from an unusual geographic location, possibly indicating fraudulent activity.
- A significant variation in a patient’s vital signs that, while within the overall normal range, deviates notably from their baseline, potentially suggesting a change in health status.
Collective outliers
Collective outliers focus on identifying a group of related data points that, when analyzed collectively, reveal an abnormal pattern. Individually, these data points might appear insignificant, but their combined behavior exposes an underlying anomaly. Examples include:
- A series of seemingly small, unsuccessful login attempts targeting a user account originating from multiple IP addresses. This could suggest an orchestrated attempt to breach the account.
- In medical diagnostics, multiple correlated lab results subtly fall outside the normal range, which might collectively indicate a developing health condition.
- Within e-commerce, a trend of minor, seemingly unrelated product returns could signal an organized effort to defraud the company.
What is Anomaly Detection in AI?
Anomaly detection in AI is essentially finding the odd ones out in a data set. It uses machine learning algorithms to identify patterns and trends in historical data and then flags anything that deviates significantly from those patterns as an anomaly.
Here’s a breakdown of the concept:
- Training with Data: The AI model is trained on a large amount of existing data. This data could be things like sensor readings from machinery, website traffic patterns, or financial transactions.
- Learning Patterns: The model learns the typical patterns and trends within the data. This could be things like the average traffic flow on a website or the normal operating temperature of a machine.
- Identifying Abweichungen (German for Deviations): Once the model understands what “normal” looks like, it can start to identify data points that fall outside the expected range. These deviations are the anomalies.
There are two main approaches to anomaly detection in AI:
- Supervised Learning: This approach requires data to be labeled as either “normal” or “anomalous” beforehand. The model is then trained to identify similar patterns in new data.
- Unsupervised Learning: This approach doesn’t require pre-labeled data. The model analyzes the data itself to determine what’s normal and what’s not.
Key Advantages Of AI In Anomaly Detection
Here are some key advantages of AI in anomaly detection compared to traditional methods:
- Superior Pattern Recognition: AI algorithms can analyze vast amounts of data and identify complex patterns that might be missed by humans or simpler statistical methods. This allows for the detection of more subtle anomalies and even entirely new types of anomalies that haven’t been encountered before.
- Automated and Continuous Monitoring: AI systems can monitor data streams 24/7 without interruption, making them ideal for real-time anomaly detection. This is crucial in scenarios where a quick response is necessary, such as fraud detection or cybersecurity threats.
- Adaptability and Learning: AI models can continuously learn and improve over time. As they are exposed to new data, they can adjust their understanding of “normal” behavior and become more adept at identifying anomalies that might deviate from evolving patterns.
- Scalability: AI systems can handle massive datasets efficiently, making them suitable for applications that generate a high volume of data, such as sensor networks or financial transactions.
- Reduced False Positives: Traditional methods might generate a high number of false positives, which are alerts triggered by normal data fluctuations. AI can learn to distinguish between true anomalies and normal variations, leading to a more focused and actionable set of alerts.
- Uncovering Hidden Insights: Anomaly detection with AI isn’t just about identifying problems. By analyzing the characteristics of anomalies, AI can help us understand underlying causes and relationships within the data. This can lead to new insights and improvements in various processes.
Why is Anomaly Detection Important?
anomaly detection serves as a critical tool for safeguarding systems, optimizing processes, and uncovering hidden opportunities. Here’s why it’s so important across diverse industries:
Cybersecurity Defense
Perhaps its most critical role is in cybersecurity. Spotting anomalies in network activity, user behavior, or system logs can be the difference between thwarting a cyberattack and suffering a devastating data breach. By proactively identifying unusual patterns, anomaly detection strengthens an organization’s defensive posture.
Data Management
Anomalies often signal errors, inconsistencies, or emerging data quality issues. Integrating anomaly detection within data pipelines helps ensure the integrity and reliability of information. This is crucial, as flawed data can lead to poor decision-making and lost opportunities.
Proactive Response
The ability to detect anomalies in real time translates directly into proactive intervention. Whether it’s preventing a catastrophic equipment failure, addressing a security incident, or capitalizing on an emerging market trend, timely detection allows organizations to respond swiftly and effectively.
Adaptability and Insight
Advanced anomaly detection systems, especially those powered by machine learning, continuously learn from the data they analyze. This adaptability translates to both protective and opportunistic benefits. Organizations gain resilience against new threats, uncover hidden patterns in customer behavior, and drive innovation as they spot new market possibilities.
Wide Applications
Anomaly detection reaches far beyond IT and cybersecurity. Let’s consider a few examples:
- Financial Fraud: Identifying unusual transactions, spending patterns, or account activity helps banks and institutions protect customers and themselves from financial losses.
- Healthcare: Anomalies in patient vital signs or lab results can indicate developing conditions, allowing for timely diagnosis and treatment.
- Manufacturing: Tracking deviations in equipment readings or product quality ensures consistency and helps pinpoint potential defects before they reach customers.
Why do you need Machine Learning for Anomaly Detection?
Traditional anomaly detection approaches often falter when faced with the realities of modern data. Machine learning offers a powerful arsenal of tools to tackle these challenges and unlock the full potential of anomaly detection:
Managing Big Data
Machine learning algorithms thrive when analyzing massive, high-dimensional datasets that would cripple rule-based systems and overwhelm human analysts. They tirelessly sift through the vastness of information to pinpoint meaningful anomalies.
Tackling Unstructured Data
Traditional techniques often struggled with data lacking clear structure – images, network logs, sensor readings, text, etc. ML, particularly deep learning, excels at extracting patterns from this complex data, uncovering anomalies that would otherwise remain hidden.
Real-Time Analysis
Legacy anomaly detection often focuses on historical data, leaving a window of vulnerability. ML powers systems that analyze data streams in real time as events happen. This is transformative in fields like cybersecurity, where rapid response to anomalies defines the difference between a minor incident and a catastrophic breach.
Enhanced Security and Reliability
By detecting anomalies across network traffic, user behavior, and system logs, ML bolsters cybersecurity defenses. Anomaly detection also plays a critical role in industries like manufacturing and healthcare. Detecting equipment malfunctions at the earliest stage through ML minimizes costly downtime and safety risks, ultimately improving operational reliability.
Adaptability and Uncovering Hidden Insights
The best ML-powered systems continuously learn and evolve. They adapt to new types of threats, adjust to changing data patterns, and can even uncover unexpected trends in normal data that reveal new customer segments for businesses or potential efficiencies in operations.
Leveraging Machine Learning Techniques
ML offers diverse techniques that surpass simple statistical baselines:
- Classification models learn to discern complex differences between normal and abnormal data points.
- Clustering algorithms group similar data, allowing anomalies to stand out as isolated points or unusual clusters.
- Deep learning models identify intricate, non-linear patterns within high-dimensional datasets.
What are the different Anomaly Detection Methods?
There are two main categories of anomaly detection methods in AI, depending on how the training data is labeled:
1. Supervised Anomaly Detection:
- Requires labeled data: This means the training data needs to be pre-classified as either “normal” or “anomalous.”
- Advantages:
- High accuracy for known anomaly types because the model is specifically trained to identify those patterns.
- Can provide a confidence score for anomaly detection.
- Disadvantages:
- Reliant on the quality and comprehensiveness of labeled data. New or unforeseen anomalies might be missed.
- Labeling data can be expensive and time-consuming.
Here are some common supervised anomaly detection methods:
* **Support Vector Machines (SVMs):** These algorithms can create a hyperplane that separates normal data points from anomalies in a high-dimensional space.
* **K-Nearest Neighbors (KNN):** This method classifies a data point as anomalous if it has few neighbors (similar data points) within a specific distance.
* **Decision Trees:** These tree-like models learn a series of rules to classify data points as normal or anomalous based on specific features.
2. Unsupervised Anomaly Detection:
- No labeled data required: The model analyzes the data itself to identify patterns and define what’s normal.
- Advantages:
- Can identify novel anomalies, even those not encountered in the training data.
- Less reliant on manual data labeling.
- Disadvantages:
- May struggle with complex or subtle anomalies, especially in high-dimensional data.
- Can generate more false positives compared to supervised methods.
Here are some common unsupervised anomaly detection methods:
* **Clustering Algorithms:** These methods group data points into clusters based on similarity. Points that fall outside established clusters can be flagged as anomalies. (e.g., K-means clustering)
* **Statistical Methods:** Techniques like outlier detection (e.g., Isolation Forest) analyze data distributions and identify points that deviate significantly from the statistical norm.
* **Neural Networks:** Autoencoders, a specific type of neural network, can be trained to reconstruct "normal" data. Data points that the autoencoder has difficulty reconstructing might be considered anomalies.
Choosing the right anomaly detection method depends on the specific application and the nature of the data. If you have well-defined anomalies you want to detect and labeled data is available, supervised methods might be a good choice. For situations where labeling is difficult or you want to identify unforeseen anomalies, unsupervised methods can be valuable.
Machine Learning Algorithms for Anomaly Detection
Machine learning offers a diverse arsenal of algorithms for anomaly detection. Let’s delve into some of the most powerful techniques:
Supervised Learning Algorithms
Decision Trees
They construct tree-like structures where branches represent decision rules based on data features. Anomalies are identified as data points sorted into classes with atypical characteristics or those that fail to fall neatly within established classifications.
Support Vector Machines (SVMs)
These find optimal hyperplanes in multi-dimensional feature spaces. These hyperplanes separate data belonging to different classes, thus isolating normal and anomalous instances. SVMs are known for handling high dimensionality and performing well even when data contains some noise.
Neural Networks
These highly flexible models are inspired by biological neural systems. With layered architectures and non-linear activation functions, they excel at capturing incredibly complex relationships between input features. This makes them effective in detecting subtle anomalies that simpler models often miss.
Unsupervised Learning Algorithms
K-Means
It partitions data points into a specified number of clusters based on similarity. Anomalies might appear as isolated outliers, belong to very small clusters that are sparsely populated or reside far away from well-formed cluster centers.
DBSCAN (Density-Based Spatial Clustering)
DBSCAN defines clusters as contiguous regions with a high concentration of data points. Anomalies frequently manifest in low-density regions or as points residing at the fringes of otherwise coherent clusters. DBSCAN is particularly robust in terms of data noise.
One-Class SVM
It is a specialized SVM variant that aims to learn the boundaries of what constitutes ‘normal’ data, as opposed to learning to separate multiple classes. Points falling significantly outside this learned ‘normal’ boundary are flagged as potential anomalies.
Isolation Forest
Isolation Forest constructs numerous decision trees, each randomly partitioning the dataset. Anomalies, due to their unique characteristics, are typically isolated with fewer partitions compared to ‘normal’ points. Their shorter ‘paths’ within the trees are used to identify them.
Deep Learning for Anomaly Detection
Autoencoders
Autoencoders are neural network architectures designed to compress input data into a compact representation and then reconstruct the original data as closely as possible. Trained on ‘normal’ data, they produce high reconstruction errors when faced with anomalies, making them especially powerful for complex data like images.
Recurrent Neural Networks (RNNs)
RNNs and their advanced variants, like LSTMs, are designed to process sequences. This makes them adept at analyzing time series data like equipment readings or network traffic. They learn expected patterns over time and can signal anomalies when those patterns are violated.
Generative Adversarial Networks (GANs)
GANs employ two competing neural networks. A ‘generator’ learns to produce data mimicking the training dataset. At the same time, a ‘discriminator’ tries to distinguish between real and generated data. Anomalies deviate significantly from what the GAN generates as ‘normal.’
Real World Use cases of AI in Anomaly Detection
Let’s delve into some real-world examples:
Cybersecurity Intrusion Detection
AI analyzes vast amounts of network traffic, user behavior data, and system logs to identify subtle deviations from established norms. This enables the detection of stealthy attacks that might evade traditional rule-based systems and reduction of alert fatigue for security analysts by pinpointing the most critical anomalies.
In 2020, cybersecurity firm Darktrace used AI to detect a novel attack on a European casino. Unusual internal network activity patterns alerted their AI system, preventing the attackers from reaching sensitive customer data and potentially stealing millions.
Fraud Detection and Operations Optimization
AI-powered contract management learns complex patterns from historical data to spot fraudulent transactions, insurance claims, or financial activity. Additionally, it can pinpoint operational bottlenecks and inefficiencies. This helps reduce financial losses by proactively identifying and preventing fraud and streamlining processes by highlighting areas that are ripe for optimization, improving operational efficiency.
PayPal, a major online payment platform, employs advanced AI models to analyze transaction data. Their systems can detect fraudulent patterns in real-time, even in seemingly legitimate transactions, helping prevent fraudulent credit card activity and chargebacks.
Health Monitoring and Fraud Prevention in Healthcare
AI in healthcare detects anomalies in patient data (vitals, lab results) that could signal developing conditions, allowing for early intervention. Also identifies unusual patterns in billing or claims that suggest fraud. This improves patient outcomes by facilitating faster diagnosis and treatment. It also Saves costs by reducing healthcare fraud and abuse, making the system more efficient.
AI is used to analyze medical imaging scans (x-rays, CTs, MRIs). Systems can detect subtle anomalies indicative of early-stage tumors or other diseases that might be missed by human radiologists, enabling timely diagnosis and improving treatment outcomes.
Defect Detection in Manufacturing
Computer vision systems powered by deep learning analyze images or videos of products, recognizing minute flaws, scratches, or misalignments with exceptionally high accuracy. This improves product quality, ensuring customers receive flawless products, reduces waste, and minimizes costly product recalls.
ArcelorMittal, one of the world’s largest steel producers, employs AI-based image analysis to detect defects in steel sheets and coils during production. This has led to significant waste reduction and improved product consistency.
Application Performance Management
AI establishes baselines of typical performance within complex IT systems, then spots anomalies in logs, metrics, and traces to pinpoint issues like bottlenecks, bugs, or misconfigurations. This minimizes IT system downtime, ensuring smooth operation and a seamless user experience. It also enables proactive problem resolution before they escalate into major outages.
Cloud platforms like Microsoft Azure and AWS incorporate AI-driven anomaly detection for their infrastructure monitoring. This allows them to proactively address issues that could degrade service quality for their customers.
Ensuring Product Quality
Beyond manufacturing, AI analyzes product data, quality reports, or customer feedback to identify subtle anomalies indicating quality degradation or deviations from standards. This protects brand reputation as AI helps in maintaining consistently high product quality and reduces costs associated with reworking, returns, or warranty claims.
Consumer electronics brands employ AI to analyze product feedback, warranty information, and customer support data. This helps them pinpoint subtle quality issues across product lines, enabling early corrective action to address potential defects.
Enhancing User Experience
AI analyzes user behavior patterns within apps or websites, identifying anomalies in navigation paths, error rates, or session lengths that reveal points of friction or issues hindering a seamless experience. It boosts user satisfaction and improves engagement with the digital product or service. It helps businesses quickly pinpoint and fix UX problems impacting conversion rates.
Major social media platforms like Facebook and Instagram utilize AI to analyze user behavior patterns. This helps them identify anomalies in how users interact with content and navigate the site or unusual spikes in error rates. These insights enable quick resolution of UX issues.
Identifying Inefficient Equipment in Manufacturing
AI analyzes sensor data from machinery, detecting unusual readings in vibration, temperature, or operating parameters that often precede equipment failures. This enables AI-powered predictive maintenance, preventing unplanned downtime and costly repairs. It also maximizes equipment lifespan and overall manufacturing efficiency.
Companies like Boeing and Airbus use AI to analyze data from aircraft engines and components. This helps predict potential failures, optimize maintenance schedules, and maximize aircraft operating time.
Risk Mitigation in IT and Telecom
AI monitors IT infrastructure, identifying anomalies in capacity, traffic patterns, or security events that could indicate impending failures, cyber-attacks, or vulnerabilities. Proactive risk mitigation reduces the financial impact of potential outages. It improves the overall reliability of IT and telecom systems, enhancing business continuity.
Telecom companies analyze network traffic patterns using AI. This helps them identify anomalies that could signal equipment issues, overloads that might lead to outages, or even cyberattacks, ensuring their network’s reliability.
Conclusion
AI-powered anomaly detection isn’t a futuristic concept – it’s a necessity in today’s data-driven world. By understanding the different types of anomalies, the techniques AI employs, and their real-world impact, businesses stand better equipped to harness this powerful technology. The ability to spot the unusual doesn’t just prevent problems; it can drive innovation.
How Can Idea Usher Help with AI-Powered Solutions For Businesses?
Unlock the power of AI without the complexity. Every business is unique. Why settle for a generic AI solution? Idea Usher understands your industry challenges. Let us build a customized AI solution to safeguard your specific operations and assets. Schedule a consultation today!
Hire ex-FANG developers, with combined 50000+ coding hours experience
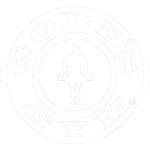
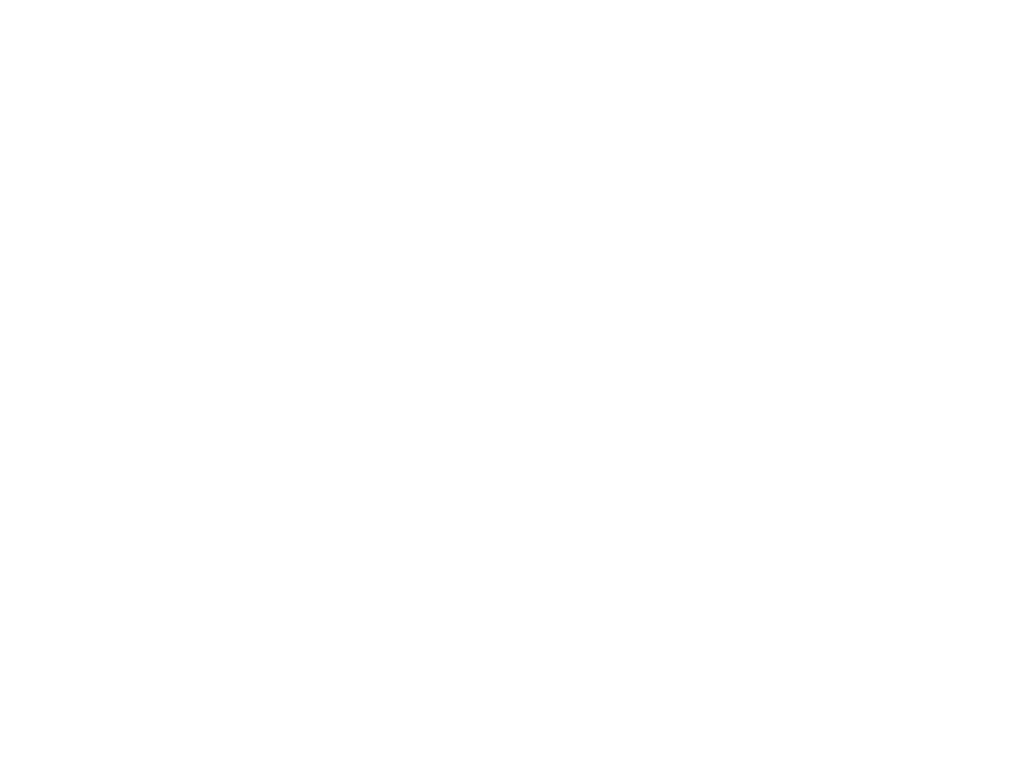
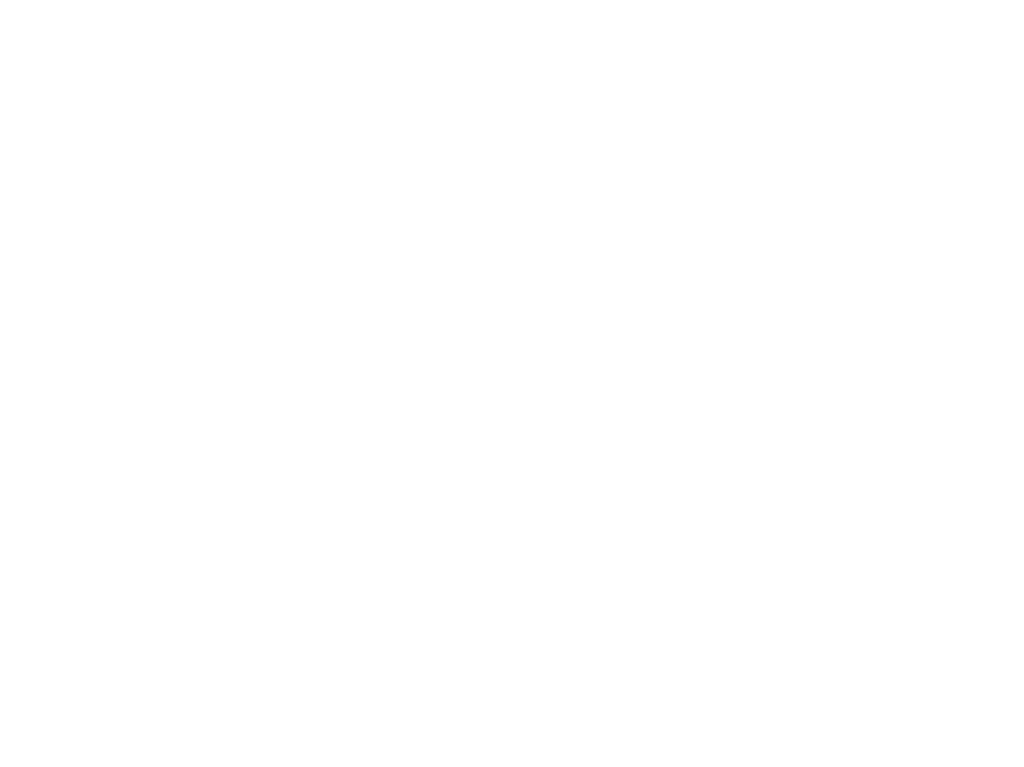
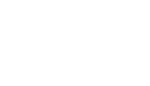
FAQs
How is AI used in anomaly detection?
AI revolutionizes anomaly detection by surpassing the limits of traditional rule-based or simple statistical approaches. It analyzes massive datasets, uncovering hidden patterns and relationships that hint at unexpected deviations. AI’s adaptability is a key advantage – models can learn from new data, refining their understanding of normality and spotting previously unknown anomalies.
Can we use generative AI in anomaly detection?
Yes! Generative AI, like GANs, can learn to model “normal” data with high fidelity. When presented with real-world data, anomalies become strikingly apparent as they deviate from the AI’s generated ideal. This approach is particularly useful when labeled examples of anomalies are scarce.
Which algorithm is best for anomaly detection?
Unfortunately, there’s no single “best” algorithm for anomaly detection. The optimal choice depends on several factors. The type of data you’re working with – it is numerical, images, text, or network logs – will largely influence algorithm selection.
Which type of AI is commonly used for detecting anomalies in network traffic?
Network anomaly detection relies on a mix of both supervised and unsupervised machine learning techniques. Supervised algorithms excel at pinpointing known types of attacks when trained on labeled examples of malicious traffic. Unsupervised methods are indispensable for detecting novel attack patterns or unusual traffic behaviors that deviate from established norms, where labeled data might be scarce.