Imagine significantly reducing the time it takes to bring life-saving medications to patients. The once-arduous journey of drug discovery is undergoing a transformative shift. Artificial intelligence (AI) is surfacing as a powerful weapon in this battle against life-threatening diseases.
This article provides a roadmap for tech developers interested in understanding the technical aspects of utilizing AI for drug discovery. It also provides a comprehensive understanding of how AI is transforming the landscape of drug discovery and its potential to bring about significant advancements in healthcare.
What AI in Drug Discovery
For decades, the search for new drugs has been a painstaking process, relying heavily on trial-and-error methods. This slow and expensive approach often left patients waiting for breakthroughs. However, the tide is turning with the emergence of artificial intelligence (AI) in drug discovery.
AI is transforming the way we discover new medications by acting as a powerful toolset. Here’s how AI is accelerating the hunt for life-saving treatments:
- Turbocharged Screening: Imagine sifting through millions of potential drug candidates in a blink! AI algorithms can analyze vast chemical libraries, identifying promising molecules with remarkable speed and efficiency. This replaces the traditional, time-consuming process of manually testing countless options.
- Precision Targeting: AI isn’t just fast, it’s also precise. By analyzing complex biological data like protein structures and disease pathways, AI can design drugs that target specific mechanisms of disease. This targeted approach can lead to more effective treatments with fewer side effects, which is a win-win for patients.
- Predictive Power: Developing drugs is a risky business, with many promising candidates failing in the later stages of clinical trials. AI can help mitigate this risk by predicting how a potential drug might interact with the body. This allows researchers to identify potential problems early on, saving valuable time and resources.
- Repurposing Existing Drugs: AI isn’t limited to creating new drugs from scratch. It can also analyze existing medications and identify new uses for them. This “drug repurposing” approach offers a faster path to treatment for new diseases or unmet medical needs.
Market overview of AI in Drug Discovery
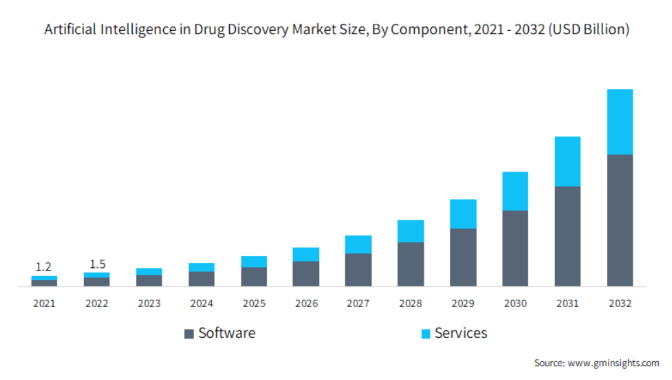
A report by Global Market Insights says that the global market size of AI in drug discovery stood at USD 1.5 billion in 2022. This market is expected to experience remarkable growth in the upcoming years, with a Compound Annual Growth Rate (CAGR) of 30.1% projected between 2023 and 2032.
Navigating the Drug Discovery Landscape
The traditional drug discovery journey takes over a decade, requiring the combined expertise of various scientific disciplines and incurring billions of dollars in costs. Each step presents a hurdle with a high potential for setbacks.
Target Identification
The initial quest involves identifying a biological target directly implicated in the disease process. This traditionally involves a combination of laborious lab tests, intricate cell-based studies, and even animal models.
Lead Discovery
Discovering a molecule that effectively interacts with the target traditionally involves screening vast libraries of compounds, a process notorious for its time-consuming nature.
Lead Optimization
After identifying a promising candidate, extensive optimization is required to ensure its potency, selectivity, and efficient absorption within the body. This typically involves medicinal chemistry, computer modeling, and further laboratory testing.
Preclinical Testing
In this process, the candidate drug is evaluated for efficacy and safety in animal models. This stage can be lengthy and expensive, requiring careful monitoring and analysis.
Clinical Trials
Clinical trials represent the most critical and resource-intensive phase. The potential treatment undergoes rigorous human testing to confirm its safety and effectiveness.
How AI Innovates Drug Delivery: Use Cases
Artificial intelligence (AI) is making remarkable waves in the realm of drug delivery, transforming the way medications reach patients and optimizing treatment effectiveness. Here are some prominent use cases that showcase the innovative applications of AI in this domain:
From Data Deluge to Discovery
Identifying potential drug targets traditionally involved sifting through a vast ocean of data – omics datasets, disease associations, research grants, and more. AI excels at navigating this information deluge. By leveraging its expansive knowledge base, AI can pinpoint novel proteins and genes that hold promise for therapeutic intervention.
Precision Through Simulation
The reliance on solely physical testing for candidate drug compounds is becoming a relic of the past. AI facilitates high-fidelity molecular simulations, essentially conducting virtual experiments on computers. This not only streamlines the process by eliminating the need for expensive laboratory procedures but also expedites the identification of promising candidates.
Prophetic Chemistry
Predicting the future behavior of a drug candidate is no longer a matter of chance. AI-powered systems can now forecast key properties like a drug’s toxicity, bioactivity, and physical characteristics. This crucial information gleaned from AI analysis translates to significant savings in time and resources during the development phase.
Molecule Innovation
The landscape of drug discovery is further reshaped by AI’s ability to design entirely new drug molecules. These pioneering systems move beyond conventional methods of screening pre-existing libraries and instead embark on a path of “de novo” drug design, essentially creating novel therapeutic agents from scratch. This opens doors to entirely new avenues for developing life-saving treatments.
Prioritizing the Most Promising
With a multitude of potential drug leads identified, AI steps in to meticulously prioritize the most efficacious candidates. This intelligent sorting mechanism surpasses traditional methods, further evaluating the most effective options, thus optimizing the drug development pipeline.
From Design to Production
AI’s capabilities extend to suggesting efficient synthesis pathways for producing these novel drug molecules. Furthermore, AI can even recommend modifications to the design to facilitate easier manufacturing, expediting the process of bringing new medications to market.
Engineering AI-driven Solutions for Drug Discovery
By harnessing the expertise of engineers, data scientists, and researchers, AI-powered solutions are poised to revolutionize the drug discovery landscape. This section delves into the engineering aspects of crafting AI-driven solutions that are revolutionizing this crucial field.
Data Management: Balancing Complexity and Volume
Imagine trying to drink from a bucket. That’s akin to the massive datasets AI craves for training. Genomic sequences, protein structures, and mountains of medical records offer a wealth of information, but managing and processing this data efficiently is crucial. Without proper strategies, the data itself can become a roadblock.
AI-powered data wrangling tools automate data collection, cleaning, and organization. These tools can sift through massive datasets, identify inconsistencies, and ensure the data is high-quality fuel for the AI engine. Techniques like natural language processing (NLP) can efficiently extract valuable insights from scientific literature, while machine learning algorithms can clean and organize the data, ensuring its accuracy and completeness.
Ensuring Model Accuracy: Refining the Predictive Power of AI
The success of AI hinges on accuracy – a single misstep can lead down a fruitless path. Ensuring the model’s predictions are reliable and generalizable to real-world situations is crucial. Refining the algorithms and continuously validating them with real data is essential to prevent AI from becoming a guessing game in the quest for new drugs.
Machine learning algorithms excel at pattern recognition within complex datasets. Researchers can leverage these algorithms to train AI models to analyze vast molecular libraries, identify promising drug candidates, and predict their potential effectiveness. This notably reduces the time and resources traditionally required for this initial phase of drug discovery.
Regulatory Considerations: Navigating the Compliance Landscape
Regulatory bodies are still grappling with the complexities of AI. Clear guidelines for developing AI models, ensuring data privacy, and understanding how these models arrive at their conclusions are still being formulated. Navigating this uncharted regulatory territory presents a significant hurdle for researchers who want to translate their AI-powered discoveries into tangible treatments.
AI-powered validation tools can be used to analyze the model’s performance on real-world data and identify any potential biases or limitations. This rigorous testing helps researchers refine the models and ensure their predictions are reliable, paving the way for successful clinical trials and, ultimately, the development of life-saving treatments.
AI Success Stories in Drug Discovery
While still in its early stages, Artificial Intelligence (AI) is demonstrably making waves in the realm of drug discovery.
Here are some notable success stories that illustrate its growing impact:
Unearthing Hidden Targets
In 2020, BenevolentAI leveraged its AI platform to discover a novel potential target for ALS, a breakthrough that bypassed years of conventional research. This exemplifies AI’s ability to sift through mountains of data and pinpoint promising avenues for therapeutic development.
AI-Powered Molecule Design
Exscientia, a frontrunner in AI-driven drug discovery, collaborated with Roche in 2021 to design molecules targeting a specific protein linked to cancer. This project achieved success in preclinical stages within a remarkably short timeframe, showcasing the potential of AI to significantly accelerate drug design.
Repurposing Existing Drugs
In 2014, IBM’s Watson played a pivotal role in identifying an existing anti-malarial drug as a potential treatment for Chagas disease. This demonstrates AI’s ability to unlock hidden therapeutic applications within established medications, saving valuable time and resources.
Optimizing Clinical Trials
Companies like Freenome are utilizing AI to analyze patient data and refine clinical trial design. Their AI platform identifies patients most likely to respond positively to specific cancer treatments, leading to more efficient and targeted trials.
AI Tools Revolutionizing Drug Discovery
AI algorithms are continuously acting as powerful tools, sifting through mountains of scientific data to identify promising drug candidates with unparalleled efficiency. Additionally, AI is continually delving into the intricate language of molecules, utilizing deep learning techniques to unveil potential targets for therapeutic intervention. This approach is paving the way for the development of drugs with even greater efficacy and targeted mechanisms of action.
Machine Learning
Imagine a high-powered sieve meticulously sorting through massive scientific data. ML algorithms excel at this, identifying promising drug candidates by recognizing hidden patterns within the data. This goes beyond simple searches, allowing researchers to analyze complex relationships between molecules, biological pathways, and disease mechanisms. This opens doors to exploring a broader range of potential drug targets and advancements.
Deep Learning
Think of DL as a master codebreaker in the world of drug design. By analyzing vast libraries of molecular data, these algorithms can decipher the intricate language of molecules. This newfound knowledge unlocks the potential to design drugs with targeted mechanisms of action, essentially striking directly at the root cause of diseases. This paves the way for more effective and specific treatments.
Natural Language Processing
Imagine having a tireless research assistant who can quickly process mountains of scientific literature. NLP acts as this assistant, rapidly extracting valuable insights like potential drug targets, existing research findings, and historical clinical trial data. This not only accelerates research but also helps uncover hidden connections that traditional literature reviews might miss.
These AI tools, working in concert, offer a powerful and versatile arsenal for researchers. Machine learning facilitates the identification of promising drug candidates, deep learning assists in the design of targeted therapies, and NLP expedites knowledge discovery from the scientific literature.
The Indispensable Features of AI in Drug Development
The traditional landscape of drug discovery is undergoing an upwheel with the integration of Artificial Intelligence (AI). AI’s unique capabilities propel it to the forefront, offering indispensable features that are transforming this crucial field. Here’s a closer look at the key aspects that make AI irreplaceable:
Data Powerhouse
Big Data Absorption: Drug discovery involves massive datasets – genomic information, protein structures, clinical trial data, and more. Efficiently processing and analyzing this information is crucial. AI must be equipped to handle this data deluge seamlessly.
Data Unification: Fragmentation across various databases and research silos hinders effective analysis. AI should seamlessly integrate data from internal databases, public repositories, and scientific publications, creating a unified knowledge base for informed decision-making.
Advanced Data Mining Techniques: AI should be an expert detective, wielding sophisticated algorithms to unearth hidden patterns and connections within complex datasets. This empowers researchers to identify promising drug targets and potential drug candidates with laser focus.
Drug Discovery Expertise
Target Sharpshooter: AI should be a master at identifying novel drug targets. Analyzing vast datasets should pinpoint molecules with high therapeutic potential and predict how potential drugs might interact with these targets.
Virtual Screening Champion: Imagine a high-throughput screening process conducted entirely in silico (through computer simulations). AI should excel at virtually screening libraries of potential drug molecules, assessing their binding affinity to target proteins, and prioritizing the most efficacious candidates for further development.
Predictive Powerhouse: Accurately predicting a drug’s efficacy, potential side effects, and pharmacokinetic behavior, such as absorption, distribution, metabolism, and excretion, is critical. AI’s predictive modeling capabilities enable early assessment of risks and facilitate the selection of safer and more effective drug candidates.
Beyond the Basics
User-Centered Design: Not everyone is a data scientist. An intuitive design and user-friendly interface are crucial for researchers with varying levels of technical expertise to utilize the AI platform and unlock its potential effectively.
Transparency and Explainability: The “black box” approach doesn’t work in drug discovery. AI systems need to be transparent, allowing researchers to understand the rationale behind the AI’s predictions. This fosters trust in the system and enables researchers to refine their approach based on the AI’s insights.
Scalability and Adaptability: The scientific landscape is constantly evolving. AI systems must be adaptable and capable of incorporating new data and scientific advancements and addressing emerging challenges in drug discovery. Additionally, the ability to scale computational resources efficiently is essential for handling increasingly complex datasets.
Unlocking Business Opportunities in the USA AI Drug Discovery Market
The US AI drug discovery market is poised for significant growth, driven by the immense potential of AI to revolutionize the entire drug development process. Here’s a glimpse into the exciting business opportunities this market presents:
Pharma & Biotech Companies: The Need for Speed and Innovation
The ever-growing prevalence of chronic illnesses like cancer, Alzheimer’s, and diabetes necessitates the constant development of novel and effective treatments. Traditional drug discovery processes are often slow and resource-intensive.
AI steps in as a game-changer, offering a faster and more efficient approach. Pharmaceutical and biotech companies are avidly investing in research and development (R&D) efforts to add AI tools into their pipelines. This not only fuels market expansion but also holds the promise of addressing critical medical needs with swift solutions.
Contract Research Organizations (CROs):
Contract Research Organizations (CROs) recognize the immense potential of AI and strategically incorporate it into their service offerings. This expansion caters to the growing demand from pharmaceutical companies seeking expertise in AI-powered drug discovery.
By leveraging AI, CROs can streamline various characteristics of the drug development process, including target identification, virtual screening, and clinical trial optimization. This translates to significant advantages for pharmaceutical companies: faster turnaround times, reduced costs, and access to the latest advancements in AI technology.
Government Support and Technological Advancements
Governments around the world are increasingly acknowledging the transformative potential of AI in healthcare. This recognition is translating into crucial support through dedicated funding for research and development initiatives in this domain.
As a result, the field is witnessing continuous improvements in AI algorithms, machine learning techniques, and computational capabilities. These advancements increase the effectiveness and accessibility of AI tools in drug discovery, making them even more powerful and user-friendly. This fosters a fertile ground for market growth as the technology matures and wider adoption becomes a reality.
Top AI-powered Apps And Software Offering Drug Discovery in the USA
The landscape of drug discovery in the USA is significantly enhanced by a diverse array of software solutions. These tools address various aspects of the research pipeline, fostering efficient data management, analysis, and visualization:
Collaborative Drug Discovery (CDD Vault)
This cloud-based platform fosters secure collaboration throughout the drug discovery process. CDD Vault facilitates efficient data sharing, experiment tracking, and intellectual property management, promoting seamless communication and knowledge exchange within research teams.
SYNTHIA Retrosynthesis Software
SYNTHIA acts as an in-silico lab assistant. It analyzes target molecules and proposes optimal synthetic pathways for their production. Researchers can leverage this tool to identify the most cost-effective methods for synthesizing potential drug candidates.
ArgusLab
ArgusLab allows researchers to design, analyze, and simulate the interaction of potential drug molecules with biological targets. This visual representation provides crucial insights into a drug candidate’s potential efficacy by depicting how it might bind to specific proteins or receptors.
Genedata Screener
High-throughput screening (HTS) experiments are a cornerstone of drug discovery. Genedata Screener manages and analyzes the data generated from these large-scale experiments, facilitating the identification of promising drug candidates for further investigation.
OpenEye Scientific
OpenEye offers functionalities like molecule library design, virtual screening, and property prediction. Researchers use this to computationally assess the potential of various drug candidates before initiating resource-intensive laboratory experiments.
How Can AI-Powered Drug Discovery Apps and Software Generate Revenue?
Apps and software designed for drug discovery can employ various strategies to generate income and sustain their operations. Here’s a closer look at the prominent methods:
Licensing
Pharmaceutical companies, research institutions, and individual researchers pay licensing fees to access the software’s functionalities. These finances can be structured based on factors like the organization’s size, the specific features used, and the duration of access.
Subscription Services
Gaining traction is the Software-as-a-Service (SaaS) model. Users gain ongoing access to the software through monthly or annual subscription fees. This approach offers flexibility for users and ensures a recurring revenue stream for the software company.
Consulting and Support
Software companies often provide additional services beyond the core product. This can encompass implementation and training services to assist users in effectively utilizing the software. Data analysis and interpretation expertise can also be offered to make sense of the complex data generated. Additionally, customization services cater to the specific needs of a research group or project.
Freemium Model
This strategy involves offering a limited free version with basic functionalities. This enables potential users to test the software before committing to a paid subscription or license. Upgraded versions with more features are available for a fee.
Partnerships and Collaborations
Strategic partnerships with pharmaceutical companies or research institutions can be another revenue stream. This might involve co-developing new software solutions or offering custom services tailored to the specific needs of the partner.
Creating An AI-Powered Drug Delivery App/Software – Step-by-Step Explanation
Developing an AI-powered drug delivery app or software necessitates a meticulous approach that prioritizes user needs, leverages cutting-edge technology, and adheres to stringent regulations.
Here’s a comprehensive roadmap outlining the crucial stages involved:
Defining Objectives and Target Audience
Building a successful AI-powered drug delivery app hinges on defining its core purpose and target audience. The initial step necessitates meticulously outlining the app’s functionalities. This involves identifying specific challenges it aims to address in medication delivery.
By clearly defining the app’s objectives and target users, you ensure a focused solution that directly addresses a specific gap in medication management. This targeted approach guarantees the app delivers the most value to its intended audience.
Data Acquisition and Integration
Drug databases offer in-depth information on medications, and medical literature provides valuable insights into potential drug interactions and adverse effects. To seamlessly integrate this data, the app can leverage APIs or develop custom algorithms. This ensures efficient data processing and paves the way for AI algorithms to function effectively and generate meaningful insights for personalized drug delivery.
Tailoring AI Algorithms
At the core of the app’s functionality lies a synergistic coupling of machine learning and natural language processing (NLP) techniques. By harnessing the collective power of these cutting-edge AI techniques, the app transforms raw data into actionable insights.
Intuitive User Interface Design
Not everyone is a data scientist. An intuitive design and user-friendly interface are crucial for researchers with varying levels of technical expertise to utilize the AI platform and unlock its potential effectively.
Choosing the Development Platform and Programming Tools
Building the app’s infrastructure requires careful selection of the development platform and programming languages. Choosing a platform like iOS, Android, or web-based development depends on the target audience and desired reach.
To leverage the latest breakthroughs in AI and machine learning, consider utilizing frameworks specifically designed for these tasks, such as TensorFlow, PyTorch, or sci-kit-learn. Selecting a programming language like Python offers extensive support for data manipulation, model development, and smooth integration with other technologies.
Prioritizing Data Privacy and Security
Protecting sensitive patient data is paramount. The app must implement robust security measures to comply with regulations like HIPAA (US) or GDPR (EU). This includes employing encryption techniques to scramble data, access controls to restrict who can view information, and secure authentication mechanisms to verify user identities. These measures safeguard patient confidentiality, prevent unauthorized access, and mitigate the risk of data breaches.
Rigorous Testing and Validation
Ensuring the app functions flawlessly requires rigorous testing. Techniques like unit testing isolate and analyze individual parts, while integration testing verifies seamless interaction between components.
User acceptance testing brings in real users to assess the app’s usability and functionality from a practical standpoint. Additionally, feedback from healthcare professionals and domain experts helps refine the app based on real-world scenarios. This iterative testing process guarantees a robust and user-friendly application.
Ensuring Regulatory Compliance
Navigating the healthcare landscape necessitates strict adherence to regulatory requirements. Familiarize yourself with regulations set by governing bodies like the FDA (US) or EMA (EU) specific to medical software. Depending on the app’s classification and intended use, obtaining regulatory approvals or certifications might be mandatory. This process ensures the app meets stringent standards for safety, efficacy, and quality, fostering trust among users and healthcare professionals.
Deployment, Maintenance, and Updates
Following rigorous testing and validation, the app graduates to deployment on relevant platforms. This could be on app stores for wider user access or on specific healthcare platforms targeting medical professionals.
Post-launch, providing ongoing maintenance and support is crucial. Addressing any issues, bugs, or user feedback ensures a smooth user experience. Regular updates are important to add new features, improvements, and security patches. This ensures the app remains optimized, aligns with evolving regulations, and maintains compliance with industry standards.
Collaboration with Healthcare Professionals
Throughout development, fostering close collaboration with healthcare professionals, pharmacists, and patients is vital. Their input and feedback validate the app’s effectiveness and usability. Consulting with domain experts ensures the app aligns with clinical best practices and addresses the specific needs of its intended users.
Engaging healthcare providers in pilot studies or clinical trials allows for a data-driven evaluation of the app’s impact on patient outcomes, medication adherence, and the broader healthcare delivery system. This collaborative approach ensures the app is not only functional but also delivers tangible benefits to patients and the healthcare landscape.
Ethical Considerations in AI-powered Drug Discovery
AI-powered drug discovery offers immense promise, but ethical considerations necessitate careful navigation:
- Bias in Algorithmic Decisions: AI algorithms trained on biased datasets can perpetuate historical inequalities. This could lead to overlooking potential treatments for underrepresented demographics or prioritizing research on diseases with greater commercial appeal. To mitigate this, ensuring diverse and inclusive datasets is crucial. Additionally, implementing mechanisms to identify and rectify potential biases within the algorithms themselves is essential.
- Safeguarding Patient Data: Drug discovery often involves sensitive patient information. Rigorous data security protocols are paramount to prevent unauthorized access and data breaches. Furthermore, obtaining clear and informed patient consent regarding the usage of their data for AI-driven drug discovery is vital. Patients deserve to understand how their information will be utilized.
- Human Expertise Remains Paramount: While AI plays a significant role in expediting drug discovery, it shouldn’t supplant the irreplaceable role of human expertise. Scientific understanding, meticulously designed clinical trials and informed medical judgment remain unwavering cornerstones in ensuring the safety and efficacy of new drugs.
- AI Decision-Making: The inner workings of AI models used in drug discovery can be intricate. Transparency regarding the decision-making processes employed by AI is essential. This allows researchers to comprehend the rationale behind the AI’s recommendations, fostering trust and confidence in the overall process.
- Bridging the Global Healthcare Divide: Unequal access to AI-powered advancements in drug discovery has the potential to exacerbate existing healthcare disparities. Efforts must be directed towards ensuring that the benefits of these innovations reach individuals globally, regardless of their socioeconomic background.
Conclusion
The confluence of artificial intelligence (AI) and drug discovery is revolutionizing healthcare. Driven by the urgent need to address unmet medical needs for chronic diseases, AI offers a faster and more efficient approach to discovering life-saving medications. Pharmaceutical companies are actively integrating AI tools into their pipelines, optimizing various stages of drug development from target identification to clinical trials.
This collaborative effort, which includes CROs, research institutions, and government support, fuels market growth while advancements in AI algorithms and computational power enhance the technology’s effectiveness. This translates to faster development of life-saving medications, reduced healthcare costs, and advancements in personalized medicine, ultimately shaping a healthier future for all.
How Can Idea Usher Help?
Unleash the power of AI to revolutionize your drug discovery efforts. our team of AI specialists merges cutting-edge expertise with deep scientific understanding to expedite your journey.
our approach integrates real-world scientific data and clinical trial results to train our AI models, ensuring practical applicability. We encourage a collaborative spirit, working closely with you throughout the process to tailor AI solutions that perfectly align with your specific drug discovery goals. Don’t wait to revolutionize drug discovery – partner with Idea Usher today and propel your research toward a healthier future.
Partner with Idea Usher today and harness the transformative potential of AI. Together, let’s propel your research towards groundbreaking new treatments.
Hire ex-FANG developers, with combined 50000+ coding hours experience
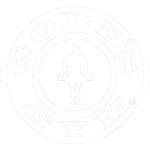
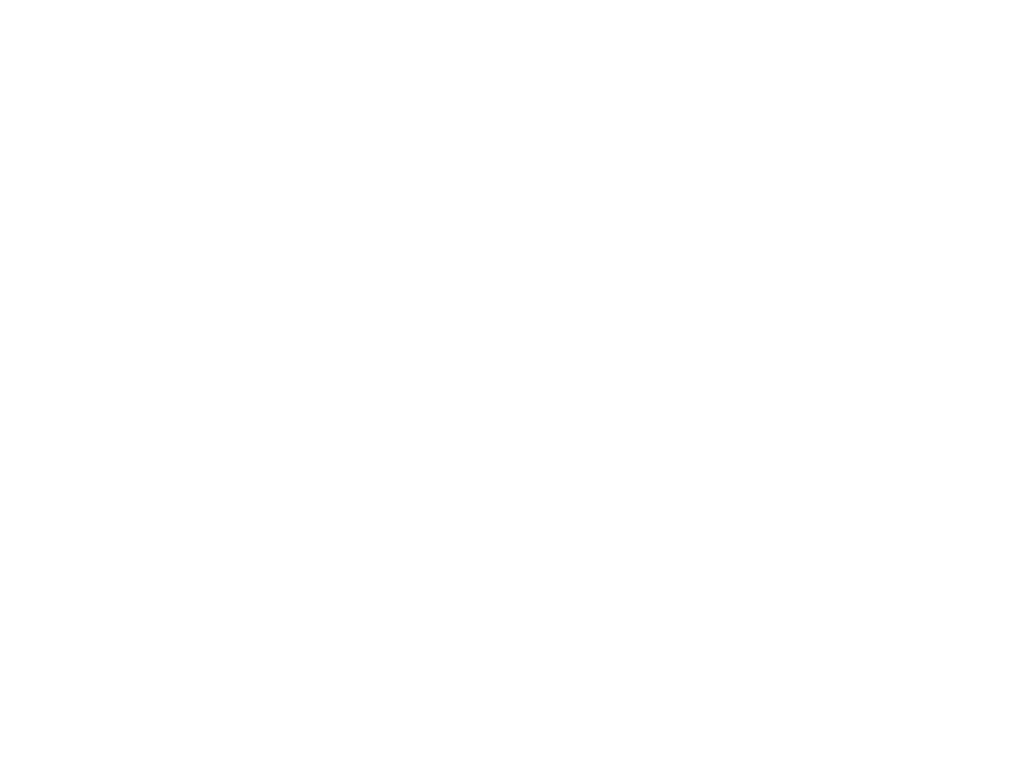
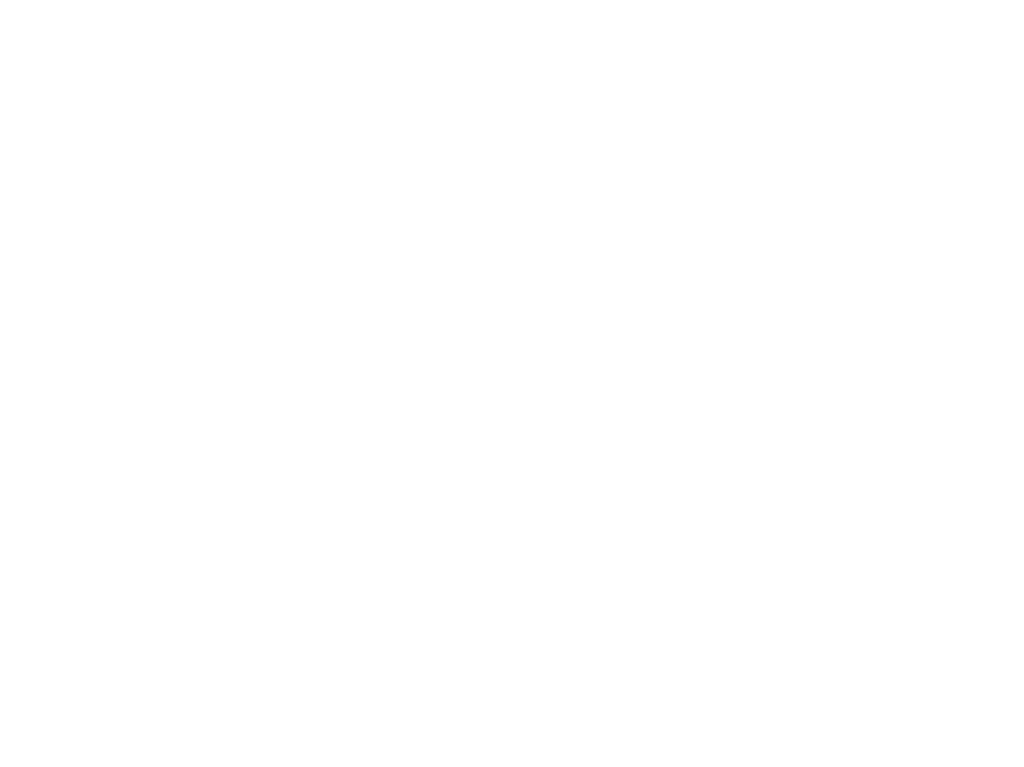
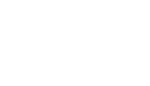
FAQs
What is the role of AI in drug development?
AI sifts through massive databases to find promising starting points for new drugs and also identifies potential drug molecules. AI predicts a drug’s effectiveness and side effects, guiding development before trials. It even helps choose the right people for trials, ensuring efficient research. This multifaceted approach holds immense promise for speeding up drug development and delivering life-saving treatments faster.
What drug was developed using AI?
Insilico Medicine, a Hong Kong-based biotech company, pioneers the field with INS018-055. This marks the world’s first AI-designed small molecule inhibitor drug to be administered to human patients.
Will AI overtake medicine?
AI is emerging as a valuable partner in the medical field, not a replacement for doctors. Imagine a doctor with a powerful AI teammate. This AI assistant accelerates the discovery of new treatments, analyzes complex medical data to aid decision-making, and customizes medicine for individual patients. This collaborative approach has the ability to revolutionize healthcare, bringing effective medications to patients at a much faster pace.
How much does it cost to build an AI application?
Building an AI app’s cost hinges on its complexity. Simpler apps cost $6,000 – $50,000+, while moderately complex projects range from $50,000 to $80,000+. Advanced solutions can cost upwards of $80,000. This variation depends on the model’s intricacy, development approach, team expertise, and data required.